What happens when you smile? To the outside world, it’s obvious that the muscles in the corners of your lips are pulled up, and your cheeks raise, but there’s even more going on than meets the eye. Your pupils might dilate, your heart rate can momentarily slow down, and the sweat glands on the surface of your skin might spark to life [1,2,3]. When learning about and decoding emotions, this information is priceless – but without specialized technology, so much of it is hidden away. This is where emotion recognition technology comes in.
Emotion recognition technology describes a collection of hardware and software that has been built to detect exhibited human emotions – in all their complexity – and convert that into data. This data can then be analysed, and even acted upon by a computer. It might sound like science fiction, but it’s becoming everyday reality.
In the case of the smile described above, the emotion could be recognised by facial expression analysis (just requiring a camera and specialized software), fEMG (facial electromyography), ECG (electrocardiography), and EDA (electrodermal activity). Not all of it is necessarily needed at the same time though, but each can add a new layer to decoding human emotions.
Why does this technology matter? For most people, spotting an emotion can seem like a trivial task, but as we spend more of our lives enveloped in technology, it’s important that this very technology can carry that out too. Below, we will go through some examples that show why – and make some predictions for where the technology is heading.
Emotion recognition technology in the automotive industry
One of the biggest adopters of emotion recognition technology is the automotive industry. With over 287 million cars in the US, and an average amount of time spent in them equalling just over 8 hours a week, there is a clear impetus to improve the driving experience. Car manufacturers can stand out from the crowd by creating experiences that enhance safety and make driving more enjoyable.
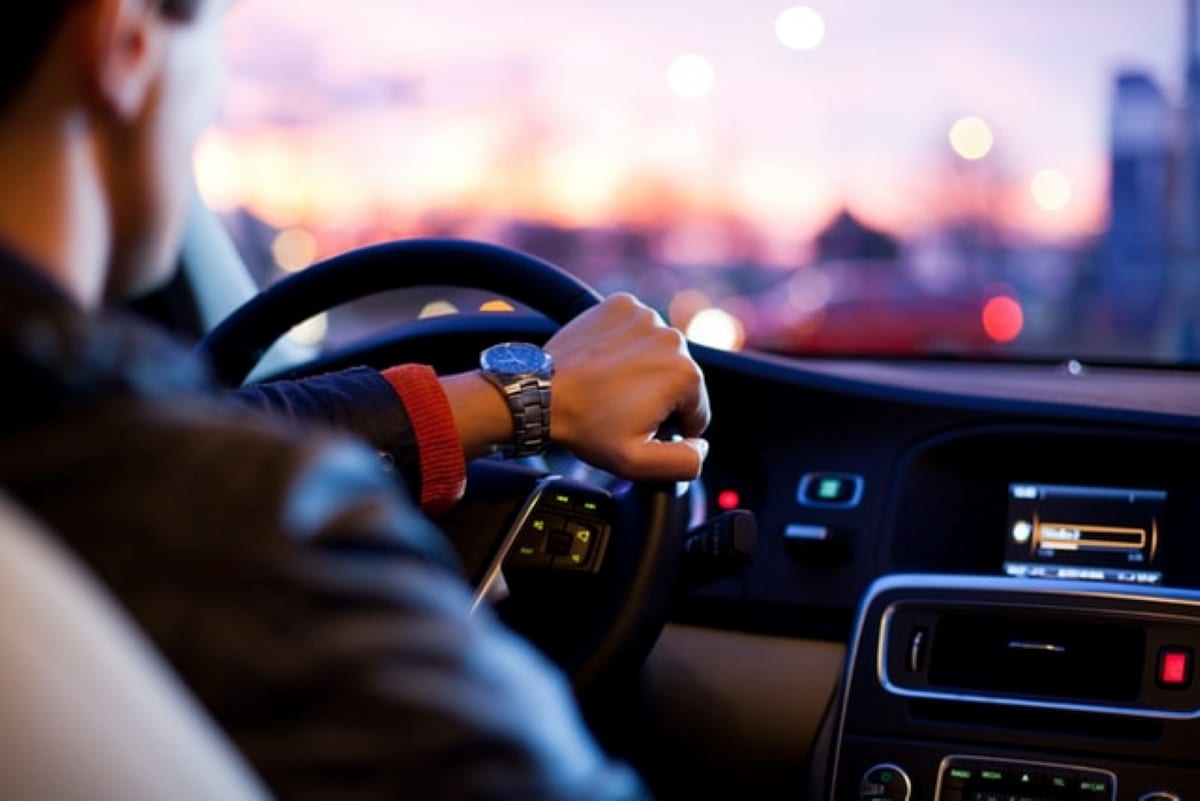
One clear target for emotion recognition technology is that of driver drowsiness – while not an emotion per se, each biosensor is perfectly equipped to detect the onset of drowsiness. Over 100,000 accidents occur each year in the US alone as a result of impaired attention caused by such drowsiness – a great portion of which could be prevented by built-in systems that can alert the driver if they begin to nod off at the wheel. Emotion recognition technology can detect this through a range of methods – from dashboard cameras carrying out eye tracking or facial expression analysis, built-in electrodermal activity sensors [4,5], or even EEG sensors [6].
Each sensor is able to provide an accurate detection of increasing sleepiness, which could trigger an alert system for the driver to pull over. Going one step further, these systems could also detect how emotions change at the wheel – responding to the onset of road rage, for example. These systems are well placed to create a safer driving experience for everyone on the road.
With the rise of autonomous cars, it’s easy to see how less time in a car will actually be spent driving – without the restriction of having to be at the wheel, a car can be transformed into a different kind of space. Emotion recognition technology can, in the future, enhance such spaces to make them more responsive, and ultimately, more human.
Emotion recognition technology in healthcare
Emotion recognition technology is also being widely employed within healthcare, for improving both diagnosis and treatment of patients. A prime example of this is its use with early detection of autism. Autism is a neurodevelopmental disorder that effects just under 1% of children, and is mainly characterised by social communication difficulties (for both speech and nonverbal communication), as well as engaging in repetitive behaviors. The Janssen Autism Knowledge Engine, powered by iMotions, uses a multisensor approach to discover the earliest points at which autism may be detectable in children [7].
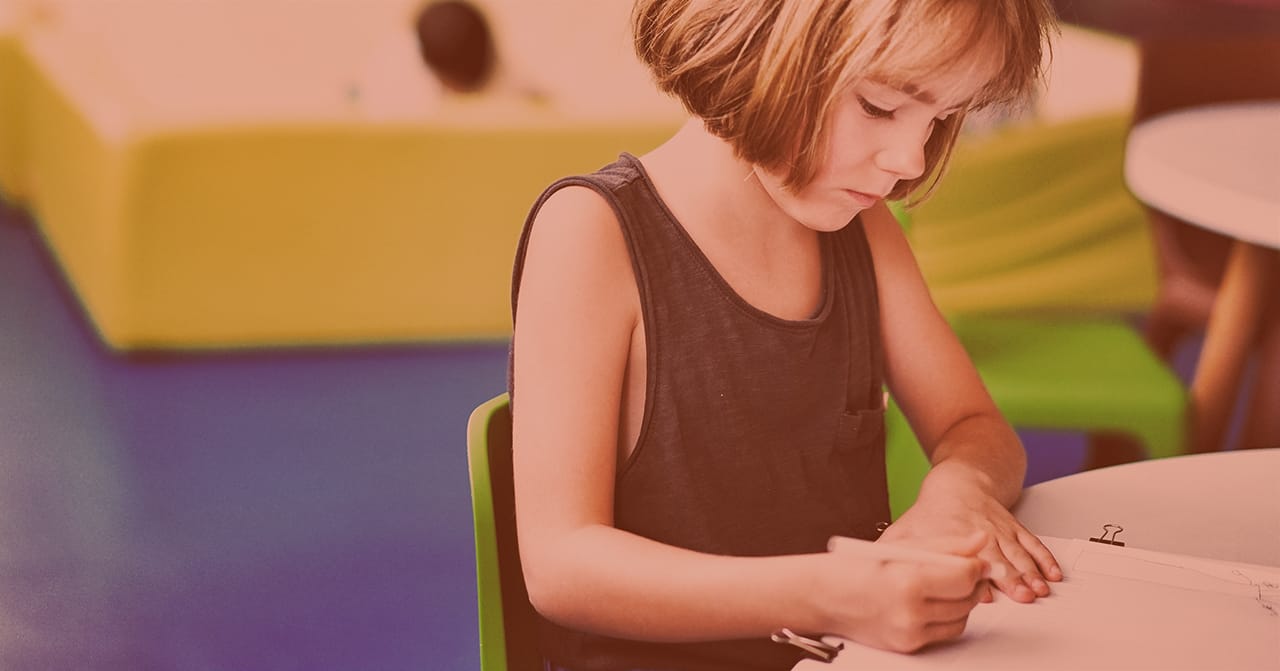
As early interventions for autism have been found to have the greatest impact on later social capabilities, early diagnosis can help ensure that children with autism receive the best care possible [8]. Beyond this, emotion recognition technology is also helping individuals with autism improve their social capabilities later in life, either with game-based training using facial expression analysis [9], or with on-the-fly guidance for emotional responses [10].
Emotion recognition technology has also been applied in a therapeutic context, to help individuals suffering from social anxiety. A recent research project, using iMotions, combines ECG, EDA, and eye tracking to monitor how patients respond to socially stressful virtual environments. The patients are provided with guidance on how to handle their stress levels, with the long-term goal being that “…anxious patients can bring the VR equipment home and thus the treatment can be made more personal, flexible and inexpensive” (Mia Beck Lichtenstein, head of the Centre for Telepsychiatry in Southern Denmark, director of the project).
Emotion recognition technology for training, simulations, and gaming
Outside of the clinic, emotion recognition technology is also being used to train the emotional responses of individuals in challenging situations. For example, flight simulations can use emotion recognition technology in a similar manner as it’s applied with driving – to detect drowsiness or maladaptive emotional states. Better training practices can be employed by understanding how pilots emotionally react in-flight [11].
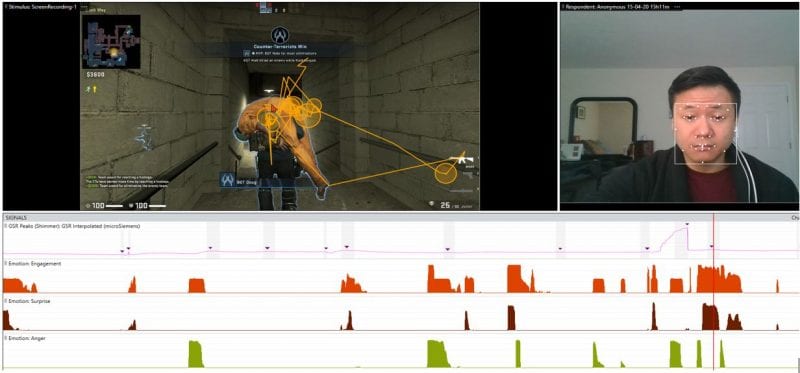
Assessing emotional reactions has also been carried out to improve empathic responses in virtual healthcare simulations [12, 13]. By providing detailed, objective feedback about patient care, practitioners can better understand how to create safer and more comfortable environments for patients. Virtual environments have also used emotion recognition technology for gaming, in which the gameplay can be altered, depending on the emotion of the player. By tracking EMG (electromyography), and EDA data, researchers have been able to track real-time emotions, which can then be used to modify the experienced environment [14, 15]. Future gameplay may come to be more adaptive to the emotions of the player, creating truly responsive, and immersive experiences.
Conclusion
Emotion recognition technology is coming of age. This has required advances in the last decade not only from the biosensor hardware, but also from software, and the algorithms that guide the emotion evaluation. A better understanding of how combinations of biosensors can provide instructive emotion data has also helped progress this technology.
While we cover some examples of emotion recognition technology in use today, there are plenty more arenas in which this technology is applied, and many more that will see such an implementation in the near future. Future technology has always been getting smarter, but now it’s also becoming more human.
Further Reading
If you are interested in finding out more about what emotion recognition technology can do to enhance your research, download our comprehensive Complete Pocket Guide on human behavior below:
Free 52-page Human Behavior Guide
For Beginners and Intermediates
- Get accessible and comprehensive walkthrough
- Valuable human behavior research insight
- Learn how to take your research to the next level
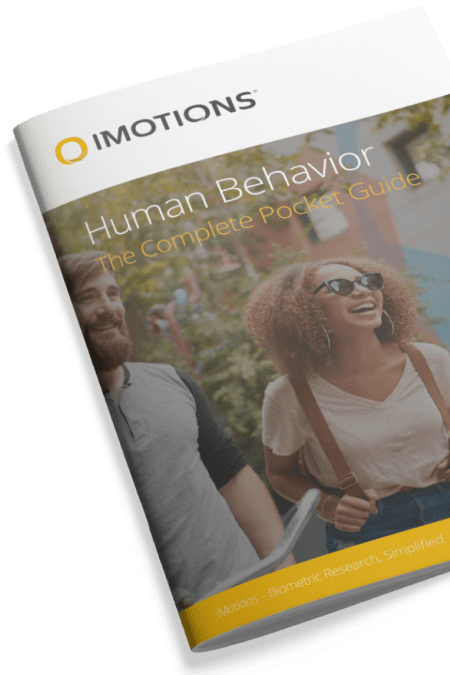
References
[1] Chołoniewski, J., Chmiel, A., Sienkiewicz, J., Hołyst, J., Küster, D., & Kappas, A. (2016). Temporal Taylor’s scaling of facial electromyography and electrodermal activity in
the course of emotional stimulation. Chaos, Solitons & Fractals, 90, 91–100. https://doi.org/10.1016/j.chaos.2016.04.023.
[2] Alghowinem, S., AlShehri, M., Goecke, R., & Wagner, M. (2014). Exploring eye activity as an indication of emotional states using an eye-tracking sensor. In L. Chen, S. Kapoor, & R. Bhatia (Eds.), Intelligent systems for science and information (pp. 261–276). Cham, Switzerland: Springer International. https://dx.doi.org/10.1007/978-3-319-04702-7_15
[3] Anttonen, J. And Surakka, V. (2005). Emotions and heart rate while sitting on a chair. In Proceedings of the ACM Conference on Human Factors in Computing Systems. ACM, New York,
491–499
[4] Ooi, J.S.K. Ahmad, S.A. Chong, Y.Z. Ali, S.H.M. Ai, G., Wagatsuma, H. (2016). Driver emotion recognition framework based on electrodermal activity measurements during simulated driving conditions. Proceedings of the 2016 IEEE EMBS Conference on Biomedical Engineering and Sciences (IECBES), pp. 365–369.
[5] Kajiwara, S. (2014). Evaluation of driver’s mental workload by facial temperature and electrodermal activity under simulated driving conditions. Int. J. Automot. Technol. 15, 65–70.
[6] Zander, T. O., Andreessen, L. M., Berg, A., Bleuel, M., Pawlitzki, J., Zawallich, L., et al. (2017). Evaluation of a dry EEG system for application of passive brain-computer interfaces in autonomous driving. Front. Hum. Neurosci. 11:78. doi: 10.3389/fnhum.2017.00078
[7] Ness SL, Bangerter A, Manyakov NV, Lewin D, Boice M, Skalkin A, et al. (2019). An observational study with the Janssen Autism Knowledge Engine (JAKE®) in individuals with Autism Spectrum Disorder. Front Neurosci, 13:111.
[8] McEachin, S. J., Smith, T., & Lovaas, O. I. (1993). Long-term outcome for children with autism who receive early intensive behavioural treatment. American Journal of Mental Retardation, 97, 359–372 (and Discussion, pp. 373–391).
[9] Garcia-Garcia, J. M., Penichet, V. M. R., Lozano, M. D. & Fernando, A. (2021). Using emotion recognition technologies to teach children with autism spectrum disorder how to identify and express emotions. Universal Access in the Information Society, https://doi.org/10.1007/s10209-021-00818-y
[10] M. Alharbi and S. Huang. (2020). An Augmentative System with Facial and Emotion Recognition for Improving Social Skills of Children with Autism Spectrum Disorders. 2020 IEEE International Systems Conference (SysCon), pp. 1-6, doi: 10.1109/SysCon47679.2020.9275659.
[11] César Cavalcanti Roza, V., & Adrian Postolache, O. (2019). Multimodal Approach for Emotion Recognition Based on Simulated Flight Experiments. Sensors, 19(24), 5516. https://doi.org/10.3390/s19245516
[12] Mano, Leandro & Mazzo, Alessandra & Torres Neto, José & Meska, Mateus & Giancristofaro, Gabriel & Ueyama, Jó & Pereira Junior, Gerson. (2019). Using emotion recognition to assess simulation-based learning. Nurse Education in Practice. 36. 10.1016/j.nepr.2019.02.017.
[13] Schreckenbach, T., Ochsendorf, F., Sterz, J. et al. Emotion recognition and extraversion of medical students interact to predict their empathic communication perceived by simulated patients. BMC Med Educ, 18, 237 (2018). https://doi.org/10.1186/s12909-018-1342-8
[14] A. Nakasone, H. Prendinger, & M. Ishizuka. (2005). Emotion recognition from electromyography and skin conductance. Proc. Int. Workshop on Biosignal Interpretation, pp. 219–222.
[15] Garner, T., & Grimshaw, M. N. (2013). The physiology of fear and sound: working with biometrics toward automated amotion recognition in adaptive gaming system. Proceedings of IADIS International Journal, Vol. 11. No. 2. Pp. 77-91. ISSN: 1645-7641
[16] H. Yong, J. Lee and J. Choi. (2019). Emotion Recognition in Gamers Wearing Head-mounted Display. 2019 IEEE Conference on Virtual Reality and 3D User Interfaces (VR), pp. 1251-1252, doi: 10.1109/VR.2019.8797736.