Of all the muscles in the face, the zygomaticus major is perhaps the most noticeable. Sitting between the corners of our lips and the upper part of our cheeks, it controls the way in which we smile.
The muscle sits atop the zygomatic bone, otherwise known as the cheekbone. Variations in the zygomatic muscle are also known to cause dimples when smiling. All of this goes to say that this area has a lot to do with our appearances, when active and inactive.
The activation of these muscles (on both sides of the face) creates a smile, whether spontaneous and genuine (a “Duchenne” smile) or planned and deliberate (a “non-Duchenne” smile).
A genuine Duchenne smile is created by both the activation of the zygomaticus major and the orbicularis oculi (the muscle the sits around the lips and causes the raising of the cheek), as well as the potential involvement of several other facial muscles. The activation of both of these muscles causes a smile that also involves the cheeks raising around the eye. A non-Duchenne smile involves activation of the zygomaticus major without activation of the orbicularis oculi.
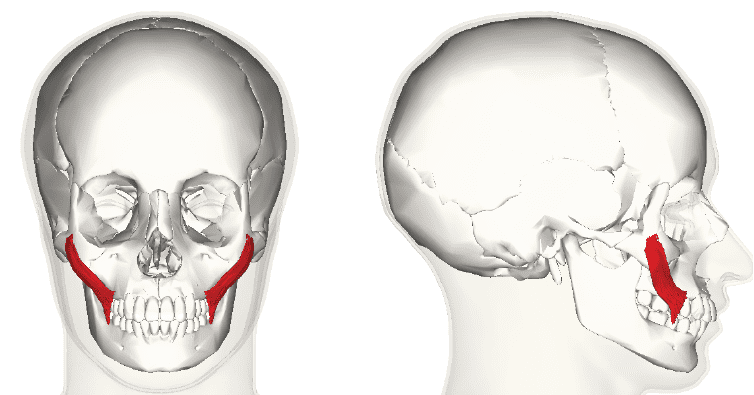
The muscle of course relates to more than just our appearances and how we’re perceived, but also how we emotionally relate to the world. Below, we will go through how to measure the activity of this muscle, and what it means for the person showing activity within this region.
fEMG
The most common way to specifically measure activity within this region is to use fEMG (facial electromyography). By placing the electrodes along the muscle, the activity can be readily measured. Electric impulses that result in muscle activation are detected by the electrodes. Research has shown how this measure is related to positive emotions (not wholly surprising considering the obvious association with smiling) [1].
Other research using fEMG has also shown how the activation of the zygomaticus major is not necessarily exclusively linked to the generation of positive emotions. As Hart and others state in a recent article “especially in more complex environments … smiling activity may be difficult to interpret: smiles can be wry, sarcastic, and smirking as well as expressions of true positive feeling” [2].
Interestingly, there are psychophysiological parameters which broadly define whether or not the smile is seen as genuine or not – spontaneous smiles are often shown for shorter durations (about 4-6 seconds), have a slower offset, and are more symmetrical than deliberate smiles [3]. It’s possible to make this discrimination with the help of fEMG recordings of the zygomaticus major, increasing the validity of relating facial expressions to emotions.
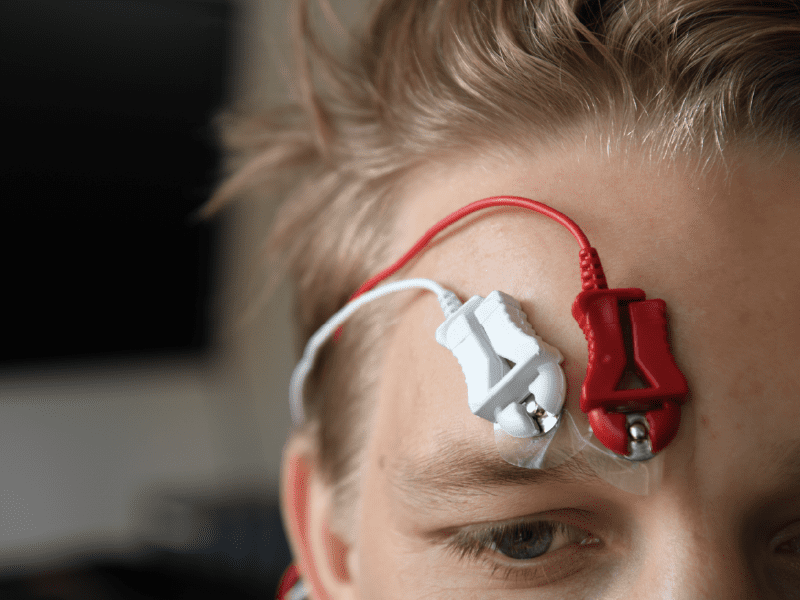
As fEMG is such a sensitive measure, it is able to detect the electrical activity underlying muscle movement even when there is no overt muscle activation (i.e. that someone can be seen to be smiling).
One such study that examined this in more details was by Larsen and other researchers in 2003 [4]. Participants were presented with a range of stimuli that had previously been validated to trigger a range of specific emotions, while fEMG, respiration, and skin conductance (GSR) was recorded. A self-report survey was also administered.
The researchers found that the activity of the zygomaticus major was linearly related to the positive valence of the stimuli – in essence, the more positive the stimulus, the more activity of this smiling muscle. Similarly, a negative relationship was found with corrugator supercilii activity (the frowning muscle), and positive stimuli.
The results suggested that these two facial muscles could potentially work as a proxy for the experience of positive and negative emotional responses. The research also suggests that if the expected emotion has a clear explanation, then the application of fEMG can also provide clear answers.
Automatic facial expression analysis
Another method for exploring activation of the zygomaticus major is through facial expression analysis, in which the movements of the face are visually recorded, and the movements are categorized into discrete muscle movements, often known as action units.
This methodology was originally carried out through manual coding of facial expressions (through the Facial Action Coding System [5], which we’ve covered before), but can now be carried out through software such as Affectiva, which employs machine learning methods to analyze facial muscle movement effectively in real-time.
One recent study compared facial muscle movements using fEMG and Affectiva by asking participants to display a set of emotions while one of the modalities was used [6]. The researchers found that these methods are essentially comparable when it comes to detecting the level of activity of the zygomaticus major, orbicularis orii, and the corrugator supercilii.
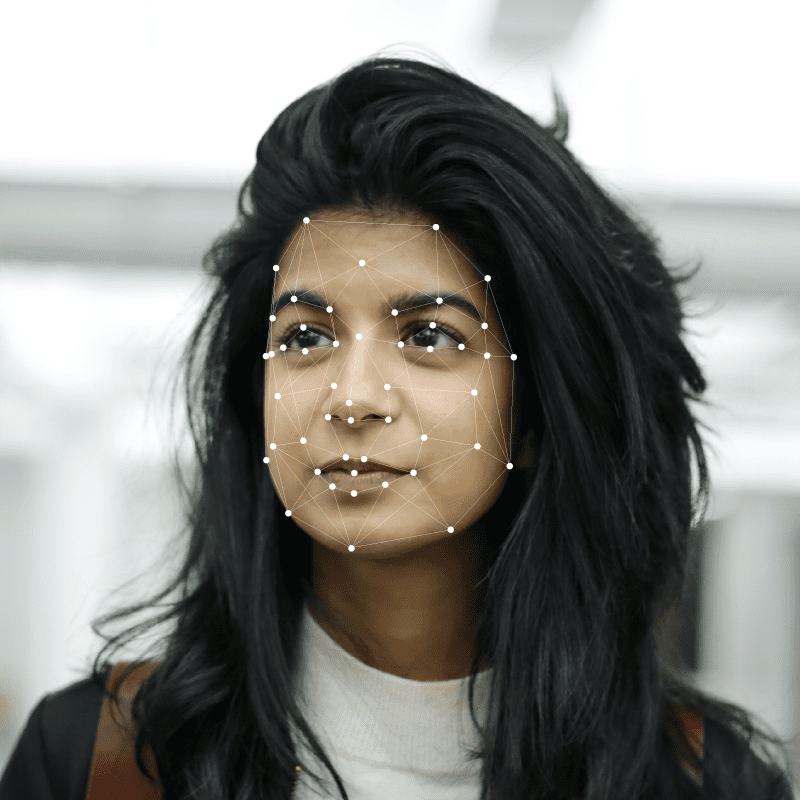
While the advantage of fEMG is its sensitivity, it becomes impractical to record the activity of many muscles at the same time (recordings of 1-3 facial muscles are the norm). Automatic facial expression analysis on the other hand allows the detection of all facial muscle movement, and can even provide a calculation of how these combined movements give rise to complex facial expressions that are associated with discrete emotions (e.g. that a widened mouth and raised eyebrows are associated with surprise).
Another study that showed the potential of automatic facial expression analysis was completed with Affectiva in 2013 [7]. Smiling responses (activation of the zygomaticus major) of over 1500 participants was classified while they watched adverts shown over the internet. The large-scale data collection was made possible due to the non-invasive nature of this methodology, allowing much more data to be collected than most research (particularly when compared to typical fEMG studies).
The researchers found that it was not only possible to classify zygomaticus major activation / smiling responses across a majority of the recordings (despite inevitable problems associated with reduced experimental control – poor lighting, increased participant movement etc), but also that accurate predictions of “liking” and “desire to view again” could be made solely from this data.
Future application of such methodologies opens up the possibility of widespread data collection, and the answering of nuanced questions about emotional preferences, based on activation of the zygomaticus major muscle (and also other associated facial muscle movements).
Despite being a single muscle, a great deal of information can be obtained from activation of the zygomaticus major, and even more when the whole repertoire of facial expressions is taken into account. For research, this is certainly something to smile about.
Free 42-page Facial Expression Analysis Guide
For Beginners and Intermediates
- Get a thorough understanding of all aspects
- Valuable facial expression analysis insights
- Learn how to take your research to the next level
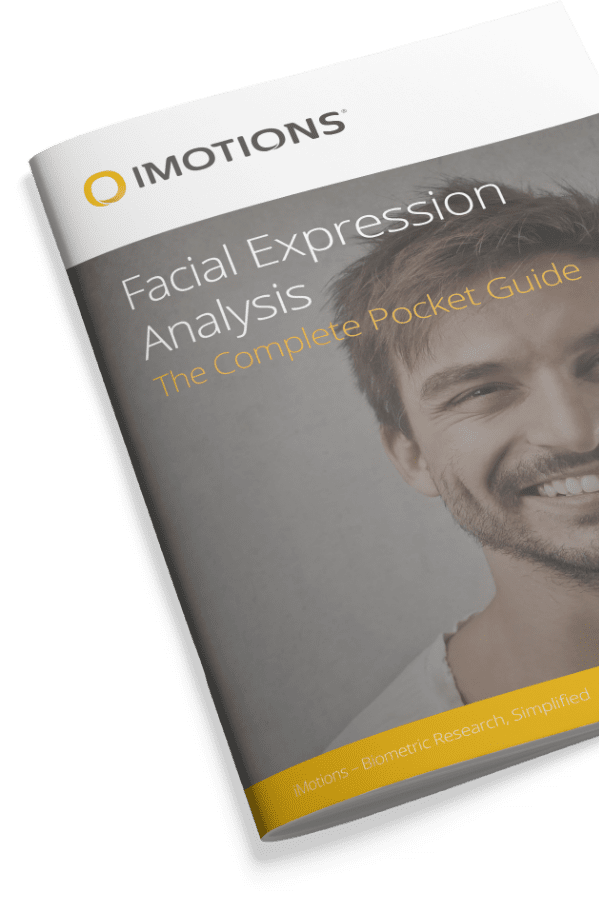
References
[1] Joyal, C., Jacob, L., Cigna, M., Guay, J., & Renaud, P. (2014). Virtual faces expressing emotions: an initial concomitant and construct validity study. Frontiers In Human Neuroscience, 8. doi: 10.3389/fnhum.2014.00787
[2] Björn’t Hart, B., Struiksma, M., van Boxtel, A., & van Berkum, J. (2018). Emotion in Stories: Facial EMG Evidence for Both Mental Simulation and Moral Evaluation. Frontiers In Psychology, 9. doi: 10.3389/fpsyg.2018.00613
[3] Schmidt, K., Ambadar, Z., Cohn, J., & Reed, L. (2006). Movement Differences between Deliberate and Spontaneous Facial Expressions: Zygomaticus Major Action in Smiling. Journal Of Nonverbal Behavior, 30(1), 37-52. doi: 10.1007/s10919-005-0003-x
[4] Larsen, J., Norris, C., & Cacioppo, J. (2003). Effects of positive and negative affect on electromyographic activity over zygomaticus major and corrugator supercilii. Psychophysiology, 40(5), 776-785. doi: 10.1111/1469-8986.00078
[5] Ekman, P., & Rosenberg, E. (2005). What the Face Reveals. New York: Oxford University Press.
[6] Kulke, L., Feyerabend, D., & Schacht, A. (2018). Comparing the Affectiva iMotions Facial Expression Analysis Software with EMG. https://doi.org/10.31234/osf.io/6c58y
[7] McDuff, D., El Kaliouby, R., Senechal, T., Demirdjian, D., & Picard, R. (2014). Automatic measurement of ad preferences from facial responses gathered over the Internet. Image And Vision Computing, 32(10), 630-640. doi: 10.1016/j.imavis.2014.01.004