Participant bias can distort research results, affecting data reliability. This article explores its types, causes, and practical strategies to mitigate bias in studies. Learn how to design experiments that minimize bias, enhance validity, and improve data accuracy. Discover expert insights and best practices for conducting unbiased human behavior research.
Table of Contents
- What’s the Bias? The Social Desirability Effect
- How Can We Prevent Social Desirability Bias?
- The Randomized Response Technique
- What’s the Bias? The Halo Effect
- How Can We Prevent This?
- What’s the Bias? Yea- and Nay-saying / Acquiescence
- How Can You Prevent Participant Fatigue?
- Using Biosensors To Overcome Participant Bias
- Free 44-page Experimental Design Guide
- References
When we carry out psychological research, we want to know what people think. We want to get to the truth of their thoughts and feelings, so that we can learn something about the way that humans tick. In an ideal world, all participants would provide honest and clear answers about their innermost thoughts – but we know that this isn’t always the case.
Participants will sometimes second-guess what the researcher is after, or change their answers or behaviors in different ways, depending on the experiment or environment [1]. This is called participant bias, or response bias, and it can have a huge impact on research findings.
Since the dawn of psychological research, self-reporting has been used to yield insights, and it has been known for almost as long [2] that this participant bias can – and often does – produce a meaningful amount of error.
This article is part of our series on bias in research! We have also discussed researcher bias and selection bias.
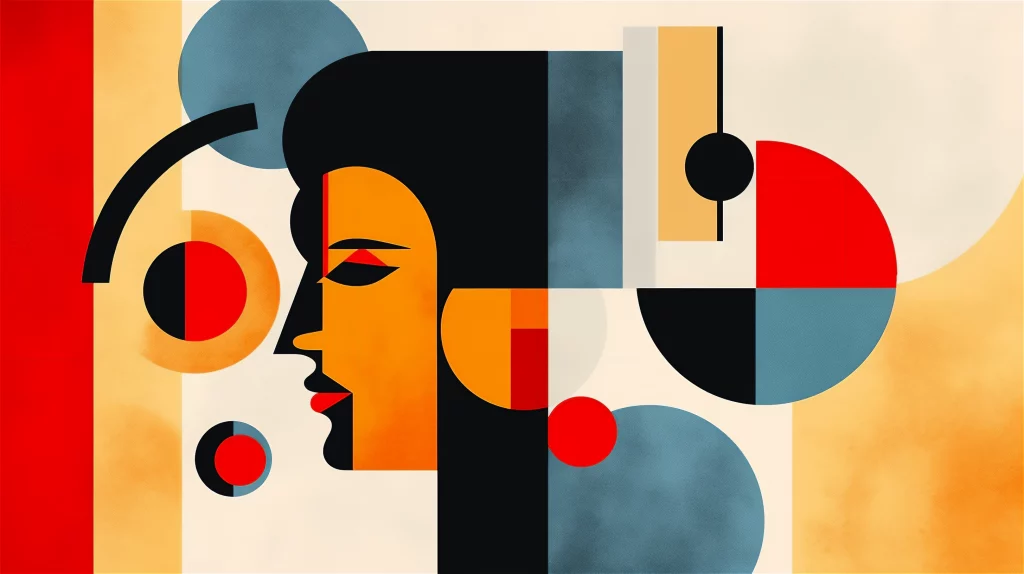
Participant bias has commonly been thought of as the participant reacting purely to what they think the researcher desires [3], but this can also occur for less apparent reasons, as we can see below.
One of the additionally confounding impacts of participant bias is that survey results can often still show internal validity (where conclusions based on the findings appear to be correct). It can therefore be difficult to determine if participant bias is even occurring, and attempts to correct for it are ultimately hampered further.
As with anything that increases error in research, it’s clear that being both aware of participant bias, and controlling for its effects from the start of the experiment, can be crucial for scientific success.
We’ll now go through some of the ways in which participant bias occurs, and what we can do to diminish the effects. Of course, no study will ever be perfect, but with a bit of caution and preparation, we can get pretty close.
What’s the Bias? The Social Desirability Effect
One of the more prevalent factors that shape participant responses is that of social desirability (known as the social desirability bias). Participants often want to present the best versions of themselves, or at least a version that is socially acceptable. It can therefore be difficult for participants to truly open up when it comes to sensitive topics.
Consider a question that relates to sensitive topics such as an individual’s income, their religion, or their benevolence. A very real pressure exists for participants to conform to what they perceive to be socially desirable. They may therefore distort their answers to what they believe is best, rather than give an honest answer.
How Can We Prevent Social Desirability Bias?
There are a number of things that can be done to mitigate the effects of social desirability bias.
By ensuring that the participants know that their data is truly confidential, they will be more likely to reveal the truth, even if they don’t believe it is of great social desirability. Taking this a step further, complete anonymity – in which the experimenter never meets the participant – could provide the individual with a sense of safety that is conducive to revealing particularly sensitive information.
Furthermore, it’s important that the information is presented in a judgement free manner. This is in regards to everything from the advertisement for the study, the formulation of the questions, and the way in which the information is treated afterwards (a researcher who treats sensitive or taboo topics with respect when publishing will give more confidence to prospective participants in the future too).
The Randomized Response Technique
One ingenious method for attempting to control for social desirability bias is called the Randomized Response technique. This involves, as the name suggests, randomizing the responses. In practice, this is done by telling participants to flip a coin, and to say “yes” if the coin lands on tails, and to tell the truth if the coin lands on heads (or whichever side has been determined to be the “truth” side of the coin).
In this way, only the participant knows if they are telling the truth (it’s of course important that the experimenter doesn’t see the results of the coin flips). This provides an extra layer of safety, as even if a participant’s results were revealed or known, it would be impossible to know which of their answers are true or not. This can be particularly helpful if the participant fears legal repercussions for their answers.
This method requires a fairly large sample size [4], and that the number of answers for “no” (or the “truth” side of the coin) should be doubled after data collection. This is because of the assumption that there exists as many participants in the group that should say “no”, but were told to say “yes” regardless.
What’s the Bias? The Halo Effect
When we like someone, we often overlook their misgivings or faults, tending to see the best in them. This applies not only to people, but to our perceived experiences with many things in life. If we want to measure an individual’s thoughts about something, we can anticipate that if they have a positive opinion about it, they will also have a positive opinion about the things that are associated with it.
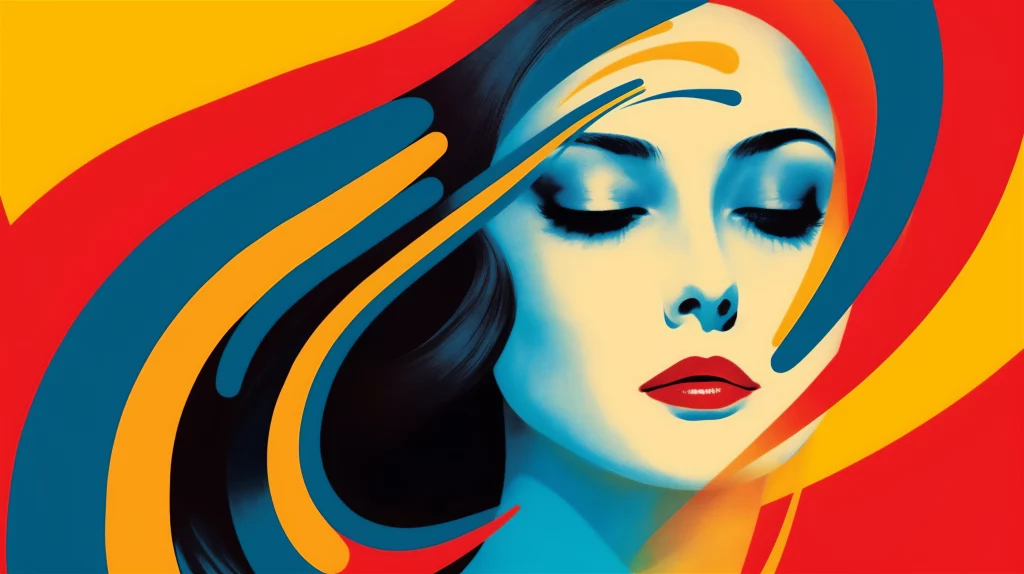
This bias also works in the opposite direction – the reverse halo effect (or “the devil effect”) means that an individual can react badly to something if it’s already associated with a negatively perceived person, or thing. This can occur even if an individual would have a neutral, or even positive, opinion about the subject in question if it was associated with something or someone else.
Both of these biases are examples of cognitive carryover effects [5], and they can have a huge effect on on how we perceive the world.
How Can We Prevent This?
This bias can be difficult to control for, as people of course have a range of preconceived opinions about almost everything they encounter in life. One of the ways to help deal with this bias is to avoid shaping participants’ ideas or experiences before they are faced with the experimental material.
Even stating seemingly innocuous details might prime an individual to form theories or thoughts that could bias their answers or behavior. It is therefore important to provide the participant with only the information that is needed for the task at hand, and to avoid extraneous detail.
Furthermore, having a large sample size is rarely a bad thing for an experiment, and in this case is particularly useful. If we have a large number of participants then we increase the likelihood of obtaining our data from a mixed population that reflects the population at large. If this is balanced for negative and positive opinions (or rather, is balanced proportional to the natural population) then we can still draw conclusions from this group.
What’s the Bias? Yea- and Nay-saying / Acquiescence
This bias can emerge in self-reporting measures (such as questionnaires that are completed by the participant), and relates to participants showing an increased proclivity to respond with “yes” to “yes” or “no” questions, or to simply respond with all “yes” or “no” answers throughout.
There are several reasons why this effect can emerge, from the participant aiming to disrupt the research, an attempt to please the experimenter [6] through acquiescence, and as a result of participant fatigue.
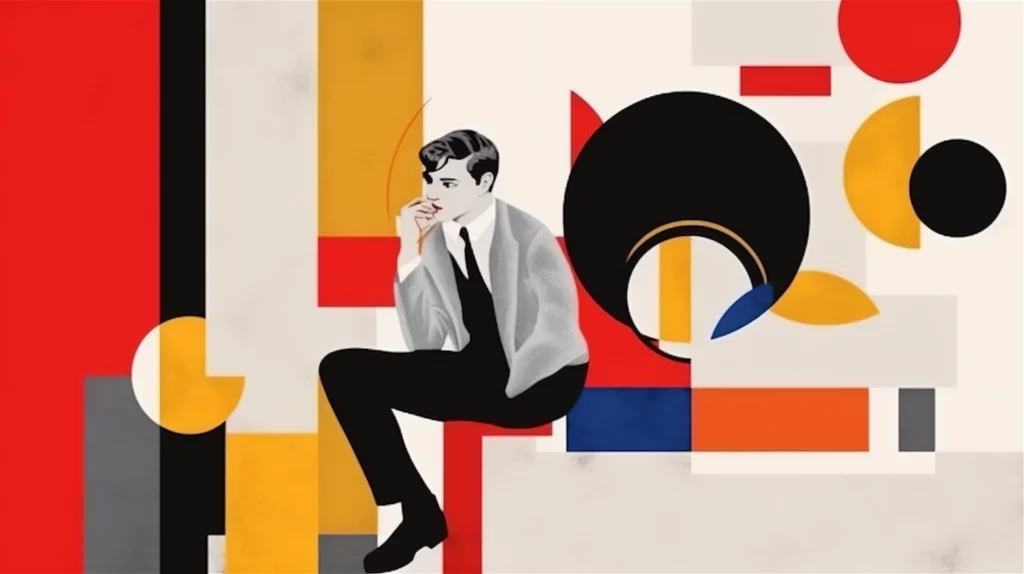
How Can You Prevent Participant Fatigue?
There are fortunately several ways in which this bias can be prevented and / or corrected for. One of the simplest methods is to ensure that the questions are balanced in their phrasing.
Ensuring that there aren’t any leading questions is important for all surveys, questionnaires, or interviews, and it is particularly relevant in this case.
This also feeds back into the social desirability bias – try to ensure that the questions aren’t phrased in such a way as to make the participant think that they have a social responsibility to answer in a certain way. This approach is much more likely to yield truthful answers.
Furthermore, balancing the questions to reveal contradictory information can help spot erroneous patterns of answers [7]. In practice this means providing oppositely phrased questions throughout. If a participant is therefore asked “do you like psychology?” then there should also be a question that asks “do you dislike psychology?”. If the participant has completed “yes” for both questions then there may be a problem with their answers.
Additionally, the number of questions shouldn’t be more than are needed – too many questions increases the chance of inducing participant fatigue, leading to answers that are given without considered thought.
Using Biosensors To Overcome Participant Bias
In addition to the steps above, there are several ways in which biosensors can be easily used to reduce the effects of participant bias in research.
It’s simple to add another counterbalance to the misleading effects of participant bias with iMotions. You can readily utilize biosensors to guard against distorting effects, and also run the experiment itself inside the software. This provides an all-in-one platform to both carry out research, and to make sure that the research is as free from bias as can be.
One example of this is through calculations of frontal asymmetry from EEG measurements. If there is increased alpha wave activity in the left hemisphere of the brain, relative to the right, the participant is likely engaged by the stimulus (conversely, increased right hemisphere alpha wave activity is indicative of feelings of avoidance). This provides a metric of enthusiasm for examining a participant’s feelings about the matter at hand.
Furthermore, eye tracking can be used to measure attention, revealing biases in how much a participant is interested in the stimuli (there is also encouraging research that relates pupil size to deception [8], providing another metric for uncovering the participant’s true feelings). Combined with facial expression analysis, we can start to reveal the emotional valence that is felt by the participant.
The strength of psychological research is in knowing as much as
possible about the participants. By combining multiple sensors in iMotions, the data to inform decisions about bias, and the conclusion, is easily obtained, and easily understood. This streamlines the steps to robust results, and adds more assurances to the validity of research.
The impact of biases in research can be both difficult to prevent, and tricky to correct for even if the effects are known. Ensuring and maintaining a high level of reliability is however a central part of research. By using the information above, complemented with biosensors, the impacts of participant bias can be reduced, guaranteeing that all you’re left with – is the truth.
This article is part of our series on bias in research! We have also discussed researcher bias, which you can read by clicking here, and selection bias, which you can read by clicking here.
If you’d like to get more information about how to design the perfect study, then click below to download our free pocket guide for experimental design, and continue your path to experimental success!
Free 44-page Experimental Design Guide
For Beginners and Intermediates
- Introduction to experimental methods
- Respondent management with groups and populations
- How to set up stimulus selection and arrangement
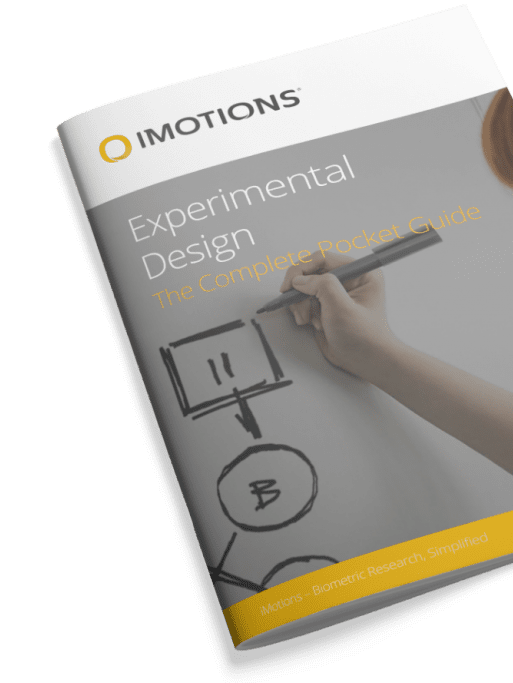
References
[1] McCambridge, J., de Bruin, M., & Witton, J. (2012). The Effects of Demand Characteristics on Research Participant Behaviours in Non-Laboratory Settings: A Systematic Review. Plos ONE, 7(6), e39116. doi: 10.1371/journal.pone.0039116
[2] Gove, W., & Geerken, M. (1977). Response Bias in Surveys of Mental Health: An Empirical Investigation. American Journal Of Sociology, 82(6), 1289-1317. doi: 10.1086/226466
[3] Greenberg, B., Abul-Ela, A., Simmons, W., & Horvitz, D. (1969). The Unrelated Question Randomized Response Model: Theoretical Framework. Journal Of The American Statistical Association, 64(326), 520. doi: 10.2307/2283636
[4] Warner, S. (1965). Randomized Response: A Survey Technique for Eliminating Evasive Answer Bias. Journal Of The American Statistical Association, 60(309), 63. doi: 10.2307/2283137
[5] Tourangeau, R., Rasinski, K., Bradburn, N., & D’Andrade, R. (1989). Carryover Effects in Attitude Surveys. Public Opinion Quarterly, 53(4), 495. doi: 10.1086/269169
[6] Knowles, E., & Nathan, K. (1997). Acquiescent Responding in Self-Reports: Cognitive Style or Social Concern?. Journal Of Research In Personality, 31(2), 293-301. doi: 10.1006/jrpe.1997.2180
[7] Cronbach, L. (1942). Studies of acquiescence as a factor in the true-false test. Journal Of Educational Psychology, 33(6), 401-415. doi: 10.1037/h0054677
[8] Dionisio, D., Granholm, E., Hillix, W., & Perrine, W. (2001). Differentiation of deception using pupillary responses as an index of cognitive processing. Psychophysiology, 38(2), 205-211. doi: 10.1111/1469-8986.3820205