It’s an age-old concept in science that if you want to understand something, you first have to measure it. Attempts to understand human behavior can involve the measurement of a range of physiological signals, but getting closer to the brain requires equipment to record its activity.
There are several neuroimaging devices available, but none of them give such a high level of temporal resolution as EEG. While the level of visual information received isn’t as detailed as some other neuroimaging techniques (such as fMRI, or functional magnetic resonance imaging), the temporal resolution, combined with the portability, relative affordability, and ease-of-use means that EEG is one of – if not the most – commonly used neuroimaging methods.
EEG is often used within strictly controlled experimental settings – scenarios in which data is collected for a set amount of time, with little movement, and clearly defined stimuli. While this has been ideal for assessing brain activity in specific settings, sometimes a different approach is required.
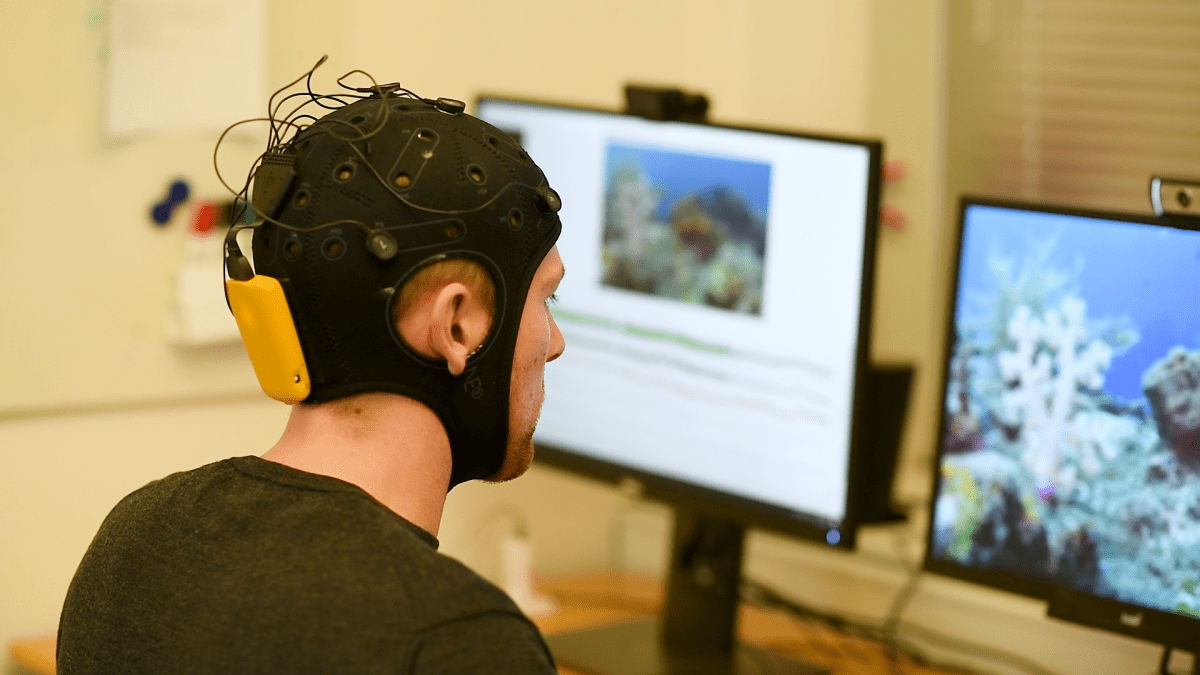
Ambulatory EEG is the method of collecting EEG data over a long period of time (this can be anything from hours to days) in naturalistic settings. This means not only that there will be more data, but also more movement, and (likely) a wide range of interacting factors will impact the data.
What are the reasons for ambulatory EEG?
Ambulatory EEG is usually carried out for the following reasons:
- Epilepsy detection and monitoring
- Seizure investigations
- Sleep studies
- Research of natural behavior (e.g. shopper studies, user interaction studies)
The former two examples are principally for medical reasons – the application of ambulatory methods is done so in order to detect or measure aberrant neurological activity throughout the day. The latter two are more concerned with understanding how brain processes change in a normal population over time (and potentially within different contexts as well).
Below, we will specifically talk about how the brain processes occurring with natural behavior can be measured using ambulatory EEG, and the common pitfalls that occur with data collection and analysis.
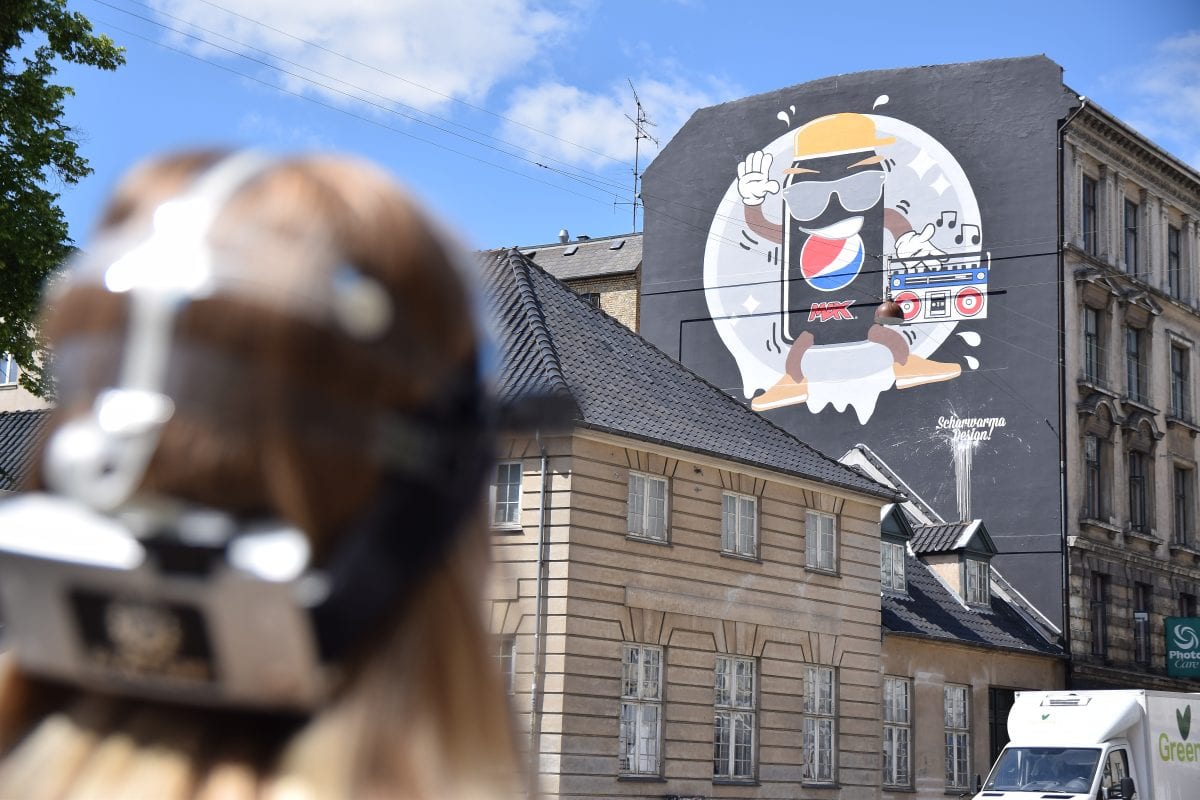
How does it work?
In many cases, ambulatory EEG is essentially the same to the usual setup of a regular EEG experiment – a participant has an EEG headset placed on the head, the electrodes make contact to the skin using some kind of conductive gel, the conductance is tested, and the whole setup is checked in the connected software.
Although there are crucial similarities, there are also crucial differences. While some EEG setups require equipment that is impractical (or impossible) to easily move around with, ambulatory EEG necessitates devices that provide ease-of-movement and portability. Any participant that is wearing an EEG headset for a length of time will need to be able to move around unobstructed, and to feel comfortable doing so.
Further considerations should be made for the data – not only where it’s going to go (collecting massive amounts of data of course requires that it’s stored efficiently), but also how the data is collected. Such contexts often require that the participant will move around, introducing noise and artifacts to the signals.
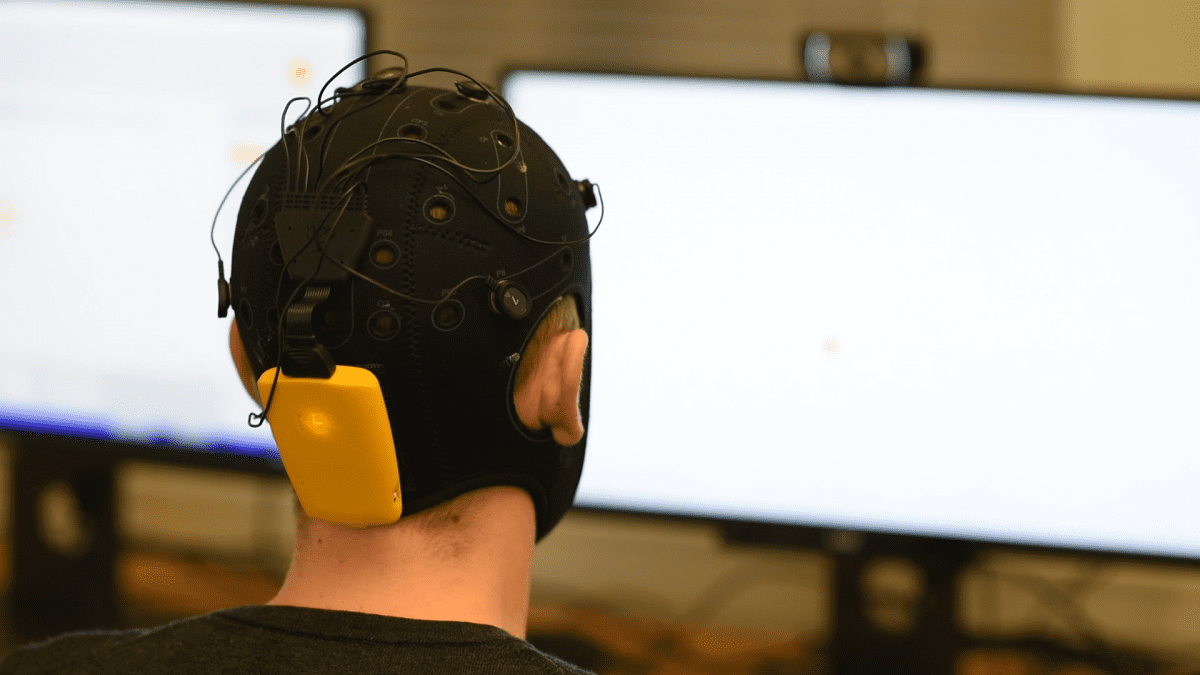
The signal and the noise
Noise will always be present in essentially any measurement, regardless of whether it’s EEG data collection, or anything else (although EEG is particularly sensitive to the presence of noise). The amount of noise might be miniscule, but a certain level of noise is always expected. Mitigating (and potentially filtering) the signal from the noise is therefore a critical component of any data analysis.
This is even more pronounced in ambulatory EEG measurements, in which the participant might be walking around or moving in other ways that can interfere with the position of the electrodes on the head. As you can see in the image of an EEG recording below, this can have a severe impact on the data quality.
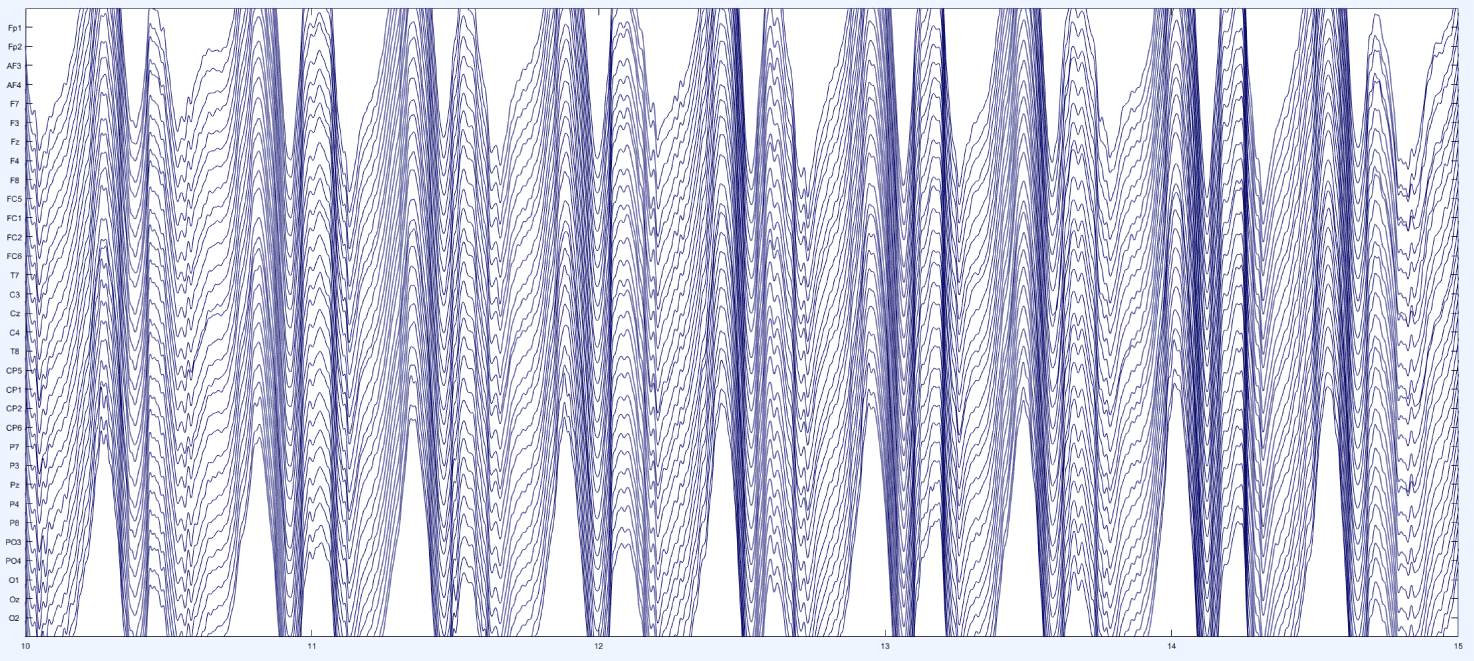
This is not to say that data collection shouldn’t happen in scenarios in which people are required to walk around, but just that a new strategy is required.
There are two main ways to mitigate the problem that this presents. The first lies within experimental design, the other within data analysis.
Experimental design for ambulatory EEG
To get around the problem of noisy data, often the best approach is to reserve ‘artifact-free’ time-windows in the recording. For instance, a common scenario for ambulatory EEG would be to record EEG data from a participant while they move around and interact with items (think of a study of shopping behavior in a supermarket, or a developmental study with children). While there may be a great deal of movement at certain points in the recording, the analysis focuses on the moments which are free of artifacts.
Experimental design can therefore ensure that there are moments of little activity from which to record from – when a participant stops to look at a supermarket shelf for example, or when a child is (finally!) still.
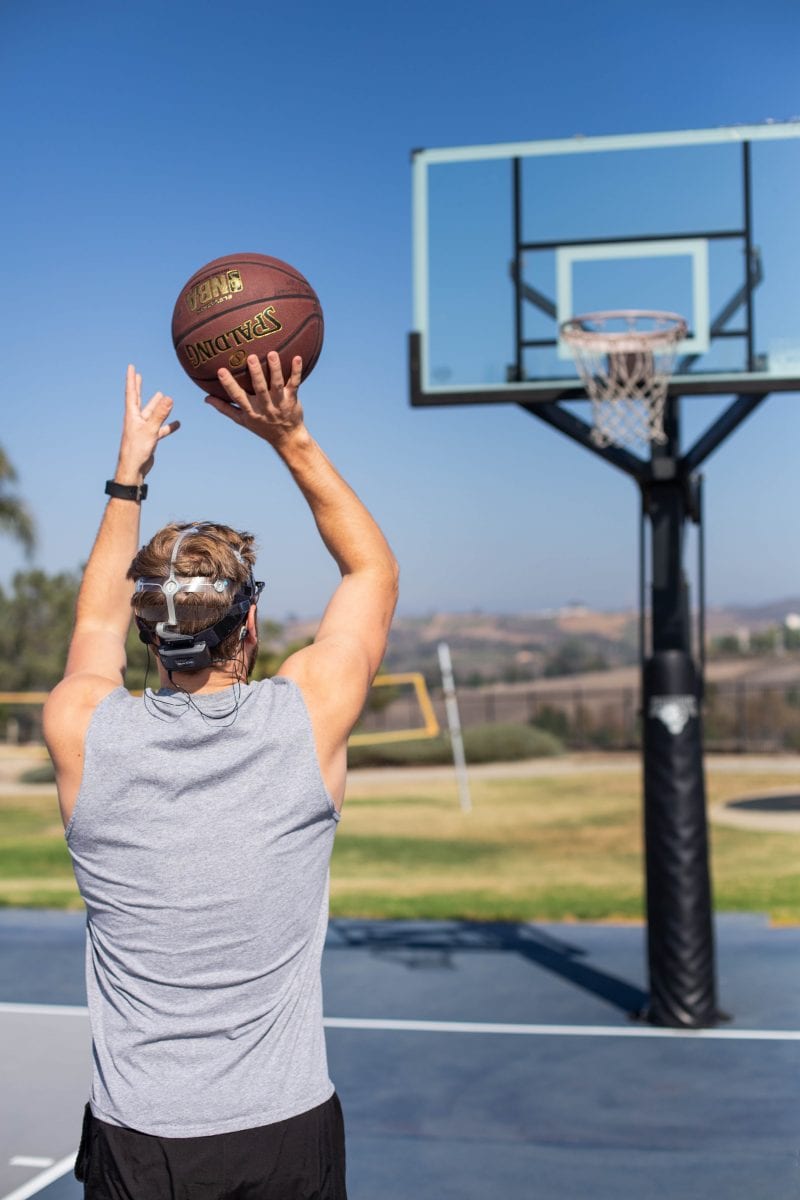
This also raises the question of which EEG hardware is best suited to ambulatory settings. Neuroelectrics headsets have the advantage of being fitted with a headcap, somewhat restricting the amount of movement to the electrodes. They can also be fitted with a variety of electrode types which can help in the speed of application or signal quality.
ABM (Advanced Brain Monitoring) headsets can also be worn portably and data collected through a Bluetooth connection, although are not configured within a cap.
Repeated measures, in which the participants are exposed to the same stimulus, and different sections of the data can be collected each time, can be used to generate a complete picture of the neural responses throughout the entire stimulus exposure duration.
This means, for example, that the EEG data for the first 10 seconds of the first stimulus exposure could be complemented with the subsequent 10 seconds from the second stimulus exposure. If averaged over enough trials and participants, a general view of participant responses can be reliably constructed.
This also applies to within-participant experiment designs – it’s more valid to compare like-to-like in experiments. This means in essence that comparing the reactions of participants to stimulus A with their reactions to stimulus B is more valid than comparing the reactions of two different groups of participants.
All of these approaches however also require that efforts are made in another area of the study – in the analysis.
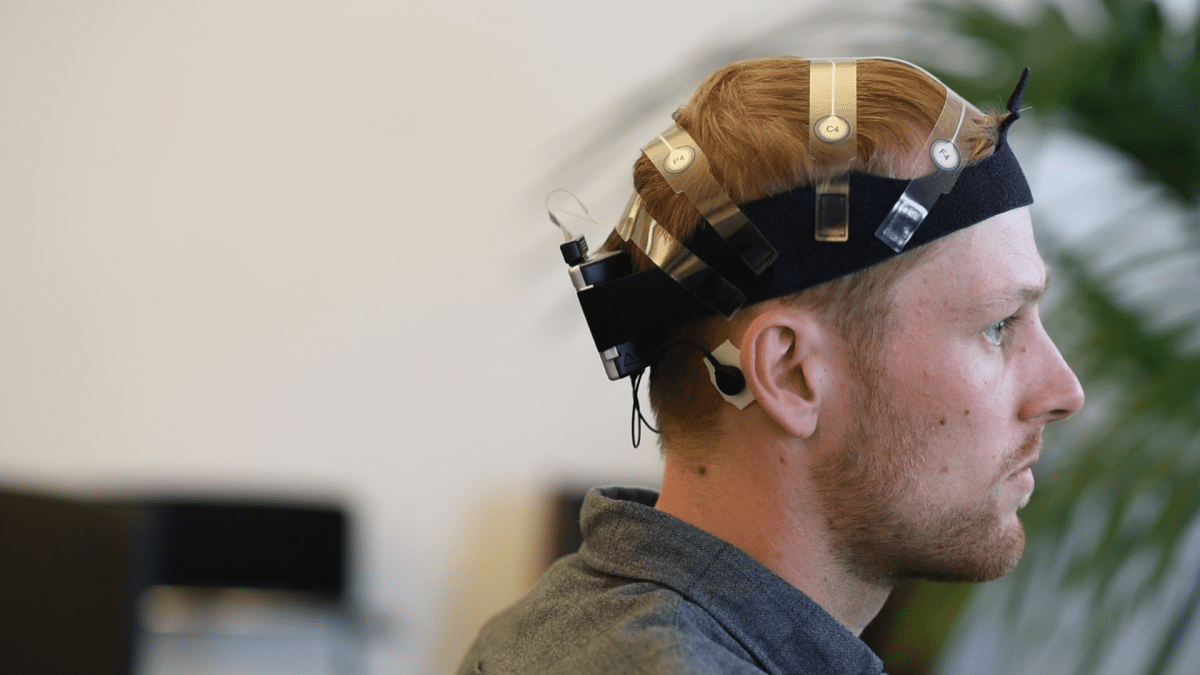
Analysis methods for ambulatory EEG
It’s clear that collecting EEG data from a dynamically changing environment over a period of time presents a challenge. Noise from a range of sources is easily introduced.
The first step in data analysis is often visual inspection – it could be that the only data that is impacted by noise is present in moments of the experiment (or electrodes) that aren’t being investigated (e.g. when the child isn’t paying attention to the stimuli / when the person is walking between shelves). These noisy parts of the data are simply removed.
If noise persists in the important parts of the data, the next step is to apply filtering. Filtering is particularly effective if noise is present in very low (below 1 Hz) or very high (above 50 Hz) frequencies – frequencies which are not usually of interest to the study.
In that case, a high-pass filter (suppressing artefacts below a cutoff) or low-pass filter (suppressing artefacts above a cutoff) can be applied to the data. For instance, if the research question concerns alpha activity (which is activity in the frequency range from 8 to12 Hz), high frequency noise can often safely be removed using a low-pass filter.
Are artifacts being taken care of within iMotions? Within iMotions, it is important to distinguish between working with ‘raw data’ or with ‘metrics’. Users who use iMotions as a recording tool, export the ‘raw data’ for further processing in specialized EEG software, such as EEGLAB, Fieldtrip, BCILAB, BESA or Cartool.
As such, the user is in full control of (and with full responsibility for) the signal processing applied to the data. Other users work with ‘metrics’, which are either provided by the hardware (for ABM or Emotiv) or by iMotions (e.g., ‘frontal asymmetry’). In that case, decontamination of the signal is already taken care of in the calculation of the metrics.
Conclusion
Ambulatory EEG offers the promise of understanding the action of the brain in realistic, dynamic environments. It also comes at the price of (often) reduced data quality. This is unfortunately not entirely avoidable, yet with the right experimental approach, study setup, and data handling, the effects can be mitigated. This means that naturalistic research can be completed.
While these methods above can help in dealing with the data collected from ambulatory EEG experiments, the single most important and beneficial aspect of any experiment (ambulatory or not) relies in piloting the approach before rolling it out to more participants. Testing the test is crucial for successful research, as it allows you to understand what does and doesn’t work.
I hope you’ve enjoyed reading about what ambulatory EEG is, and how it works. If you’d like a deeper walkthrough of EEG, then download our free guide below.
Free 59-page EEG Guide
For Beginners and Intermediates
- Get a thorough understanding of the essentials
- Valuable EEG research insight
- Learn how to take your research to the next level
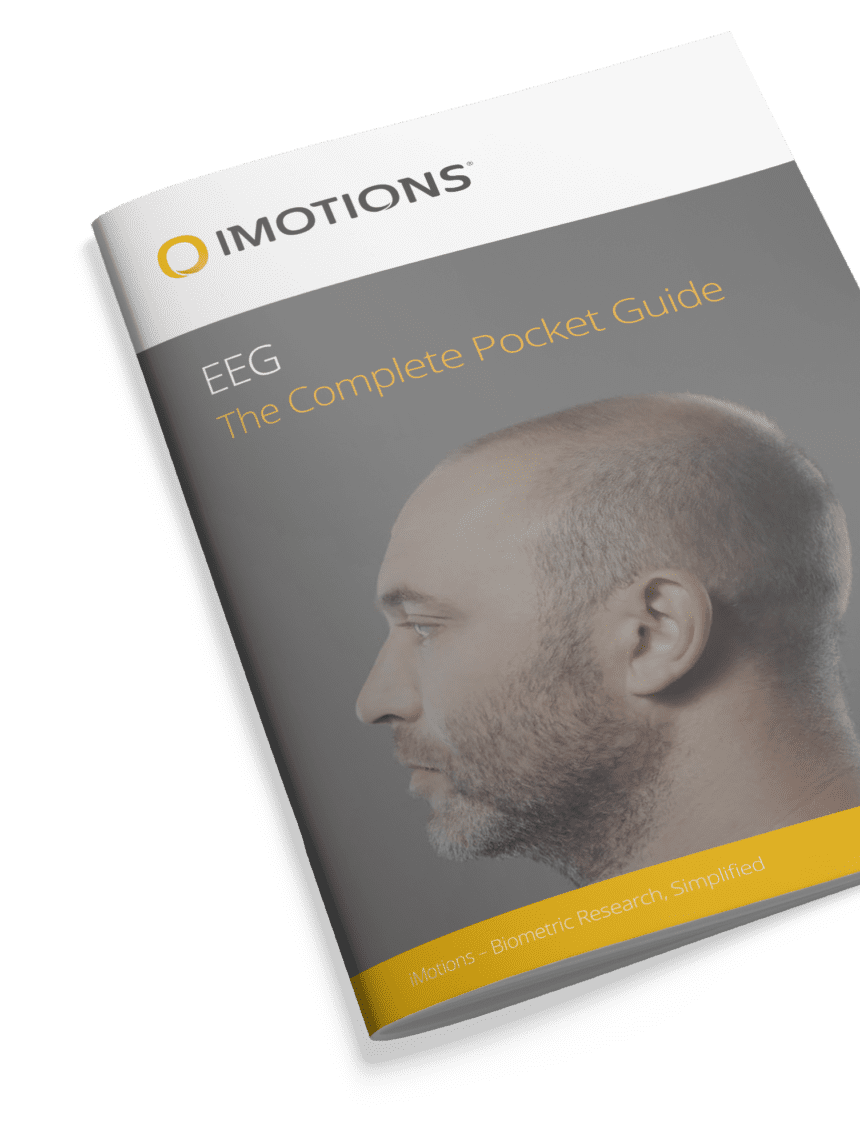