Good research begins well before the first experiment starts.
During World War II, a statistician by the name of Abraham Wald was given a rather unexpected job, given his background: of improving the survival rate of US aircraft. Wald was a smart man and looked over the prior analyses that had been done. The previous investigators had seen the damage and destruction dealt to the aircrafts, and advised more armor being added to the most damaged areas, to increase their protection. Specific parts were shot and torn up, so new armor was added.
Yet the survivability rate didn’t increase. In fact, it decreased, as the new armor added weight and reduced the agility of the planes, and they still arrived back with damage in the same areas. Wald observed all of this and advised that the airforce start adding armor only to the untouched areas – the parts without a trace of damage. He reasoned that the only data about survivability was coming from the surviving planes themselves; the ones that came back with damage showed exactly where the non-lethal blows could be dealt.
With the advice taken onboard, the survivability increased and the rest is, well, history. While this makes for a great example of lateral thinking, it also tells us something critical about data collection – that of selection bias.
Selection bias is an experimental error that occurs when the participant pool, or the subsequent data, is not representative of the target population.
There are several types of selection bias, and most can be prevented before the results are delivered. Although there might not always be an entire airforce on the line when it comes to getting it right, it’s still essential for good research.
Let’s go through some examples, and explore what can be done to stop this bias occurring before the first data point is even collected.
Sampling Bias
There are several aspects of sampling bias, all of which ultimately mean that the population being studied does not provide the data that we require to make conclusions.
A common example of this happening in practice is through self-selection. Specific groups of people may be drawn to taking part in a particular study because of self-selecting characteristics. It is known that individuals who are inclined to sensation-seeking, or thrill-seeking are more likely to take part in certain studies, which could skew data from a study if it is examining those personality traits (and possibly within other studies too).
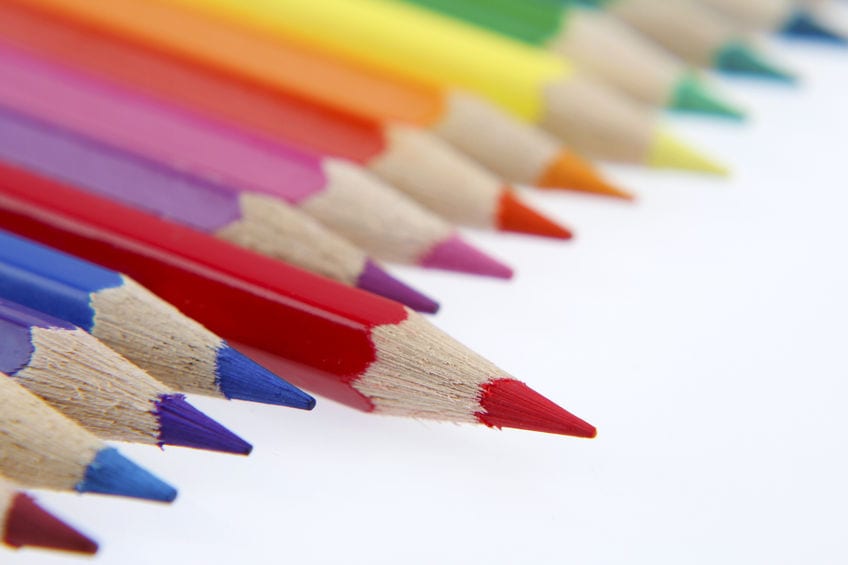
The best way around this bias is to draw from a sample that is not self-selecting. This may not always be possible of course, due to experimental constraints (particularly for studies requiring volunteers), but particular effort should be made to avoid the potential for this bias when examining different personality types. The effects of this bias are unlikely to be so detrimental if the experiment is concerned with something more constant, such as psychophysiological measurements.
Pre-screening
Another pitfall that experimenter’s can fall into is to pre-screen participants. There can be good reasons to do so (for example, to ensure correct control groups), but this can also have the effect of distorting the sample population. As a consequence this could result in selecting participants that share a common characteristic that will affect the results.
This is similar to self-selection in outcome, but is lead by the researcher (and usually with good intentions). To avoid this, a double-blind experiment may be necessary where participant screening has to be performed, meaning that the choices are made by an individual who is independent of the research goals (which also avoids experimenter bias).
Participant attrition
The sample can also be affected by the experimental setup while it’s in action. If participants drop out of the study in a biased way – if there is a non-random reason why this is occurring – then the remaining participants are unlikely to be representative of the original sample pool (never mind the population at large).
This drop out rate is known as participant attrition, and is most commonly seen within investigations where there is an ongoing intervention with several measurements. For example, a medical trial may see numerous participants exit the study if the medicine doesn’t appear to be working (or is making them ill). In this way, only the remaining (or surviving, in Wald’s case above) participants will be investigated at the end of the experiment.
It’s therefore important that participants who drop out of the study are followed up after doing so, in order to determine whether their attrition is due to a common factor with other participants, or for reasons external to the experiment.
Let’s talk!
We love to learn about the research projects that you are working on and helping you out with achieving your goals. Get a free demo of our software, get advice on hardware purchases or learn about our enablement services that teaches you the skills needed for conducting biometrics research.
Undercover / classified
It should come as no surprise that having too few participants will limit the strength of the conclusions that can be made (if they can be made at all), yet many studies do suffer from undercoverage of sample groups.
It is therefore critical that enough participants are available and selected beforehand. This can be calculated in advance, allowing you to plan the study accordingly. If too many participants also drop out due to attrition, then the study may need to be repeated.
A further point to note is that even if you have enough participants, you need to make sure that they’re classified correctly, and put into the right experimental group. Carrying out a study on bilinguals and monolinguals would of course be hampered if it came to light that some participants spoke one more (or less) languages than their grouping would suggest.
This is particularly pertinent in studies examining different mental disorders, in which the grouping definition could be unclear. For example, studies of anxiety may need to differentiate between participants who have been diagnosed with a generalized anxiety disorder or those who suffer from panic attacks, and even if participants exhibit subclinical/prodromal symptoms.
Ensuring that the sample is well-defined, and well-characterized, before beginning the study will, therefore, ensure that the findings are relevant to the group of interest.
Picking cherries, dredging data
While most of the selection biases occur before the data has been collected, there are several steps that occur post-hoc which are open to erroneous distortion. These steps instead to relate to how the data, rather than the sample, is selected.
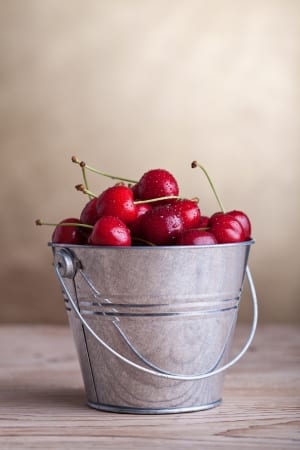
Cherry-picking is undoubtedly a good way to prepare a pie, but is also the phrase given to the act of only selecting data that conforms with what the experimenter is expecting, or hoping, to see.
This can occur due to malpractice, or perhaps wishful thinking on behalf of the investigator. Ultimately though, this leads to bad science either way. The investigator must remain open-minded to the contents of the data, and question how they are interpreting things. It may also help if several people (ideally independent) check the data of the study.
Similar to the above, data-dredging (also known as fishing for data, or p-hacking) is the practice of only considering the data that is significant after the experiment, and inventing post-hoc conclusions for why that emerged. This usually arises when a large number of variables investigated, and spurious results can appear significant.
By taking only significant variables from a dataset, this is essentially the same as running the same experiment multiple times, and publishing the one occurrence in which significant differences were found.
Experimental reproducibility is a particularly important tenet of science that should be maintained when there is a possibility of data-dredging. With enough replications, the research will be shown to be true or false.
Trick splits
Finally, in a similar way to misclassifying the participants before the experiment, their data can be misclassified after the fact. Incorrect partitioning of data is a way of dividing, or not using, certain parts of the data based on false assumptions.
This veers quite strongly into fraudulent data manipulation, but it can also occur for reasons due to technical errors, rather than through intentional malpractice.
Back it up
In addition to the steps above, there are a few ways in which using iMotions for data collection implicitly guards against some trappings of selection bias, particularly after data collection has taken place.
Using multiple data sources, as with multiple biosensors, can provide another way in which to check your data, by observing if the recordings are in agreement with each other. For example, using both GSR and ECG can help you confirm the levels of physiological arousal, while facial expression analysis can complement survey testing (if someone appears unhappy while claiming the opposite in the survey, then this could be reason for caution with their data). These measures can ultimately give you more confidence in the data that is collected.
Furthermore, being able to view the data recorded in real time in a graphical and intuitive format decreases the chance of being misled by the numbers alone. A spreadsheet of endless numbers can offer almost as many opportunities to find confusion, yet having the real data displayed in an easily understood format provides clarity for investigation.
How to fix everything by not keeping secrets
The use of iMotions largely helps protect against the data selection bias, yet the selection of participants is something that primarily relies on good experimental design.
While the attempts to fix the emergence of sampling biases may not always be completely feasible, there is one central thing that can be done to stem the bias – be clear with the results. When stating findings, it’s important to be transparent with whom the results are applicable to.
In our article about participant bias we talked about how the internal validity of the experiment could be problematic, as the results would appear to be correct, yet would actually be biased. For selection bias however, we find that external validity is a more likely culprit – the results appear to be applicable to the population at large, yet are actually biased and invalid for such generalizations.
For experimental integrity, it’s therefore important that the participant information, the data analysis, and the resulting conclusions are made as open and clear as can be.
If you want to know more about biases in research, or would like to learn about how iMotions can help your research, then feel free to contact us.
I hope you’ve enjoyed reading about how to avoid selection bias in research. If you want more tips and tricks for great research, then check out our free pocket guide for experimental design below.
Free 44-page Experimental Design Guide
For Beginners and Intermediates
- Introduction to experimental methods
- Respondent management with groups and populations
- How to set up stimulus selection and arrangement
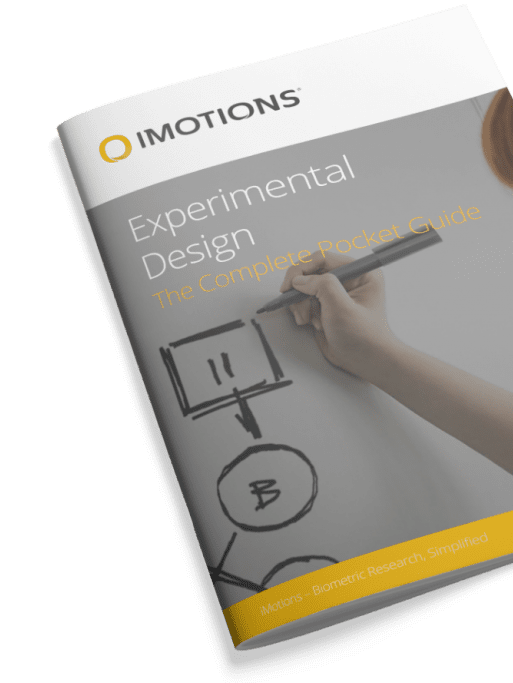