Science is all about getting to the truth. The truth of humans however, is perhaps even more elusive than in any other realm of science. It should come as no great surprise then that researching humans is a tricky feat, and difficult to get right.
Without a properly and rigorously designed experimental setup, errors can emerge in multiple ways. Not least among these are biases in research, that can have a broad impact, and without preparation, are difficult to stop. Such biasing factors can be produced entirely without intention, but can ultimately damage the reliability (and credibility) of research if it’s not properly controlled.
There are several aspects and pitfalls within research that can produce these erroneous biases, leading both the participants, or researchers astray, and dealing with data that is not truly reflective of the tested thoughts and behavior.
Biases within research are widespread, but can often be overcome with good methodological controls, and by picking the most suitable equipment to yield the right answers. Below we’ll go through some of the most common biases that plague research, and provide routes to avoid them. With these in mind, you can guide your research to ever greater discoveries.
Table of Contents
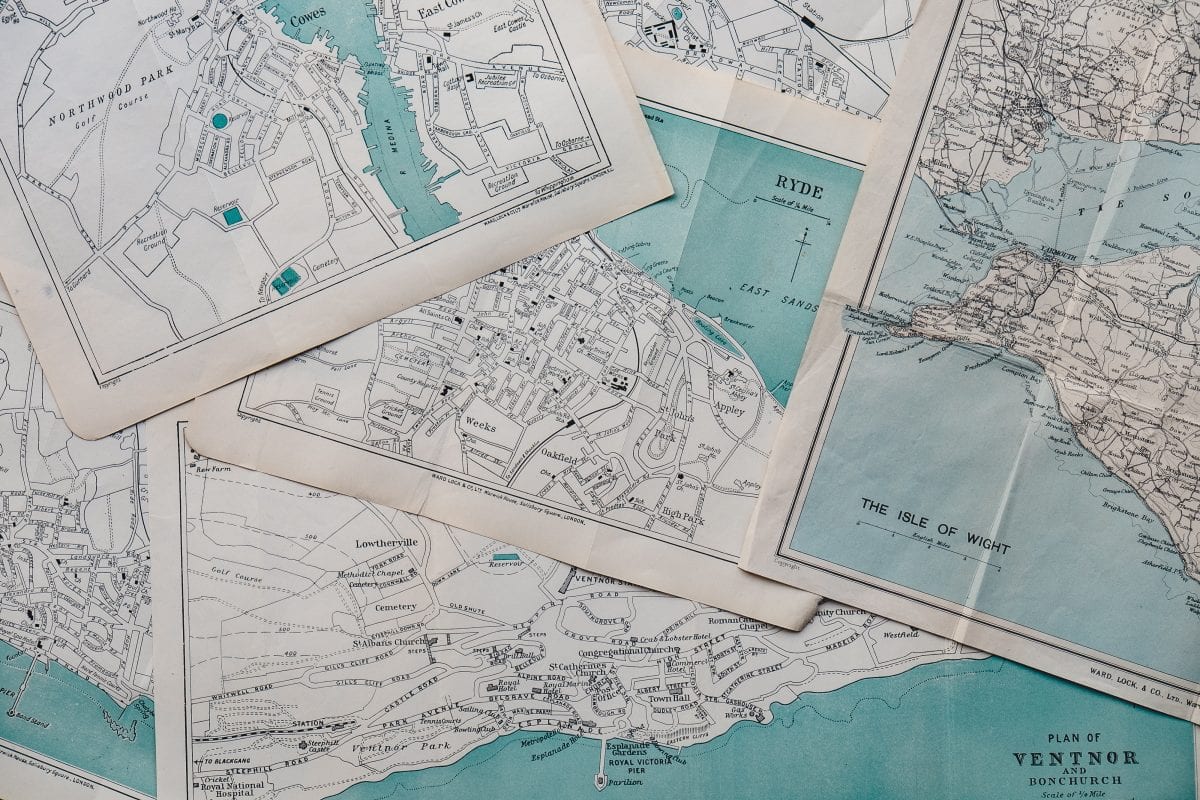
Three Biases that can impact research
1. Participant Bias
One of the central biases that can hamper and negatively impact research is that of participant bias. This has often been described as the participant reacting purely to what they think the researcher desires, but this can also occur for less obvious reasons.
The social-desirability bias is an example of this. Participants may have preconceived notions about what is an acceptable answer, or behavior, so will cater their responses to match this – either consciously or nonconsciously.
This reaction is particularly likely with experiments that cover sensitive topics (such as with personal income, or religion for example) and will ultimately distort the findings into something that isn’t true.
Participants may also acquiesce to everything, or answer negatively to the questions (also known as “yea-saying” or “nay-saying”). This can happen due to fatigue, boredom, or even intentional attempts to disrupt the research.
So those are some of the problems that can occur with participant bias, but what about solutions? Taking precautions with experimental design can help a great deal, and having the right tools can help further still.
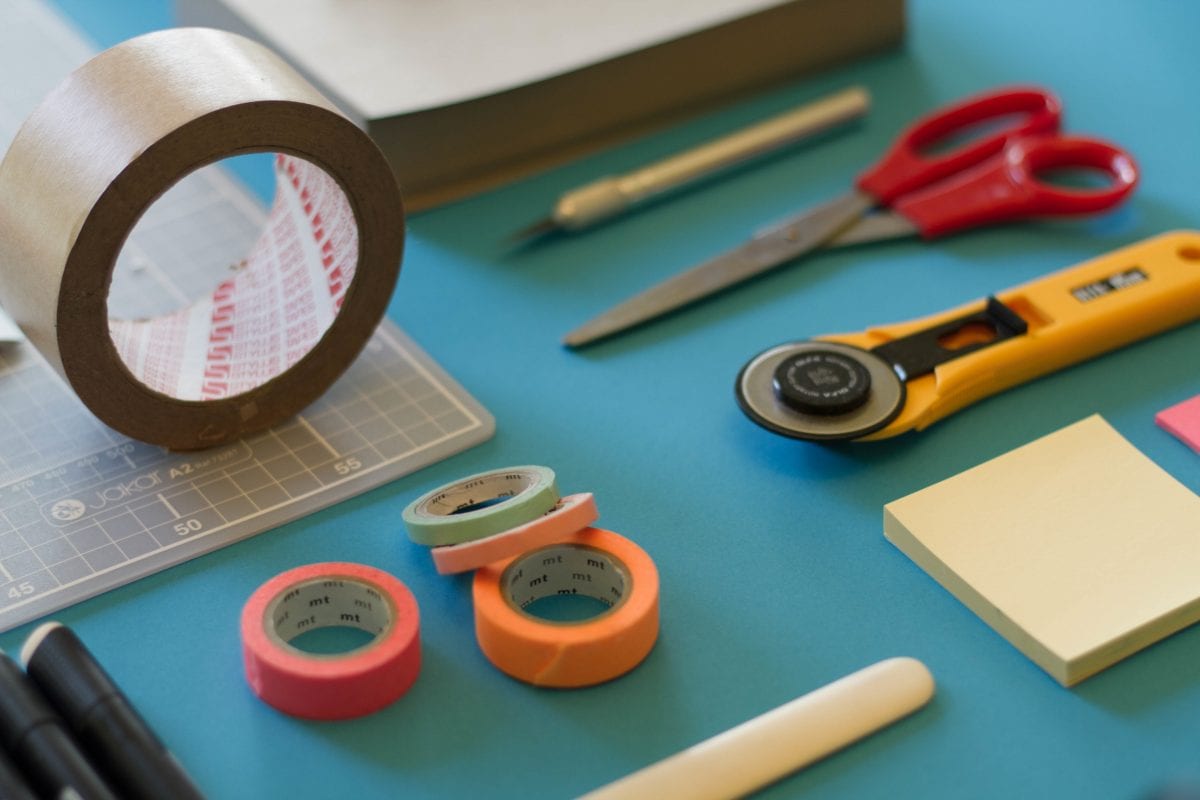
In the case of social-desirability biases, it is important to inform the participant about their anonymity (and to ensure that too). For “yea- / nay-saying”, it is important to properly motivate the participant – either with remuneration, or enough breaks to ensure they don’t become fatigued. Checking for outliers in the data can also help as a last check.
Check out: What is Participant Bias? (And How to Defeat it)
Further to this, psychophysiological measurements can help you see through the potentially misleading answers or behaviors, and provide a clearer picture of what’s really going on. Biosensors enable you to measure a participant’s response, without it being consciously filtered.
They can also provide data without any real effort from the participants. For example, measuring the attention of a participant is easily completed with eye tracking, and doesn’t require extra energy from them. This makes it much easier to keep the participant engaged in the study.
It’s also possible to record a participant’s emotional state – their valence – through automatic facial expression analysis, and combine this with recordings of their physiological arousal (such as through galvanic skin response recordings), while they complete an experiment. The combination of these methods provides a complete interrogation of a participant’s mental state, without adding any mental strain.
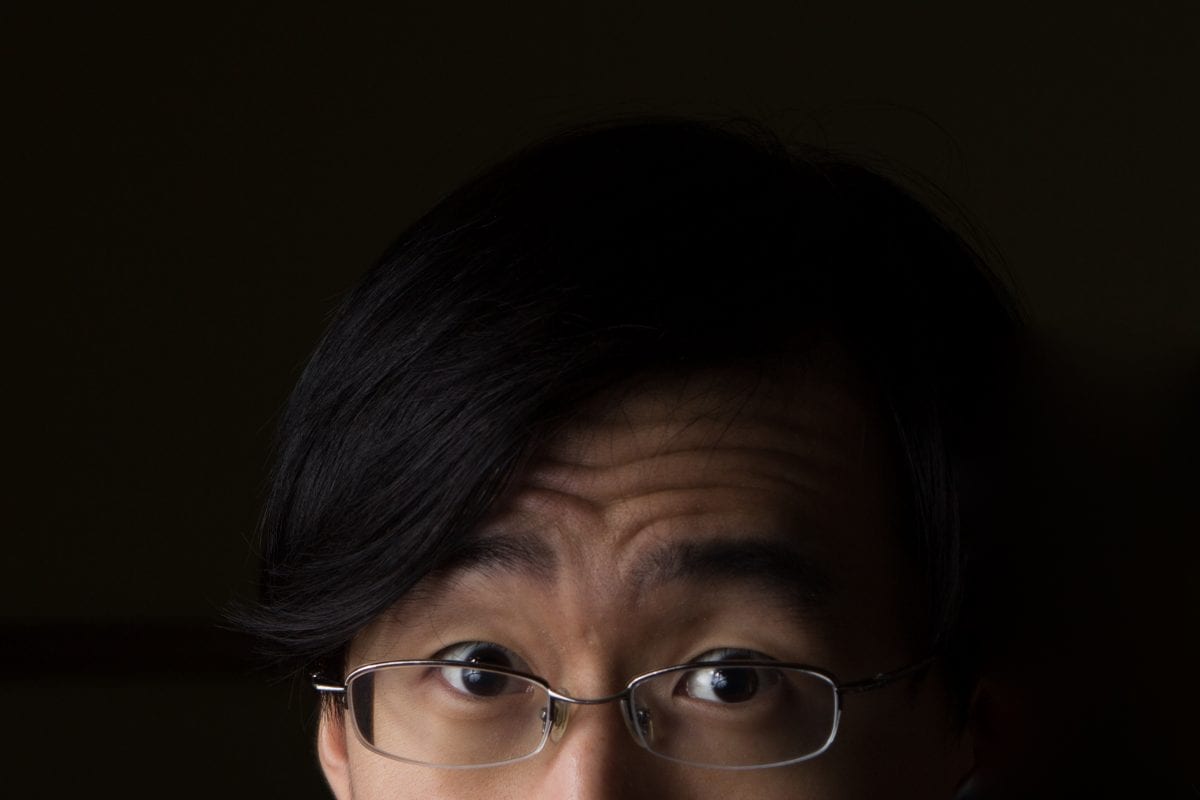
2. Selection Bias
Before the participants complete the experiment, they must first be selected, and this is where selection bias comes in. This can be defined as an experimental error that occurs when the participant pool, or the subsequent data, is not representative of the target population.
This can occur for several reasons, some of which are more avoidable than others. For example, the participants themselves may be self-selecting – particularly when the study is on a volunteer basis – and certain personality types may be more prevalent in that population.
Not having enough participants, or selecting the resulting data in incorrect ways are also examples of methodological aspects that ultimately lead to the incorrect participant pool being examined.
Learn more: What is Selection Bias? (And How to Defeat it)
These biasing factors can be corrected for in multiple ways. Preventing the bias of a self-selecting participant group can be dealt with by having multiple channels or routes open for participants to access the study through. Ideally they will be drawn from a mixed sample group, of self-selecting, or selected participants (for example, with university students completing the study for course credits, and volunteers).
Beyond this, having a large participant pool pretty much always helps too (although this might not always be possible), while being transparent about the data sources will also aid the credibility of a study.
Psychophysiological measurements can also aid the reliability of the findings from participants, as they easily are combined as multiple recordings, in which cross-validation of the data sources can occur. Combining a wide array of metrics means that outliers should be much easier to spot.
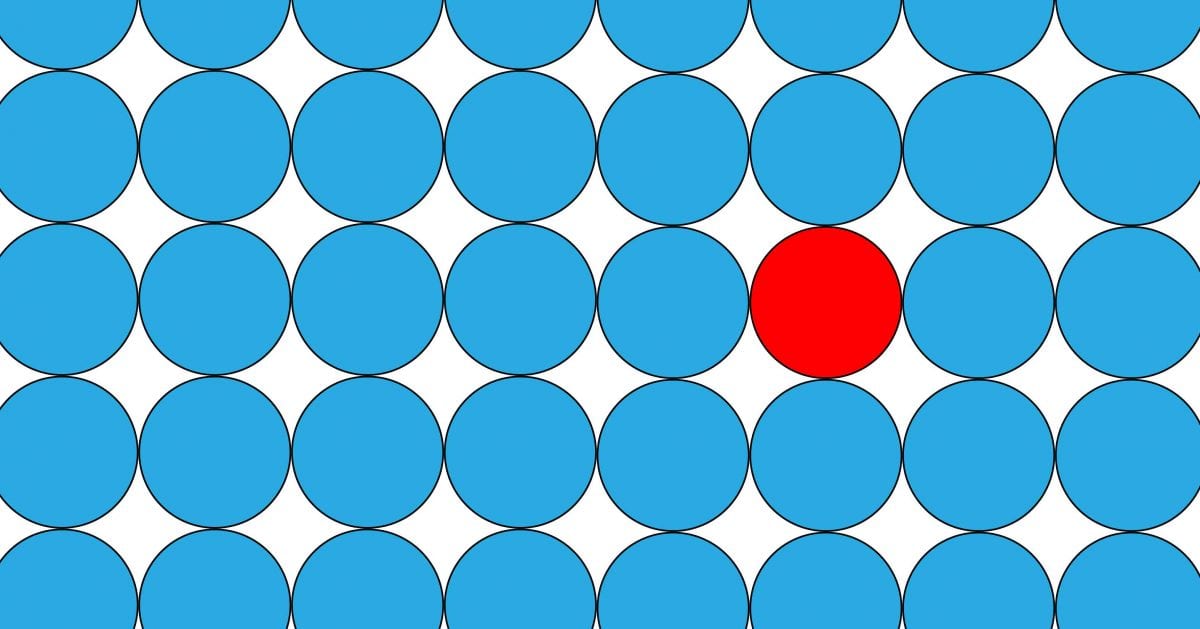
3. Researcher Bias
There is also the often overlooked, and unfortunately, too frequent effects of researcher bias, in which the scientists themselves mislead the research they carry out, often unintentionally but sometimes intentionally.
The researchers may be implicitly biased in favor of a certain result, and troublesome data collection can lead in that direction too, even if false. They may also affect the participants simply by being present – overlooking others can have quite drastic effects (known as The Hawthorne Effect), and change behaviors in unrepresentative ways.
Check out: What is Researcher Bias? (And How to Defeat it)
Getting around this could require completing the research as a double-blind study – in which the participants, and the people carrying out the data collection, don’t know which experimental group is which. This reduces a large degree of bias that could otherwise occur, and despite it adding a large amount of reliability to an experimental setting, it could be too laborious or costly to carry out.
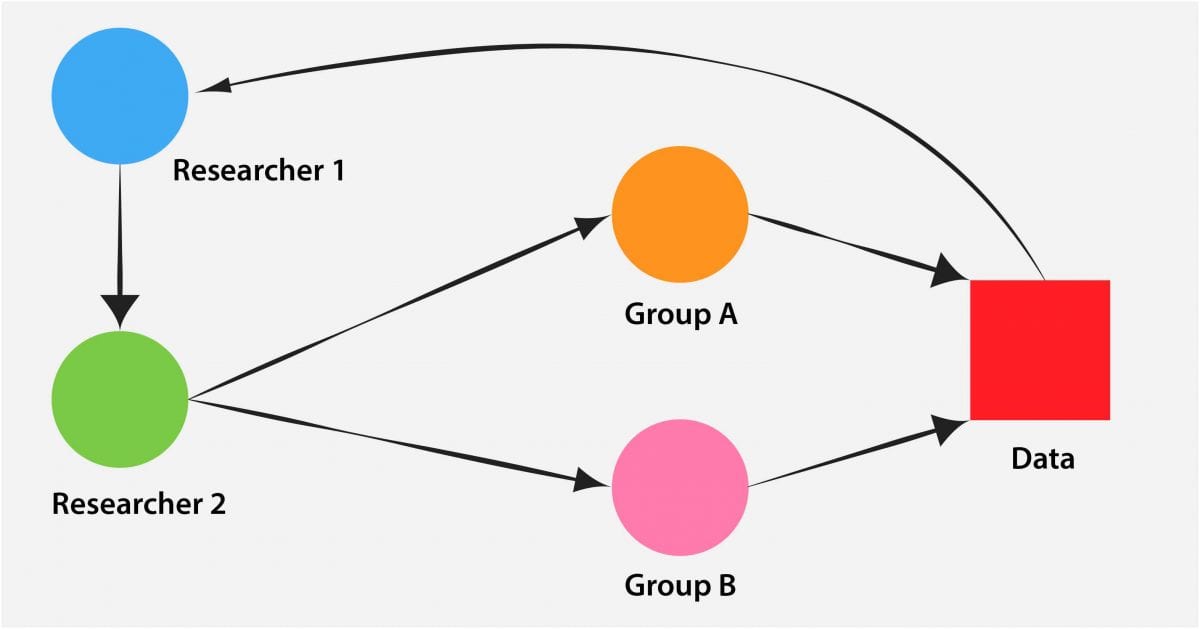
Using predefined platforms to create an experimental plan, and to enforce the conditions within it, ensures a level of consistency and reliability that is otherwise difficult to construct. By implementing (and recording from) the different experimental conditions with a standardized approach, everything can be made consistent, which reduces the chance of any potentially confounding interference occurring.
Using software such as iMotions in this way also helps researchers spend less time on having to direct participants through the study. This enables more time to be spent on getting the methodology right, interpreting the data, and getting the results out.
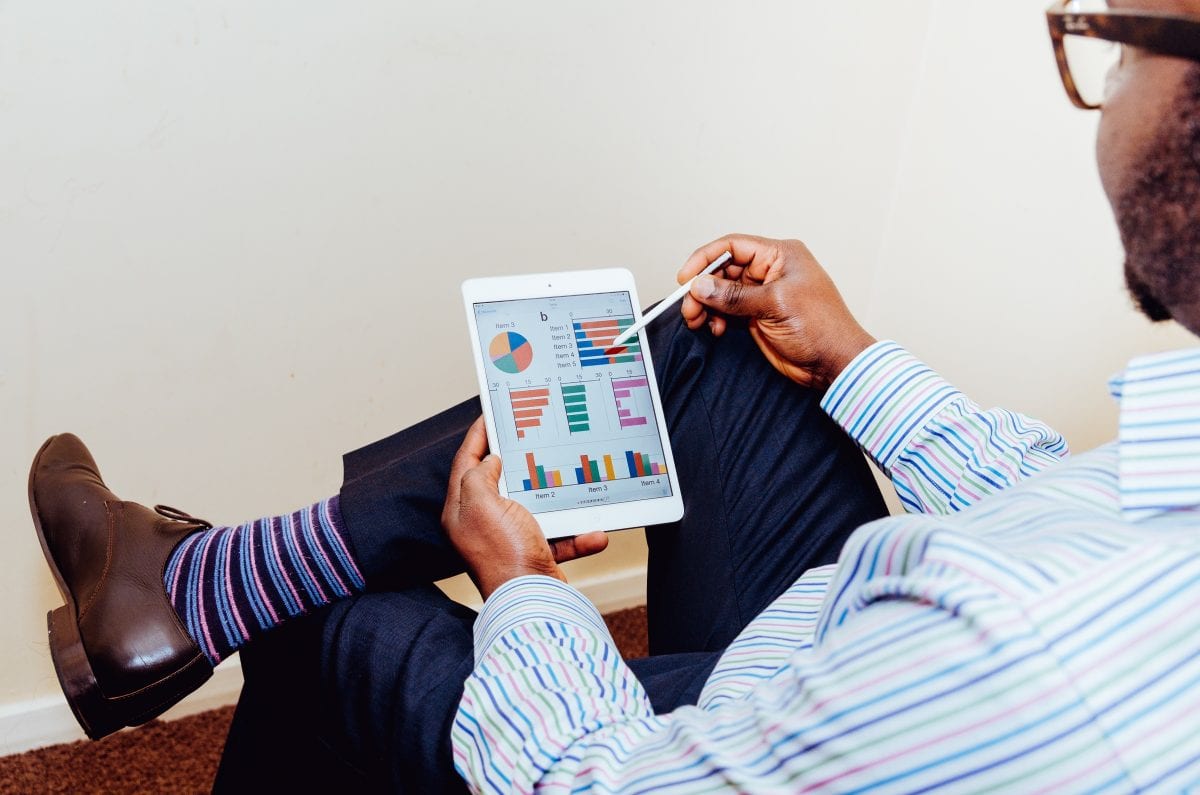
Conclusion
Psychophysiological measurements ultimately allow researchers to peer further into participants’ minds, and their underlying physiological states, which gives access to unfiltered responses and feelings. The recordings from such biosensors can paint a much more honest picture of what someone is thinking, and why they are behaving in a certain way.
Using biosensors in combination allows both cross-validation and a greater depth to findings, increasing the validity of the findings, and therefore the strength of the experiment. This is both easier and less time-consuming, in iMotions.
With this in mind, it’s simpler to both add more data sources to a study, and to use time in a more effective way, meaning that getting to unbiased results – and incredible findings – is easier than ever.
Check out: The Study of Human Behavior: Measuring, analyzing and understanding [Cheat Sheets]
Bias is all too prevalent within research, and I hope this article helps guide you to more objective, reliable, and reproducible results. If you’d like to learn more about bias, then have a look at our past articles that cover participant bias, selection bias, and researcher bias in more detail. And if you’re looking for even more advice and top tips for research, have a read through our comprehensive guide to experimental design. It’s free and amazing, a perfect combination.
Free 44-page Experimental Design Guide
For Beginners and Intermediates
- Introduction to experimental methods
- Respondent management with groups and populations
- How to set up stimulus selection and arrangement
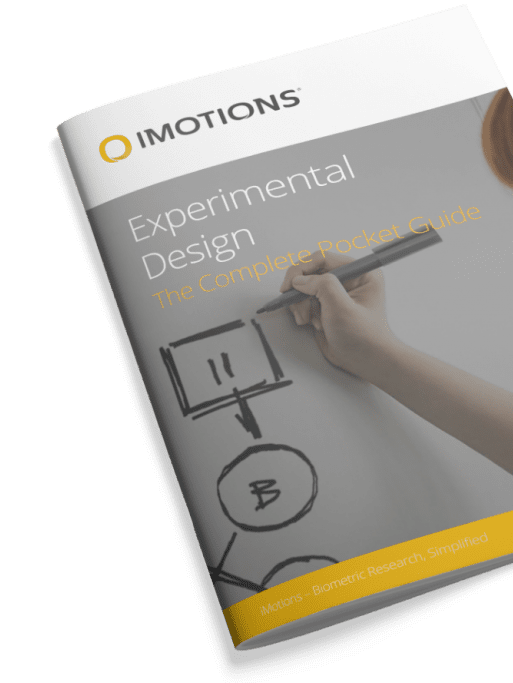