Emotions are the essence of what makes us human. They impact our daily routines, our social interactions, our attention, perception, and memory.
One of the strongest indicators for emotions is our face. As we laugh or cry we’re putting our emotions on display, allowing others to glimpse into our minds as they “read” our face based on changes in key face features such as eyes, brows, lids, nostrils, and lips.
Computer-based facial expression recognition mimics our human coding skills quite impressively as it captures raw, unfiltered emotional responses towards any type of emotionally engaging content. But how exactly does it work?
We have the answers. This definitive guide to facial expression analysis is all you need to get the knack of emotion recognition and research into the quality of emotional behavior. Now is the right time to get started.
N.B. this post is an excerpt from our Facial Expression Analysis Pocket Guide. You can download your free copy below and get even more insights into the world of Facial Expression Analysis.
Free 42-page Facial Expression Analysis Guide
For Beginners and Intermediates
- Get a thorough understanding of all aspects
- Valuable facial expression analysis insights
- Learn how to take your research to the next level
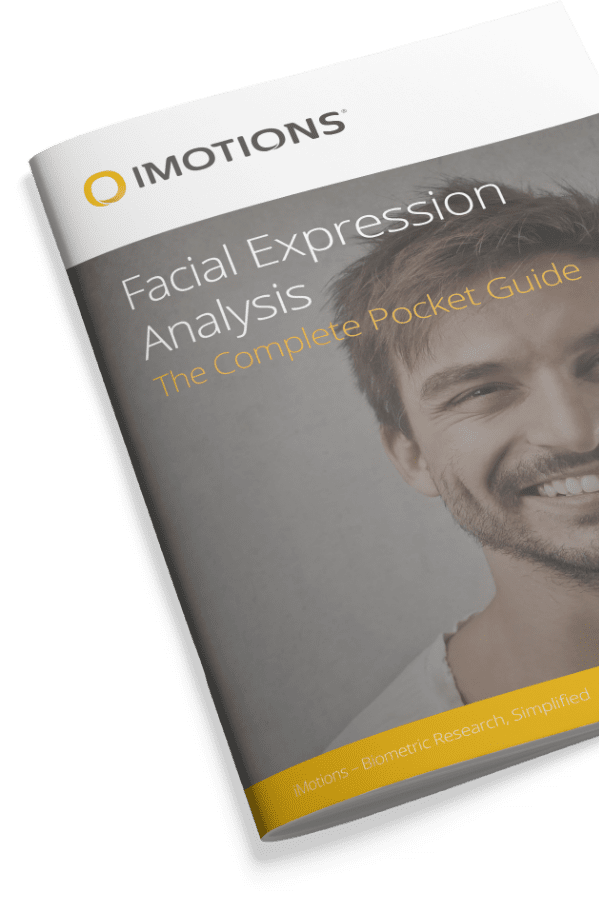
Table of Contents
- Free 42-page Facial Expression Analysis Guide
- What are facial expressions?
- Facial expressions and emotions
- Facial expression analysis techniques
- Facial expression analysis Technology
- Equipment
- Facial Expression Analysis (FEA): Application fields
- How to do it right with iMotions
- Free 42-page Facial Expression Analysis Guide
What are facial expressions?
Our face is an intricate, highly differentiated part of our body – in fact, it is one of the most complex signal systems available to us. It includes over 40 structurally and functionally autonomous muscles, each of which can be triggered independently of each other.
The facial muscular system is the only place in our body where muscles are either attached to a bone and facial tissue (other muscles in the human body connect to two bones), or to facial tissue only such as the muscle surrounding the eyes or lips.
Obviously, facial muscle activity is highly specialized for expression – it allows us to share social information with others and communicate both verbally and non verbally.
Did you know?
Almost all facial muscles are triggered by one single nerve – the facial nerve. There is one exception, though: The upper eyelid is innervated by the oculomotor nerve, which is responsible for a great part of eye movements, pupil contractions, and raising the eyelid.
The facial nerve controls the majority of facial muscles
All muscles in our body are innervated by nerves, which route all the way into the spinal cord and brain. The nerve connection is bidirectional, which means that the nerve is triggering muscle contractions based on brain signals (brain-to-muscle), while it at the same time communicates information on the current muscle state back to the brain (muscle-to-brain).
What are facial expressions?
Facial expressions are movements of the numerous muscles supplied by the facial nerve that are attached to and move the facial skin.
Almost all facial muscles are innervated by a single nerve, therefore simply referred to as facial nerve.
In slightly more medical terms, the facial nerve is also known under “VII. cranial nerve”.
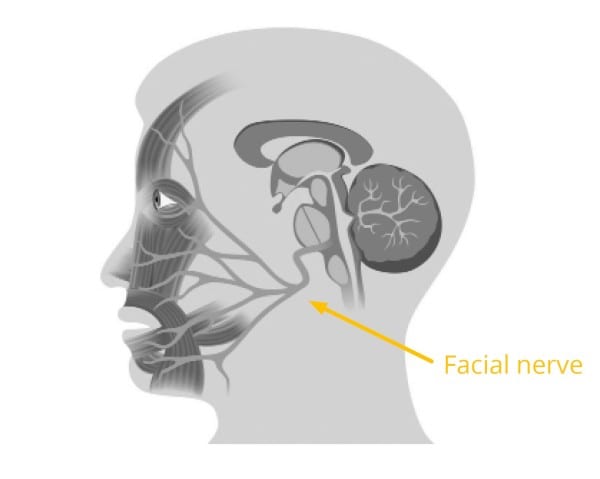
The facial nerve emerges from deep within the brainstem, leaves the skull slightly below the ear, and branches off to all muscles like a tree. Interestingly, the facial nerve is also wired up with much younger motor regions in our neo-cortex (neo as these areas are present only in mammalian brains), which are primarily responsible for facial muscle movements required for talking.
Did you know?
As the name indicates, the brainstem is an evolutionary very ancient brain area which humans share with almost all living animals.
Facial expressions and emotions
The facial feedback hypothesis: As ingeniously discovered by Fritz Strack and colleagues in 1988, facial expressions and emotions are closely intertwined. In their study, respondents were asked to hold a pen in their mouths while rating cartoons for their humor content. While one group held the pen between their teeth with lips open (mimicking a smile), the other group held the pen with their lips only (preventing a proper smile).
Here‘s what Fritz Strack found out: The first group rated the cartoon as more humorous. Strack and team took this as evidence for the facial feedback hypothesis postulating that selective activation or inhibition of facial muscles has a strong impact on the emotional response to stimuli.
Emotions, feelings, moods
What exactly are emotions?
In everyday language, emotions are any relatively brief conscious experiences characterized by intense mental activity and a high degree of pleasure or displeasure. In scientific research, a consistent definition has not been found yet. There’s certainly conceptual overlaps between the psychological and neuroscientific underpinnings of emotions, moods, and feelings.
Emotions are closely linked to physiological and psychological arousal with various levels of arousal relating to specific emotions. In neurobiological terms, emotions could be defined as complex action programs triggered by the presence of certain external or internal stimuli.
These action programs contain the following elements:
- Bodily symptoms such as increased heart rate or skin conductance. Mostly, these symptoms are unconscious and involuntary.
- Action tendencies, for example “fight-or-flight” actions to either immediately evade from a dangerous situation or to prepare a physical attack of the opponent.
- Facial expressions, for example baring one’s teeth and frowning.
- Cognitive evaluations of events, stimuli or objects.
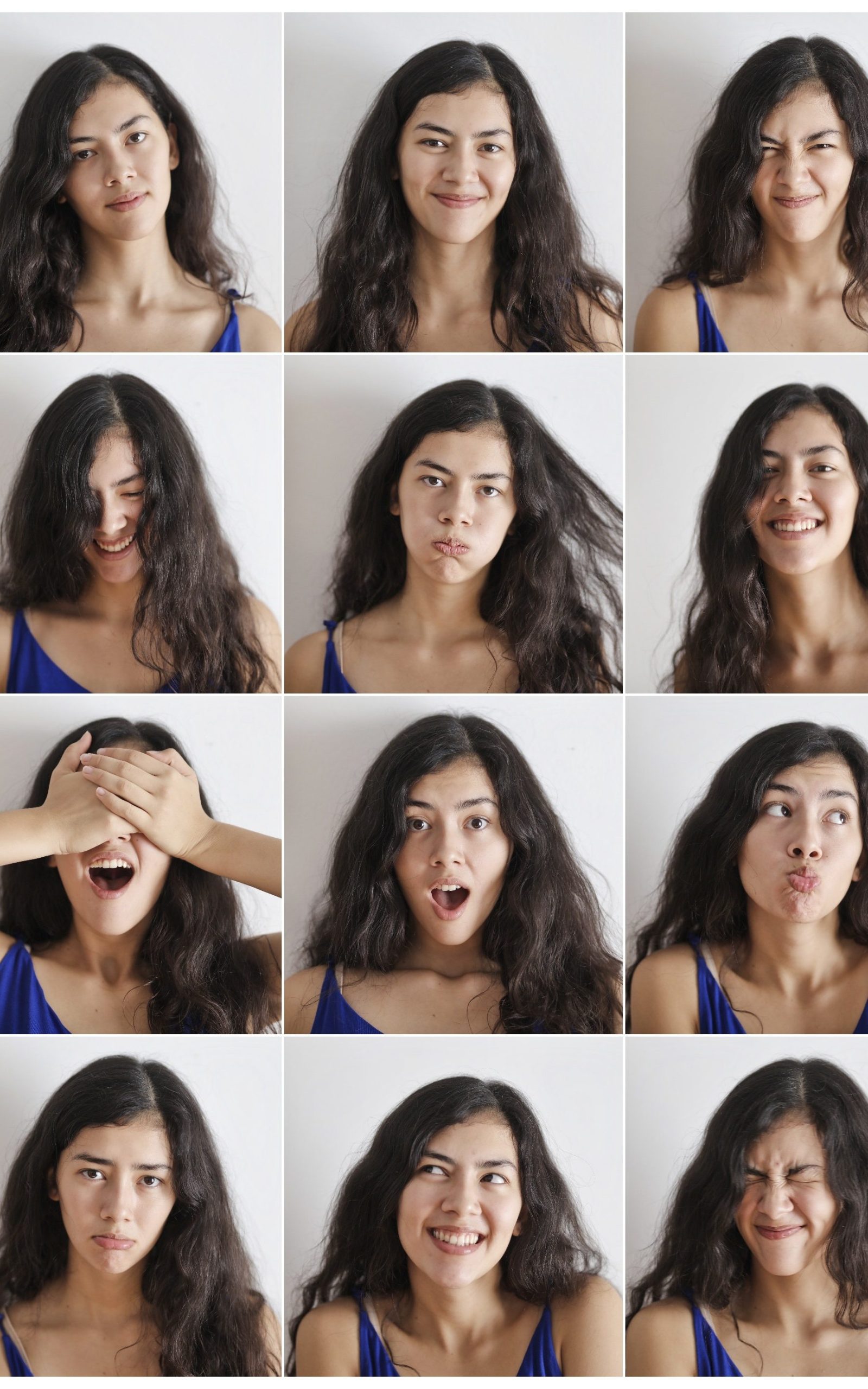
Emotions vs. feelings vs. moods
Feelings are subjective perceptions of the emotional action programs. Feelings are driven by conscious thoughts and reflections – we surely can have emotions without having feelings, however we simply cannot have feelings without having emotions.
Moods are diffuse internal, subjective states, generally being less intense than emotions and lasting significantly longer. Moods are highly affected by personality traits and characteristics. Voluntary facial expressions (smiling, for example) can produce bodily effects similar to those triggered by an actual emotion (happiness, for example).
Affect describes emotions, feelings and moods together.
Can you classify emotions?
Facial expressions are only one out of many correlates of emotion, but they might be the most apparent ones. Humans are obviously able to produce thousands of slightly varying sets of facial expressions – however, there is only a small set of distinctive facial configurations that almost every one associates with certain emotions, irrespective of gender, age, cultural background and socialization history.
These categorical emotions are:
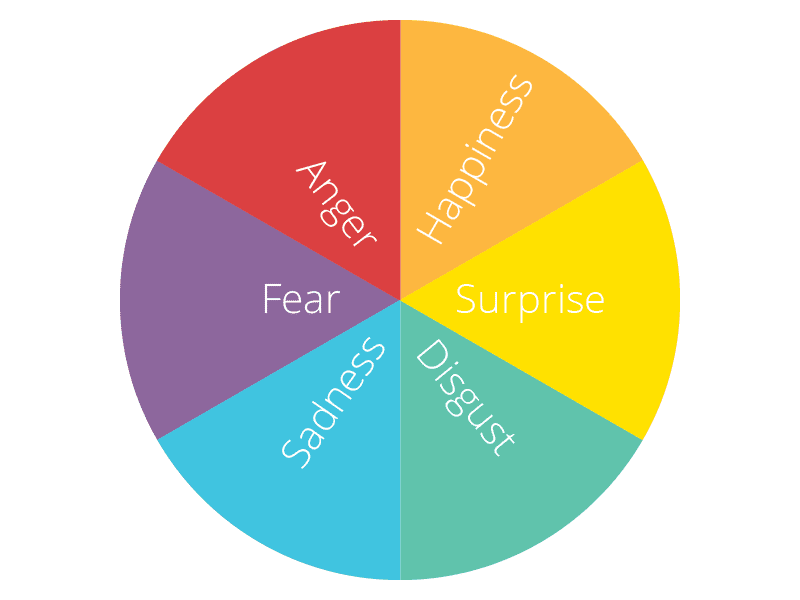
The finding that almost everyone can produce and recognize the associated facial expressions of these emotions has led some researchers to the (debated!) assumption that they are universal.
Check out our webinar on: What are emotions and How do we Measure Them
Facial expression analysis techniques
Facial expressions can be collected and analyzed in three different ways:
- By tracking of facial electromyographic activity (fEMG)
- By live observation and manual coding of facial activity
- By automatic facial expression analysis using computer-vision algorithms
Let‘s explain them in more detail.
Facial electromyography (fEMG)
With facial EMG you can track the activity of facial muscles with electrodes attached to the skin surface. fEMG detects and amplifies the tiny electrical impulses generated by the respective muscle fibers during contraction. The most common fEMG sites are in proximity to the following two major muscle groups:
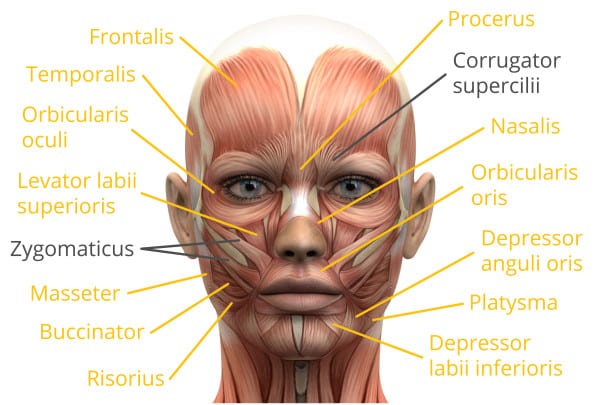
1. Right/left corrugator supercilii (“eyebrow wrinkler”):
This is a small, narrow, pyramidal muscle near the eye brow, generally associated with frowning. The corrugator draws the eyebrow downward and towards the face center, producing a vertical wrinkling of the forehead. This muscle group is active to prevent high sun glare or when expressing negative emotions such as suffering.
2. Right/left zygomaticus (major):
This muscle extends from each cheekbone to the corners of the mouth and draws the angle of the mouth up and out, typically associated with smiling.
The Facial Action Coding System (FACS)
The Facial Action Coding System (FACS) represents a fully standardized classification system of facial expressions for expert human coders based on anatomic features. Experts carefully examine face videos and describe any occurrence of facial expressions as combinations of elementary components called Action Units (AUs).
A common misbelief is that FACS is related to reading emotions. In fact, FACS is just a measurement system and does not interpret the meaning of the expressions. It’s like saying the purpose of riding a bike is to go to work. Sure, you can ride a bike to work, but you can use your bike for a lot of other things as well (for leisure, sports training, etc.). You get the idea.
However, during the analytical phase, the FACS system allows for a modular construction of emotions based on the combination of AUs.
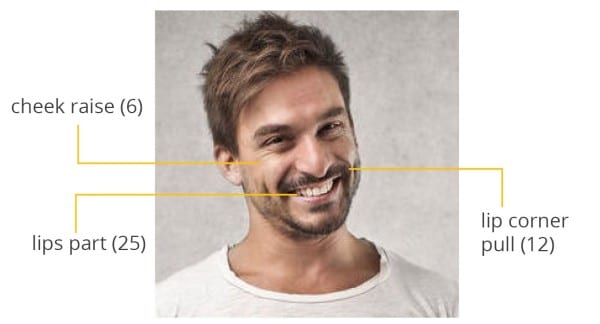
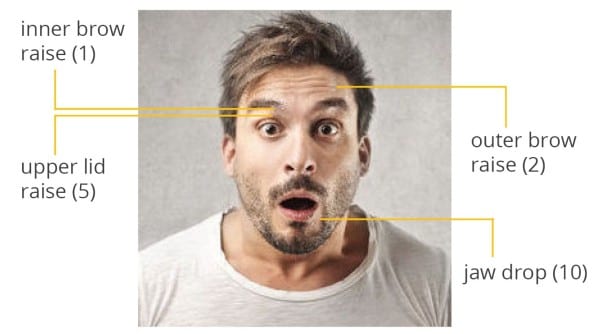
With facial action unit coding you can get all the knowledge to dissociate between the following three facial expression categories:
- Macroexpressions typically last between 0.5 – 4 seconds, occur in daily interactions, and generally are obvious to the naked eye.
- Microexpressions last less than half a second, occur when trying to consciously or unconsciously conceal or repress the current emotional state.
- Subtle expressions are associated with the intensity and depth of the underlying emotion. We’re not perpetually smiling or raising eyebrows – the intensity of these facial actions constantly varies. Subtle expressions denote any onset of a facial expression where the intensity of the associated emotion is still considered low.
Out of these limitations, a new generation of facial expression technologies has emerged being fully automated and computer-based.
Facial expression analysis Technology
Built on the groundbreaking research of core academic institutions in the United States and Europe, automatic facial expression recognition procedures have been developed and made available to the broader public, instantaneously detecting faces, coding facial expressions, and recognizing emotional states.
This breakthrough has been mainly enabled by the adoption of state-of-the-art computer vision and machine learning algorithms along with the gathering of high-quality databases of facial expressions all across the globe.
Did you know?
Automated facial coding has revolutionized the field of affective neuroscience and biosensor engineering, making emotion analytics and insights commercially available to the scientific community, commercial applications, and the public domain.
These technologies use cameras embedded in laptops, tablets, and mobile phones or standalone webcams mounted to computer screens to capture videos of respondents as they are exposed to content of various categories.
The use of inexpensive webcams eliminates the requirement for specialized high-class devices, making automatic expression coding ideally suited to capture face videos in a wide variety of naturalistic environmental settings such as respondents’ homes, workplace, car, public transportation, and many more.
Check out our webinar on Remote Research with iMotions: Facial Expression Analysis & Behavioral Coding from Your Couch
What exactly are the scientific and technological procedures under the hood of this magic black box?
The technology behind automatic facial coding
More or less, all emotion recognition engines comprise the same steps. Emotient FACET, for example, applies the following:
Face detection
The position of a face is found in a video frame or image, which can be achieved by applying the Viola Jones Cascaded Classifier algorithm, for example. This might sound sophisticated, but you can actually find this technology in the camera of your iPhone or Android smartphone as well. The result is a face box framing the detected face.
Feature detection
Within the detected face, facial landmarks such as eyes and eye corners, brows, mouth corners, the nose tip etc. are detected. After this, an internal face model is adjusted in position, size, and scale in order to match the respondent’s actual face. You can imagine this like an invisible virtual mesh that is put onto the face of the respondent: Whenever the respondent’s face moves or changes expressions, the face model adapts and follows instantaneously. As the name indicates, the face model is a simplified version of the respondent’s actual face. It has much less details, (so-called features) compared to the actual face, however it contains exactly those face features to get the job done. Exemplary features are single landmark points (eyebrow corners, mouth corners, nose tip) as well as feature groups (the entire mouth, the entire arch of the eyebrows etc.), reflecting the entire “Gestalt” of an emotionally indicative face area.
Feature classification
Once the simplified face model is available, position and orientation information of all the key features is fed as input into classification algorithms which translate the features into Action Unit codes, emotional states, and other affective metrics.
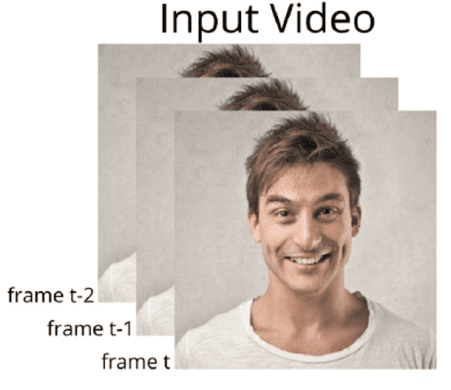
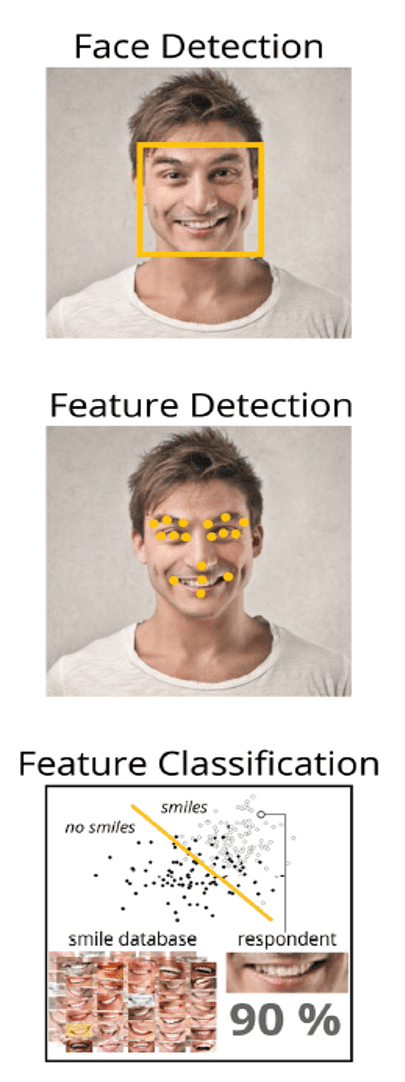

- Head orientation (yaw, pitch, roll)
- Interocular distance and 33 facial landmarks
- 7 basic emotions; valence, engagement, attention
- 20 facial expression metrics
Here’s a little theory (and analysis) behind it.
The translation from face features into metrics is accomplished statistically, comparing the actual appearance of the face and the configuration of the features numerically with the normative databases provided by the facial expression engines.
What does that mean? The current facial expression of a respondent is not compared one-by-one with all hundreds of thousands of pictures in the database – this would be quite tedious and take forever. Instead, the databases contain statistics and normative distributions of all feature characteristics across respondents from multiple geographic regions and demographic profiles as well as stimulus categories and recording conditions. Every facial expression analysis engine uses a different database. This is one of the reasons why you might get slightly varying results when feeding the very same source material into different engines.
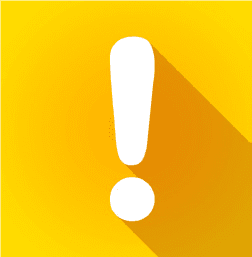
The classification is done on a purely statistical level. Here‘s an example: If the respondent’s mouth corners are pulled upward, a human coder would code this as activity of AU12 (“lip corner puller”) – stating that the respondent is smiling. The facial expression engine instead has to compute the vertical difference between mouth corners and mouth center, returning a value of 10 mm. This value is compared to all possible values in the database (values between 0 mm and 20 mm, for example).
In our example, the respondent’s expression apparently is right in the middle of the “smile” distribution (0 < 10 < 20 mm). For the engine it’s definitely safe to say that the current expression is stronger than a slight smile, however not as strong as a big smile.
In an ideal world, the classifier returns either “Yes” or “No” for each emotion, AU or metric. Unfortunately, it is not that easy. Take a look again at the “smile” classifier:
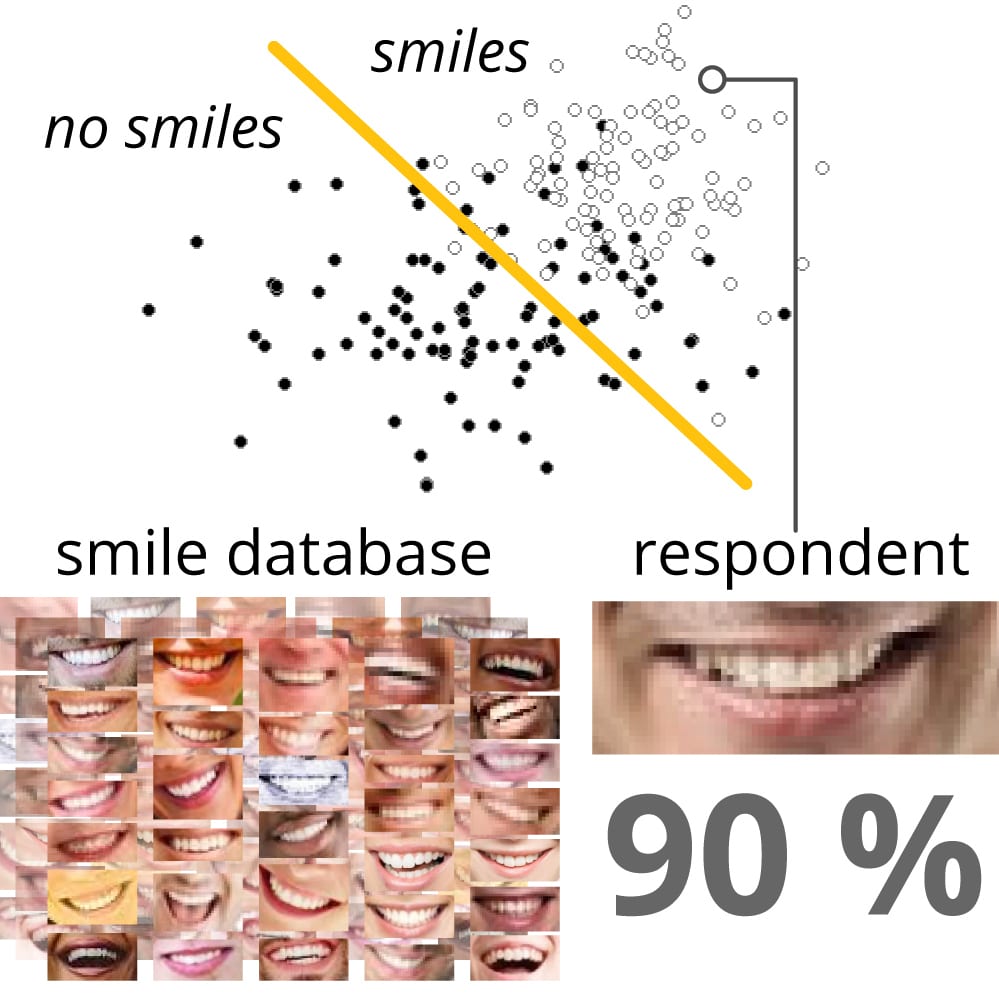
The filled dots (rather to the left) are all mouth configurations in the database that are not classified as smiles, while the white dots (rather towards the right) represent mouth configurations that are regarded as smiles.
The orange line denotes the classification criterion.
Everything on the left side of the line is classified as “not a smile”, whereas everything on the right side is classified as “smile”.
There obviously is a diffuse transition zone between non-smiles and smiles where some mouth configurations are misclassified – they might be subtle smiles that stay under the radar or other mouth opening configurations that might be rather yawns than smiles. As a consequence, the classifier can only return a probabilistic result, reflecting the likelihood or chance that the expression is an authentic “smile”.
In our example, the respondent’s smile is rather obvious, ending up all to the right side of the “feature space”. Here, the classifier is very certain about the result of a smile and returns a solid 90%. In other cases, the classifier might be less confident about its performance.
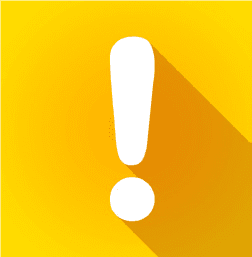
Feature classification is done for each emotion, Action Unit, and key feature independently – the classifier for smiles doesn’t know anything about the classifier for frowns, they are simply coded independently of each other. The good thing about this is that the automatic coding is accomplished much more objectively than manual coding where humans – particularly novice coders – tend to interpret the activation of an Action Unit in concert with other Action Units, which significantly alters the results.
Before we dig deeper into the visualization and analysis of the facial classification results, we will have a peak into some guidelines and recommendations on how to collect best-in-class data.
Equipment
Let‘s get practical.
What should you consider when acquiring high-quality data, what are optimal stimuli, what to keep in mind when recruiting and instructing respondents? We‘re here to help.
- For starters, automatic facial expression recognition can be accomplished online, that is, while respondents are exposed to emotional stimuli and their facial expressions are monitored (and/or recorded).
- Apart from online data collection, facial expression detection can be applied offline, for example to process facial captures that have been recorded before. You can even record a larger audience and, with minimal editing, analyze each respondents’ facial expressions.
For online automatic facial coding with webcams, keep the following camera specifications in mind:
- Lens: Use a standard lens; avoid wide-angle or fisheye lenses as they may distort the image and cause errors in processing.
- Resolution: The minimum resolution varies across facial expression analysis engines. You certainly don’t need HD or 4K camera resolutions – automated facial expression analysis already works for low resolutions as long as the respondent’s face is clearly visible.
- Autofocus: You certainly want to use a camera that is able to track respondent’s faces within a certain range of distance. Some camera models offer additional software-based tracking procedures to make sure your respondents don’t get out of focus.
- Aperture, brightness and white balance: Generally, most cameras are able to automatically adjust to lighting conditions. However, in case of backlighting the gain control in many cameras reduces image contrast in the foreground, which may deteriorate performance.
Did you know?
Some camera might dynamically adjust the frame number dependent on lighting conditions (they reduce the frame rate to compensate for poor lighting, for example), leaving you with a video file with a variable frame rate. This certainly is not ideal as you will end up with variable frames per second, making it harder to compare and aggregate the results across respondents.
A word on webcams: We suggest to use any HD webcam. You can of course accomplish automatic facial expression analysis with any other camera including smartphone cameras, IP cameras or internal laptop webcams (dependent on your application). However, be aware that these cameras generally come with limited auto-focus, brightness, and white balance compensation capabilities.
Check out: How Facial Expressions Analysis can be done remotely
Facial Expression Analysis (FEA): Application fields
With facial expression recognition you can test the impact of any content, product or service that is supposed to elicit emotional arousal and facial responses – physical objects such as food probes or packages, videos and images, sounds, odors, tactile stimuli, etc. Particularly involuntary expressions as well as a subtle widening of the eyelids are of key interest as they are considered to reflect changes in the emotional state triggered by actual external stimuli or mental images.
Now which fields of commercial and academic research have been adopting emotion recognition techniques lately? Here is a peek at the most prominent research areas:
1. Consumer neuroscience and neuromarketing
There is no doubt about it: Evaluating consumer preferences and delivering persuasive communication are critical elements in marketing. While self-reports and questionnaires might be ideal tools to get insights into respondents’ attitudes and awareness, they might be limited in capturing emotional responses unbiased by self-awareness and social desirability. That‘s where the value of emotion analytics comes in: Tracking facial expressions can be leveraged to substantially enrich self-reports with quantified measures of more unconscious emotional responses towards a product or service. Based on facial expression analysis, products can be optimized, market segments can be assessed, and target audiences and personas can be identified. There‘s quite a lot that facial expression analysis can do for you to enhance your marketing strategy – just think about it!
2. Media testing & advertisement
In media research, individual respondents or focus groups can be exposed to TV advertisements, trailers, and full-length pilots while monitoring their facial expressions. Identifying scenes where emotional responses (particularly smiles) were expected but the audience just didn’t “get it” is as crucial as to find the key frames that result in the most extreme facial expressions.
In this context, you might want to isolate and improve scenes that trigger unwanted negative expressions indicating elevated levels of disgust, frustration or confusion (those kind of emotions wouldn‘t exactly help a comedy show to become a hit series, would they?) or utilize your audience‘s response towards a screening in order to increase the overall level of positive expressions in the final release.
3. Psychological research
Psychologists analyze facial expressions to identify how humans respond emotionally towards external and internal stimuli. In systematic studies, researchers can specifically vary stimulus properties (color, shape, duration of presentation) and social expectancies in order to evaluate how personality characteristics and individual learning histories impact facial expressions.
4. Clinical psychology and psychotherapy
Clinical populations such as patients suffering from Autism Spectrum Disorder (ASD), depression or borderline personality disorder (BPD) are characterized by strong impairments in modulating, processing, and interpreting their own and others’ facial expressions. Monitoring facial expressions while patients are exposed to emotionally arousing stimuli or social cues (faces of others, for example) can significantly boost the success of the underlying cognitive-behavioral therapy, both during the diagnostic as well as the intervention phase. An excellent example is the “Smile Maze” as developed by the Temporal Dynamics of Learning Center (TDLC) at UC San Diego. Here, autistic children train their facial expressions by playing a Pacman-like game where smiling steers the game character.
5. Medical applications & plastic surgery
The effects of facial nerve paralysis can be devastating. Causes include Bell’s Palsy, tumors, trauma, diseases, and infections. Patients generally struggle with significant changes in their physical appearance, the ability to communicate, and to express emotions. Facial expression analysis can be used to quantify the deterioration and evaluate the success of surgical interventions, occupational and physical therapy targeted towards reactivating the paralyzed muscle groups.
6. Software UI & website design
Ideally, handling software and navigating websites should be a pleasant experience – frustration and confusion levels should certainly be kept as low as possible. Monitoring facial expressions while testers browse websites or software dialogs can provide insights into the emotional satisfaction of the desired target group. Whenever users encounter road blocks or get lost in complex sub-menus, you might certainly see increased “negative” facial expressions such as brow furrowing or frowning.
7. Engineering of artificial social agents (avatars)
Until recently, robots and avatars were programmed to respond to user commands based on keyboard and mouse input. Latest breakthroughs in hardware technology, computer vision, and machine learning have laid the foundation for artificial social agents, who are able to reliably detect and flexibly respond to emotional states of the human communication partner. Apple’s Siri might be the first generation of emotionally truly intelligent machines, however computer scientists, physicians, and neuroscientists all over the world are working hard on even smarter sensors and algorithms to understand the current emotional state of the human user, and to respond appropriately.
How to do it right with iMotions
N.B. This is an excerpt from our free guide “Facial Expression Analysis – The Complete Pocket Guide”. To get the full guide with further information on stimulus setup, data output & visualization, additional biosensors, and “how to do it right with iMotions”, click the banner below. If you have any questions regarding facial expression analysis please feel free to contact us.
Free 42-page Facial Expression Analysis Guide
For Beginners and Intermediates
- Get a thorough understanding of all aspects
- Valuable facial expression analysis insights
- Learn how to take your research to the next level
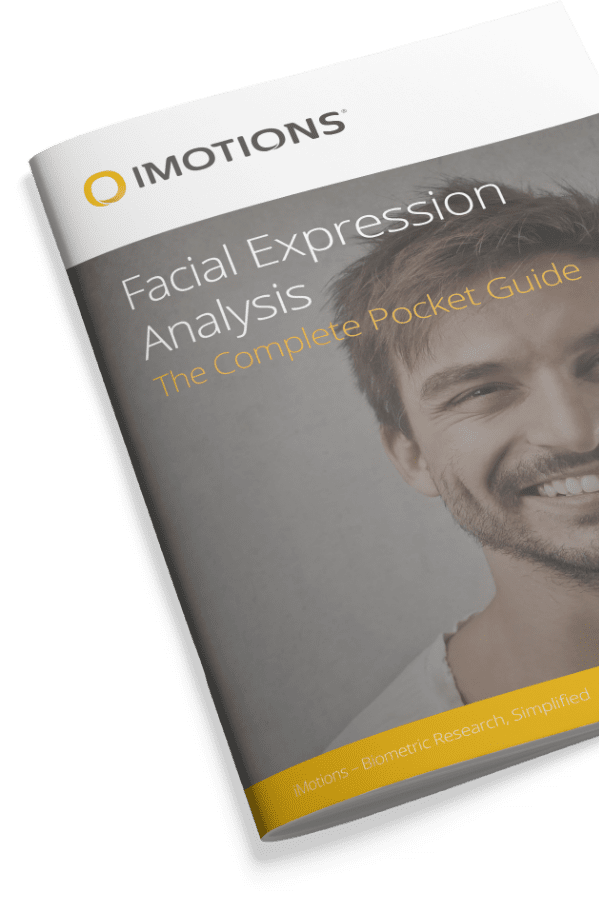
Psychology Research with iMotions
The world’s leading human behavior software