EMG (electromyography) records the movement of our muscles. It is based on the simple fact that whenever a muscle contracts, a burst of electric activity is generated which propagates through adjacent tissue and bone and can be recorded from neighboring skin areas.
Table of Contents
How do muscles move
The process of course begins in the brain. Triggering muscle movements begins in the motor cortex, where neural activity (a series of action potentials) signals to the spinal cord, and the information about the movement is conveyed to the relevant muscle via motor neurons [1]. This begins with upper motor neurons, that carry the signal to lower motor neurons.
The lower motor neurons are the actual instigators of muscle movement, as they innervate the muscle directly at the neuromuscular junction. This innervation causes the release of Calcium ions within the muscle, ultimately creating a mechanical change in the tension of the muscle [1, 2]. As this process involves depolarization (a change in the electrochemical gradient), the difference in current can be detected by EMG.
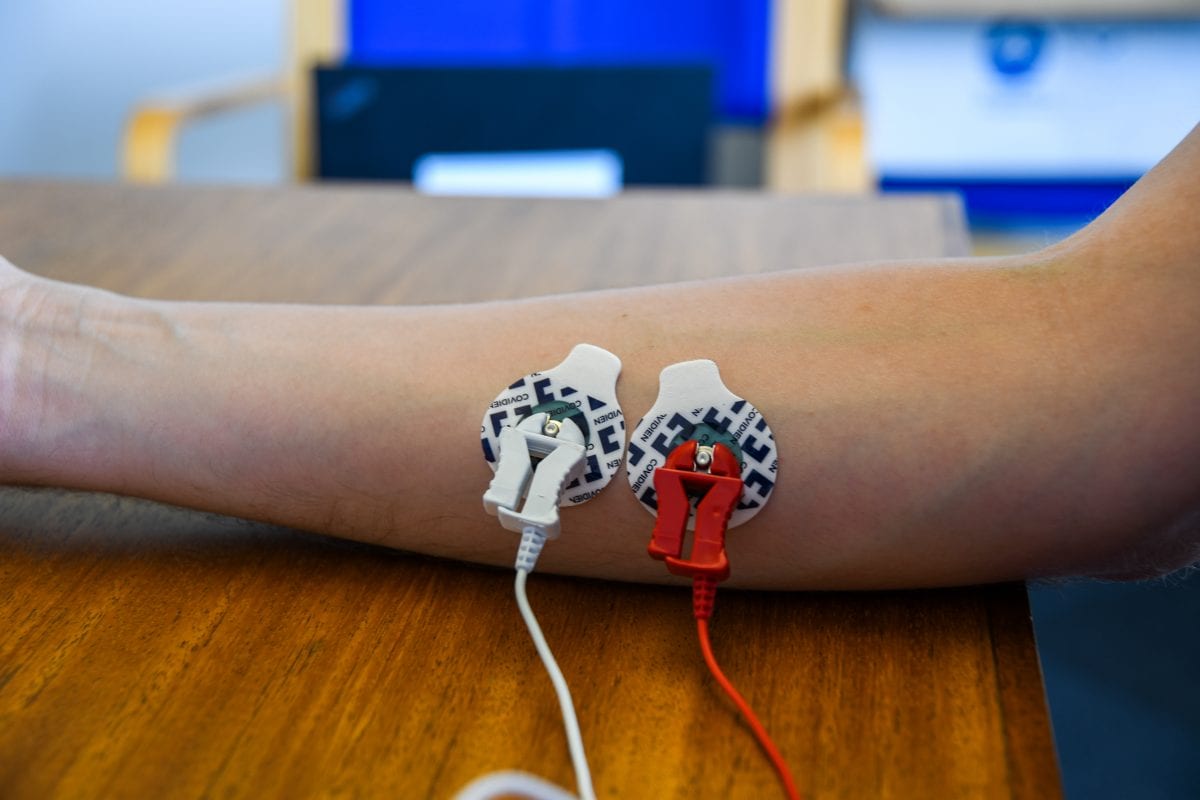
How does EMG work?
As EMG activity (measured in microvolts) is linearly related to the amount of muscle contraction as well as the number of contracted muscles – or in other words, the stronger the muscle contraction and the higher the number of activated muscles, the higher the recorded voltage amplitude will be.
As EMG activity is even measurable when we do not display obvious actions or even inhibit certain behaviors, EMG recordings represent an additional source of information into cognitive-behavioral processing which would be hidden based on pure observation.
Previous research indicates a close coupling between muscular EMG and motor cortex EEG as reflected by significant correlations in signal features such as frequency power and phase in the (12 – 25 Hz) beta band [3, 4, 5]. This emphasizes the power of EMG recordings for monitoring the interaction of cortical and motor systems.
While EMG is clearly helpful in understanding how people move, the use of fEMG (facial electromyography, in which EMG signals are recorded from the muscles of the face), can also provide information about facial expressions.
What is Facial electromyography (fEMG)
The particular strength of fEMG as compared to automated or manual facial expression analysis (that is based on the analysis of video recordings), is the sensitivity it has in detecting signals. While more difficult to set up than video recordings, the data it provides is more robust. It can even detect the non-visible muscular activity of the face, providing information about suppressed expressions, or those that otherwise do not pass the threshold for visible activity.
Check out: Automated Facial Coding vs. fEMG – Which Tool is Best for Your Research?
This sensitivity is instrumental in understanding the hidden (intentionally or not) facial expressions that can be linked to an internal emotional state [6, 7], allowing a window to see how someone is implicitly feeling.
This process can be made even more powerful if complemented with other measures of human behavior, such as eye tracking or GSR (galvanic skin response), allowing you to see where someone looks, and their level of emotional arousal, as well as the direction of those emotions.
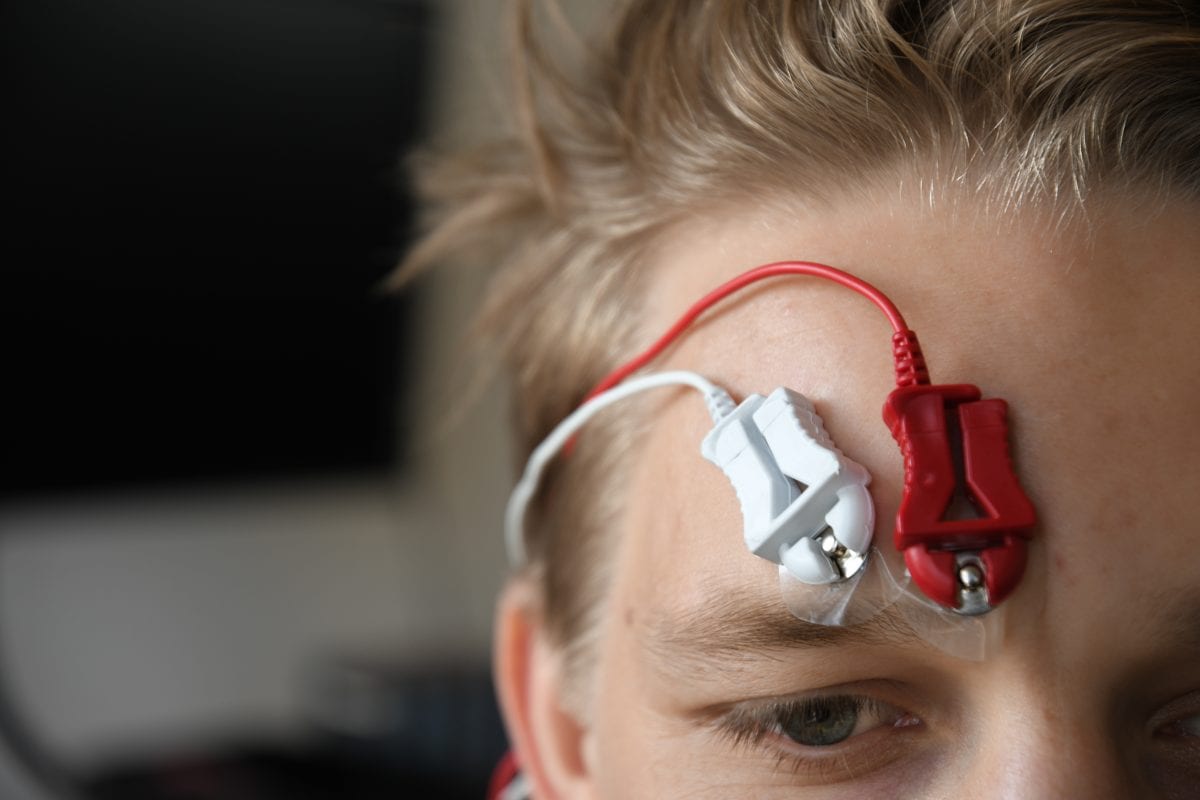
Check out our Webinars for all things biosensors
How to get started with EMG sensors
- Use surface electrodes
- Surface EMG is a completely non-invasive technology that allows you to easily place EMG electrodes with stickers to the skin.
- As these electrodes are non-invasive, EMG is an ideal method for monitoring physiological processes without interfering with established routines and movement patterns.
- In order to obtain high-quality data, keep in mind to always clean the recording sites and remove makeup using alcohol rubs.
- Place EMG electrodes over muscle groups of interest
- Admittedly, this requires a certain level of anatomic knowledge. Only if you know the muscle regimes involved in a specific action you will be able to get valid and reliable signals.
- Facial EMG recordings, for example, are complicated by the fact that there are 43 muscles in the face. Most of these are controlled by the seventh cranial nerve (the “facial nerve”), which routes from the cerebral cortex to five primary branches (temporal, zygomatic, buccal, mandibular and cervical).
- Each branch innervates muscles in different face areas, allowing for intricate facial twists and contortions.
- Select an appropriate reference site
- EMG data is collected as the voltage difference between recording site and reference site, therefore selecting an appropriate reference site is as important as the actual recording site.
- We recommend placing EMG reference channels at bony body parts such as an elbow, hip or collar bones.
- Use short electrode cables/leads
- In order to minimize the amount of electrical noise picked up from surrounding power sources, keep the length of the cables that connect the recording electrodes with the amplifier/recording device as short as possible.
For more information on how to set up the perfect experiment, download our comprehensive guide below.
EMG
The Complete Pocket Guide
- 32 pages of comprehensive material on electromyography
- Valuable electromyography research insights (with examples)
- Learn how to take your research to the next level
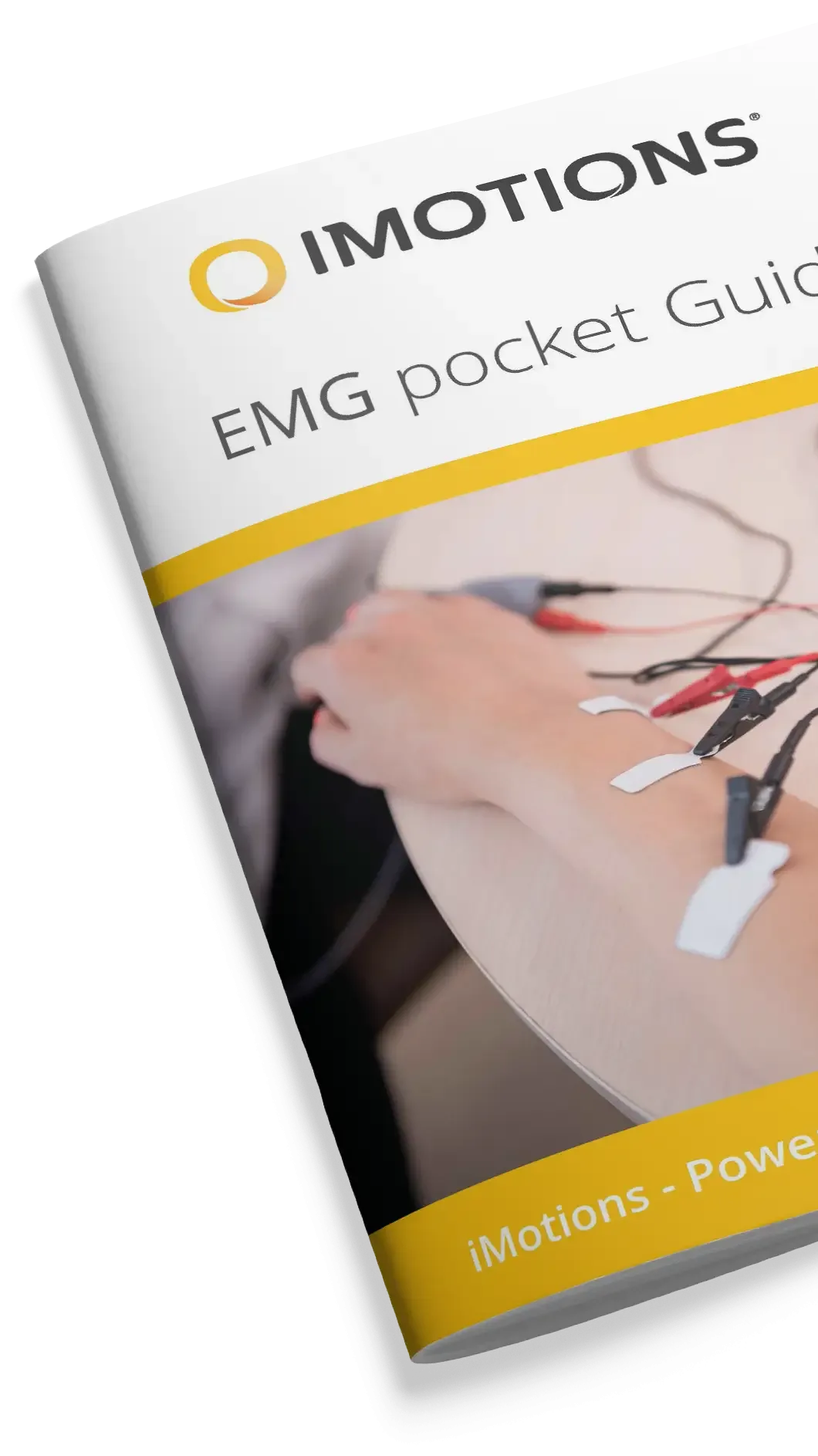
References
[1] Winter DA. (1984). Biomechanics of human movement with applications to the study of human locomotion. Crit Rev Biomed Eng. 9:287–314.
[2] Picard N, Strick PL. (1996) Motor areas of the medial wall: a review of their location and functional activation. Cereb. Cortex. 6:342–353. doi: 10.1093/cercor/6.3.342.
[3] Hashimoto Y., Ushiba J., Kimura A., Liu M. & Tomita Y. (2010). Correlation between EEG-EMG coherence during isometric contraction and its imaginary execution. Acta Neurobiol. Exp. 70, 76–85.
[4] Churchland MM, Cunningham JP, Kaufman MT, Ryu SI, Shenoy KV. (2010). Cortical preparatory activity: representation of movement or first cog in a dynamical machine? Neuron 68: 387–400.
[5] Churchland MM, Santhanam G, Shenoy KV. (2006). Preparatory activity in premotor and motor cortex reflects the speed of the upcoming reach. J Neurophysiol 96: 3130–3146.
[6] Y. G. Yang, and S. Yang, (2011). Study of emotion recognition based on surface electromyography and improved least squares support vector machine, Journal of Computers, vol. 6, no. 8, pp. 1707-1714.
[7] Tan, J.W., Walter, S., Scheck, A., Hrabal, D., Hoffmann, H., Kessler, H. & Traue, H. (2011). Facial electromyography (femg) activities in response to affective visual stimulation. In Affective Computational Intelligence (WACI), 2011 IEEE Workshop on, 1 –5.[/fusion_builder_column][/fusion_builder_row][/fusion_builder_container]