Mental Workload (MWL) has grown into an important variable with which we understand user performance in HCI usability design, automotive design, human factors research, ergonomics research, and more. This article gives a brief introduction to MWL and the possibilities of exploring it with iMotions.
What is Mental Workload?
The term MWL can be defined from a variety of different perspectives. From a psychological background, MWL can be referred to as task switching or allocation of attention (Wickens, 2008); however, from a system design perspective, it can be defined as a demand placed on the user by the system. In the systematic review (Charles & Nixon, 2019), the term “Taskload” and “MWL” were distinguished like this: “Taskload” can be originating from the work, for example, the number of tasks whereas “MWL” encompasses the subjective experience of a given Taskload. Here the definition of MWL is mediated by many factors, with Taskload being just one. One example to illustrate this is if you are repeating a simple task (Taskload is low) but then given a time pressure, you will feel stressed, and MWL is thus increased.
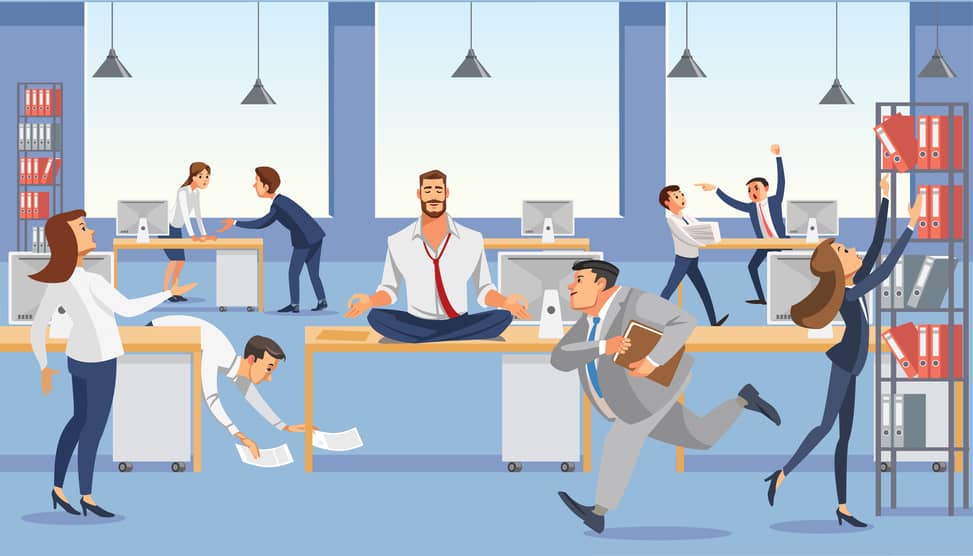
What Application Domains Mental Workload Has been Studied For?
One can see MWL has been widely studied in multiple domains across simulated flight tasks, actual flight, air traffic control (ATC), simulated nuclear control tasks, and simulated driving tasks. The type of tasks can vary from desk-based tasks such as mental arithmetic to varied tasks such as the Multi-Attribute Task Battery (MATB). These conditions all share the potential of exceeding a man’s capability to attend to and process information. Identifying MWL is an important source of monitoring or even predicting performance errors and optimizing the structure of task demands or environmental stressors.
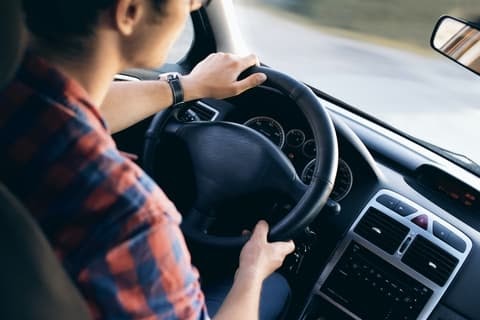
How To Measure Mental Workload?
The evidence of measurement of mental workload using major physiological measures has been reported. In a systematic review presented in (Charles & Nixon, 2019), physiological signals like ECG, blink rate, EEG from peer-reviewed literature across multiple domains were presented as to how they reflect MWL.
Electrocardiac Activity
It has been discovered that Heart Rate (HR) measured from ECG increased with increasing task demands (De Rivecourt et al., 2008). Not only the task load conditions but also the task type has been reported to give differences in HR changes: Sosnowski et al. (2004) saw a larger increase in HR during tasks requiring problem-solving compared to tasks requiring logical completion of a series of elements.
iMotions ECG Heart Rate and Heart Rate Variability R Notebook will facilitate your computation from raw ECG signal (which you collect through ECG devices in iMotions) to HR. The HR data will be displayed both in time-based signal and single value metrics, for your further comparison and interpretation. If you want to know more about our R Notebook – a transparent, flexible R-based signal processing tool in iMotions, please refer to here.
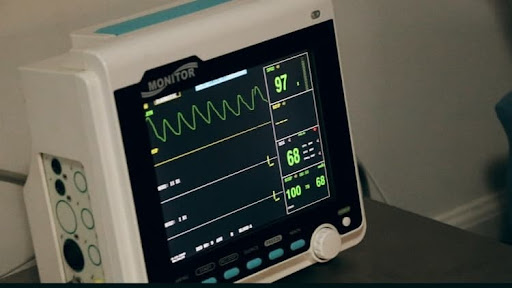
Blink Rate
Blink rate has been shown to be sensitive when it comes to visual workload, so may be used to measure MWL when a person is presented with visual stimuli. For example, during an air traffic control (ATC) task (Brookings et al., 1996), a simulated flight task (Veltman and Gaillard, 1996), or actual flight (Wilson, 2002), the increased visual demand led to lower blink rates. Values in blink rate for reflecting MWL, however, factors like light, air quality, and air conditioning could have significant effects on measures across all reports.
iMotions has incorporated blink detection R Notebooks based on both eye tracking data and facial expression data, which you can collect in the iMotions platform directly. The blinks will be detected and blink rate will be calculated by R Notebook. It is also possible to export these results into a summary format file, for further analysis and comparison. If you want to know more about our R Notebook – a transparent, flexible R-based signal processing tool in iMotions, you can read more here.
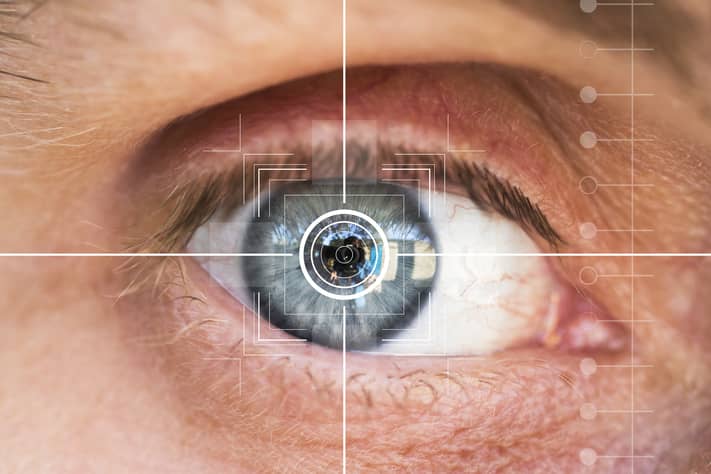
Brain Activity
Generally, EEG frequencies have been seen to change during changes in task demand. Specifically, during tasks of a multi attribute test, theta activity was seen to increase during high demand tasks compared to low demand tasks (Fairclough et al., 2005). In tasks like memory tasks (Klimesch, 1997), high workload multi-task test (Fournier et al., 1999), or take-off and landing phases of actual flight (Wilson, 2002), a decrease in alpha power has been observed.
Changes in room ambiance, such as temperature, can also have an effect on mental workload. You can read more about that here.
iMotions EEG R Notebooks provide flexibility, transparency, and speed for computing power data from the popularly used powerbands: delta, theta, alpha, beta, and gamma. The computation is done on the raw EEG data that you are able to collect directly in the iMotions platform. The subsequent results can be viewed both in the software’s replay function and summarized in exported .CSV files, to better facilitate further analysis. It is important to note that a careful study design, good quality data collection, and proper signal processing steps are all keys to meaningful and interpretable EEG results, based on any set of conducted research.
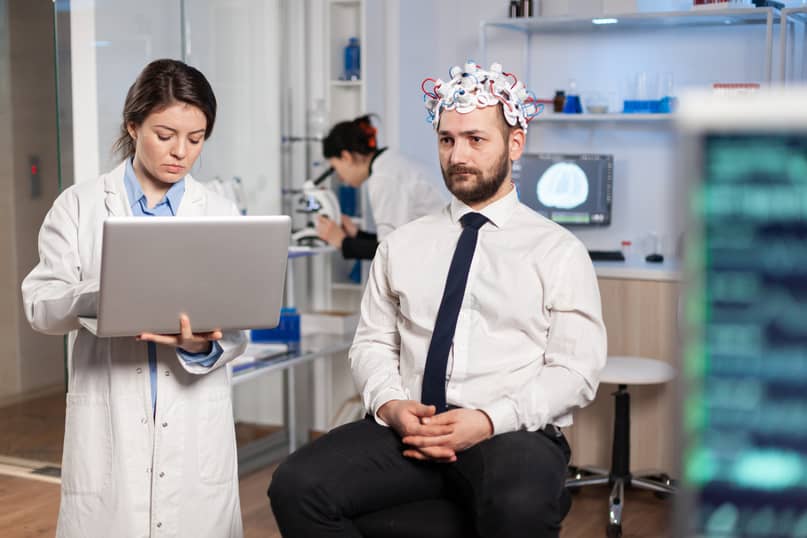
For more inspiration on how to conduct EEG research with the iMotions software suite, please refer to our blogs on the subject:
The Anatomy of an EEG Cap,
5 Basics of EEG 101: Data Collection, Processing & Analysis
Respiration
Backs et al. (2000) and (Brookings et al., 1996) reported an increased respiration rate which correlated with an increased level of difficulty during simulated Air Traffic Control tasks (ATC). The increase in respiration rate may be interpreted as a direct result of the increased metabolic demands that are required to perform certain tasks.
The metabolic rate was also found to be higher in poorer performers than good performers in Backs et al. (1994) that used a memory task as the stimulus. However, be cautious when considering respiratory measures while speech or exertion are involved since these types of measurements are highly dependent on physical activity (Grassmann et al., 2016).
iMotions Respiration Processing R Notebook will facilitate your computation from raw respiration signal (which you collect through a respiration device in iMotions) to metrics like how fast a person breathes or how long each breath takes. The subsequent results can be viewed both in the software’s replay function and summarized in exported .CSV files, to better facilitate further analysis. If you want to know more about our R Notebook – a transparent, flexible R-based signal processing tool in iMotions, please refer to it here.
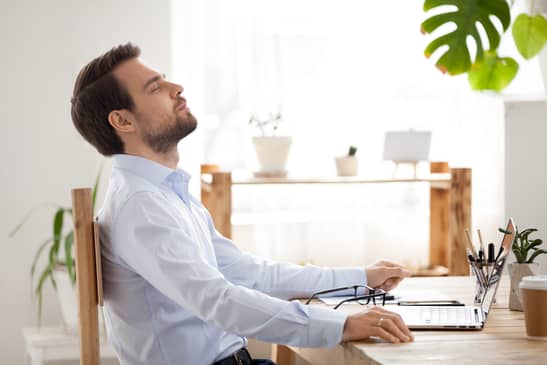
References
[1] Wickens, Christopher D., 2008. Multiple resources and mental workload. Hum. Factors 50 (3), 397–403.
[2] Charles, R. L., & Nixon, J. (2019). Measuring mental workload using physiological measures: A systematic review. Applied Ergonomics, 74, 221–232. https://doi.org/10.1016/j.apergo.2018.08.028
[3] De Rivecourt, M., et al., 2008. Cardiovascular and eye activity measures as indices for momentary changes in mental effort during simulated flight. Ergonomics 51 (9), 1295–1319.
[4] Sosnowski, T., et al., 2004. Program running versus problem solving: mental task effect on
tonic heart rate. Psychophysiology 41 (3), 467–475.
[5] Brookings, J.B., Wilson, G.F., Swain, C.R., 1996. Psychophysiological responses to
changes in workload during simulated air traffic control. Biol. Psychol. 42 (3), 361–377
[6] Veltman, J.A., Gaillard, W.K., 1996. Physiological indices of workload in a simulated
flight task. Biol. Psychol. 42 (3), 323–342
[7] Wilson, G.F., 2002. An analysis of mental workload in pilots during flight using multiple
psychophysiological measures. Int. J. Aviat. Psychol. 12 (1), 3–18.
[8] Fairclough, S.H., et al., 2005. The influence of task demand and learning on the psychophysiological response. Int. J. Psychophysiol. 56 (2), 171–184.
[9] Klimesch, W., 1997. EEG-alpha rhythms and memory processes. Int. J. Psychophysiol. 26
(1–3), 319–340. https://doi.org/10.1016/S0167-8760(97)00773-3 , Accessed date: 1
June 2016
[10] Fournier, L.R., et al., 1999. Electrophysiological, behavioral, and subjective indexes of
workload when performing multiple tasks: manipulations of task difficulty and
training. Int. J. Psychophysiol. 31, 129–145.
[11] Backs, R.W., et al., 2000. Cardiorespiratory indices of mental workload during simulated
air traffic control. In: Proceedings of the IEA 2000/HFES 2000 Congress, vol. 3. Pp. 89–92.
[12] Brookings, J.B., Wilson, G.F., Swain, C.R., 1996. Psychophysiological responses to
changes in workload during simulated air traffic control. Biol. Psychol. 42 (3), 361–377.
[13] Backs, R.W., 1994. Metabolic and cardiorespiratory measures of mental effort: the effects
of level of difficulty in a working memory task. Int. J. Psychophysiol. 16 (1), 57–68.
[14] Grassmann, Mariel, Vlemincx, Elke, von Leupoldt, Andreas, Mittelstädt, Justin M., Van
den Bergh, Omer, 2016. Respiratory changes in response to cognitive load: a systematic review. Neural Plast. 2016, 8146809 16 pages. https://doi.org/10.1155/2016/8146809