Traffic accidents are a leading cause of death worldwide. According to the WHO’s 2018 Report on global road safety, 1.35 million deaths were caused by traffic accidents in one year. Human driving errors due to attention loss and cognitive load are the biggest reasons for the high prevalence of traffic accidents (WHO, 2018).
Improving drivers’ performance by better understanding the factors that lead to cognitive overload, can drastically reduce the fatalities caused by car crashes. Autonomous vehicles (AVs) are another promising way to reduce the number of crashes by reducing human driving errors and driver misconduct. As AVs come to the market, the interaction of human drivers with these vehicles will present a new scene for error, since human drivers may not be sure how an autonomous vehicle will behave in urban driving scenarios (Shariff, Bonnefon & Rahwan, 2017).
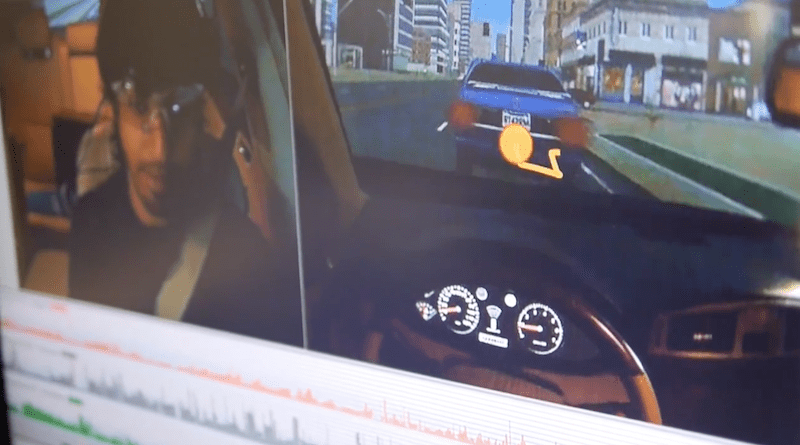
A driverless car to a human may elicit anxiety and fear during driving, and in turn increase the rate of accidents (Acheampong & Cugurullo, 2019; Brown et al., 2018). Therefore it is crucial to study human attention during driving and human-machine interaction in the transition phase from non-autonomous cars to fully self-driving cars.
Biosensor research is a common method applied in this field in simulations that allow the creation of new safety and control systems for our vehicles, creating new tools that help to reduce future traffic accidents. Below are three interesting studies in this field, which have used biosensor technologies to carry out their projects, obtaining very interesting results that may be applied in the future to improve AV products in the automotive industry.
Biosensors can Quantify ‘Takeover Performance’ in Real-Time Automated Driving Simulations
It seems that new autonomous cars will not be fully automated anytime soon, most likely human drivers will still need to be alert to take over an automated car when necessary. According to the Society of Automotive Engineers’ classification, the automation level of a vehicle ranges from L0 (no automation) to L5 (fully automatic). The highest degree AV to come to the market soonest is expected to be at the L3 level of automation. At L3 level, the human driver is not actively controlling the car, but still needs to be alert to take over the control if required.
The human driver is expected to take over from automation when there are critical urban driving situations, such as when there is an unexpected construction zone, or a police car approaching from the left lane. These scenarios are challenging from a cognitive psychology perspective because they require the executive capacity of task-switching, the ability to effectively switch between two demanding cognitive tasks.
To tackle this problem, it is crucial to know what the cognitive limits are for human drivers so that we can ensure safe performance in autonomous vehicles. Researchers from University of Michigan (Du et al., 2020) tackled this issue by studying driver performance in a simulated autonomous driving session. The aim of the researchers was to use physiological data from human drivers to computationally predict costs on the task switching performance when there is a need to take over the vehicle. The study used eye-tracking, galvanic skin response, and heart rate to measure cognitive and bodily states. The wearable sensors were placed in a driving simulator, data was streamed into iMotions real-time and synchronized.
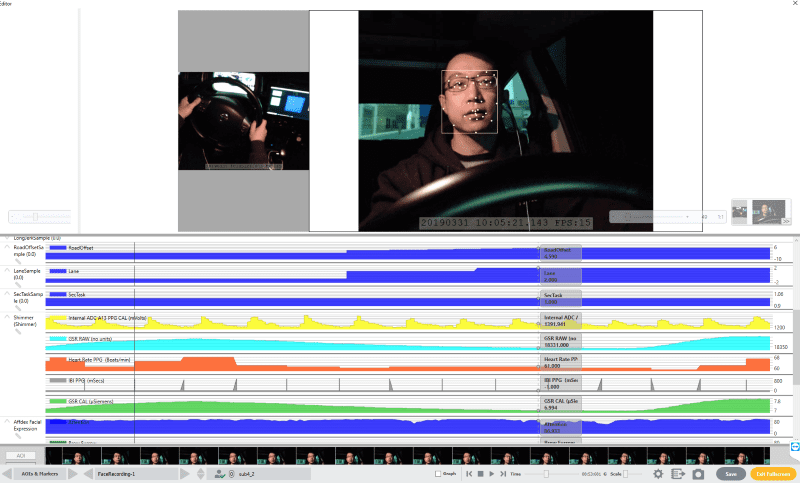
The participants had to unexpectedly take over in different urban driving scenarios, while doing a visual memory task at other times. While the computational model to predict drivers’ performance is quite complex, the researchers used metrics such as AOI’s to see when the drivers’ eyes were on the road, scan patterns, number of blinks, GSR peak times to calculate driving performance. Impressively, the researchers had over 70% accuracy to predict drivers’ takeover performance using their physiological data.
Biosensors Enable Studying Driver Safety in a Virtual Driving Zone with Autonomous Vehicles
Accessibility of VR headsets make it possible to study more complex human-machine interaction scenarios when human drivers confront self-driving vehicles. Human factors researchers from University of Virginia studied human drivers’ performance, measuring their arousal and heart rate when driving with an autonomous car on the same road in a simulation.
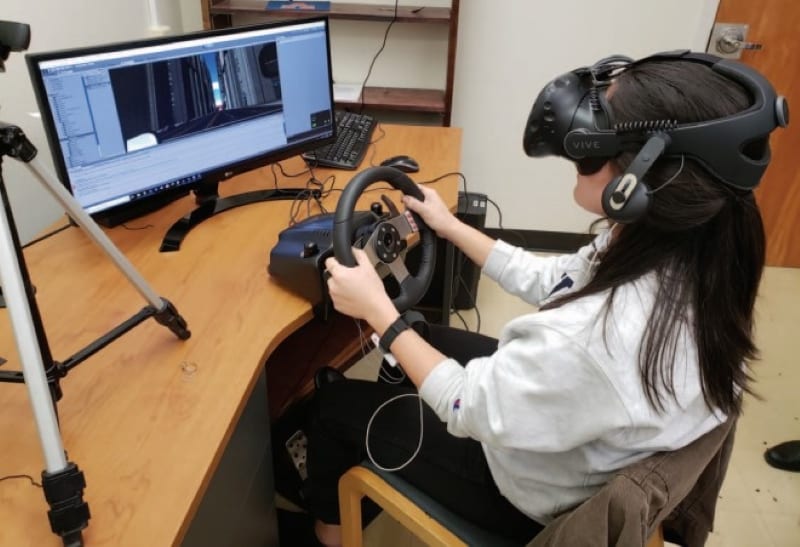
The participants were put on a Shimmer GSR and Optical Pulse ear-clip heart rate device while wearing the Unity HTC Vive headset in a metropolitan driving simulator as all measurements were synchronized in iMotions. GSR and heart rate data were used to quantify stress and nervousness of drivers. Participants needed to follow an autonomous car in front of them, and avoid collision at various zones. The study ended with a questionnaire on trust and acceptance towards self-driving cars. The researchers were interested in the drivers’ physiological data, trust and acceptance factors that were associated with the driving behavior and collision rate.
One of the main findings was that the following distance is an important variable to categorize drivers and predict their behavior. It was found that participants who showed more trust and acceptance to AVs preferred a shorter following distance when driving behind one whereas drivers who were hesitant towards AVs preferred a much higher following distance. In addition, it was observed that drivers’ caution level changes if they collide, significantly increasing the following distance afterward. Another finding showed that GSR peaks increase in dilemma situations (e.g. yellow light), showing nervousness and arousal in these conditions.
The researchers conclude that biosensors and VR simulation are reliable ways to study driver behavior, and recommend the authorities to use VR as a way to acclimate to using autonomous vehicles. The findings emphasize that human factors should inform the developers of autonomous vehicles to consider the appropriate following distances and decision-making algorithms based on their findings.
Understanding the Driving experience with Biosensors
A great example is from our client Mazda Motor Europe, who along with researchers from the University of Fribourg and 60 lucky participants, ventured into the cold, and used facial expression analysis and galvanic skin response to investigate driver engagement on a more… challenging track than usual.
Quite an extensive research setup, not only in terms of respondent count, design of experiment, and general ambitions, but also by the marketing video below they produced along the way.
Attention Modulation with Music to Improve Driving Performance
Impact of music on mood and attention is widely studied by psychologists. The positive impact of music on drivers’ focus was already demonstrated before (Van der Zwaag et al., 2012). Avila-Vazquez and colleagues’ (2017) study aimed to manipulate this knowledge in a real-world driving scenario, using biometric tools to measure the music’s impact and come up with audio recommendations as a way to maintain drivers’ focus.
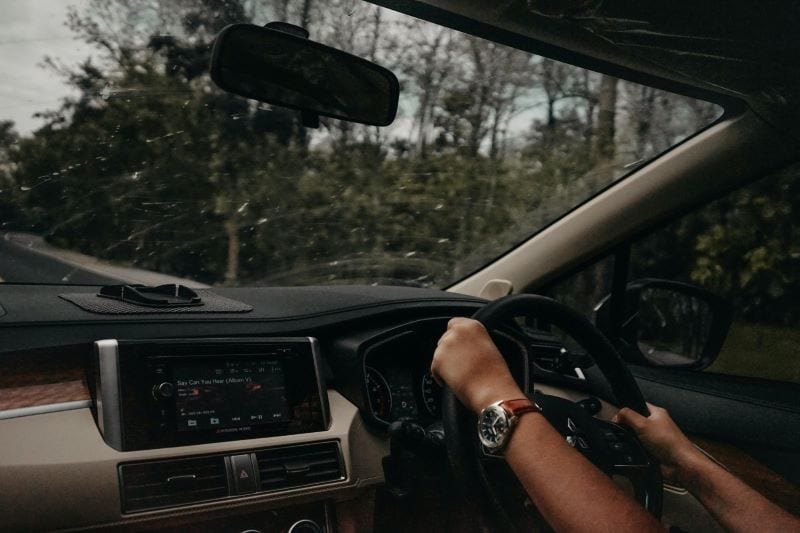
In the study, participants drove in a car in a city while their faces were being recorded with a webcam for facial expression analysis (FEA). The computer webcam was used to gather FEA data and simultaneously playing different kinds of music, and synchronizing the facial expressions data in iMotions. This way, facial expressions were able to indicate when the drivers were losing focus and alertness and the correlation with the type of music being played. The researchers suggested that their approach can be used to personalize music based on an individual’s cognitive profile. For instance, face recognition systems can be implemented in cars to read the drivers’ responses and give feedback to an audio system to play the right music for the individual. The use of music as an element to provide comfort and assistance to the driver.
Learn more about iMotions Platform
These studies show that physiological sensor data can be used to gain important insights into the safest and most effective practices while driving and interacting with vehicles. These insights can be used to improve both automotive product development and driver performance.
Download iMotions
VR Eye Tracking Brochure
iMotions is the world’s leading biosensor platform. Learn more about how VR Eye Tracking can help you with your human behavior research.
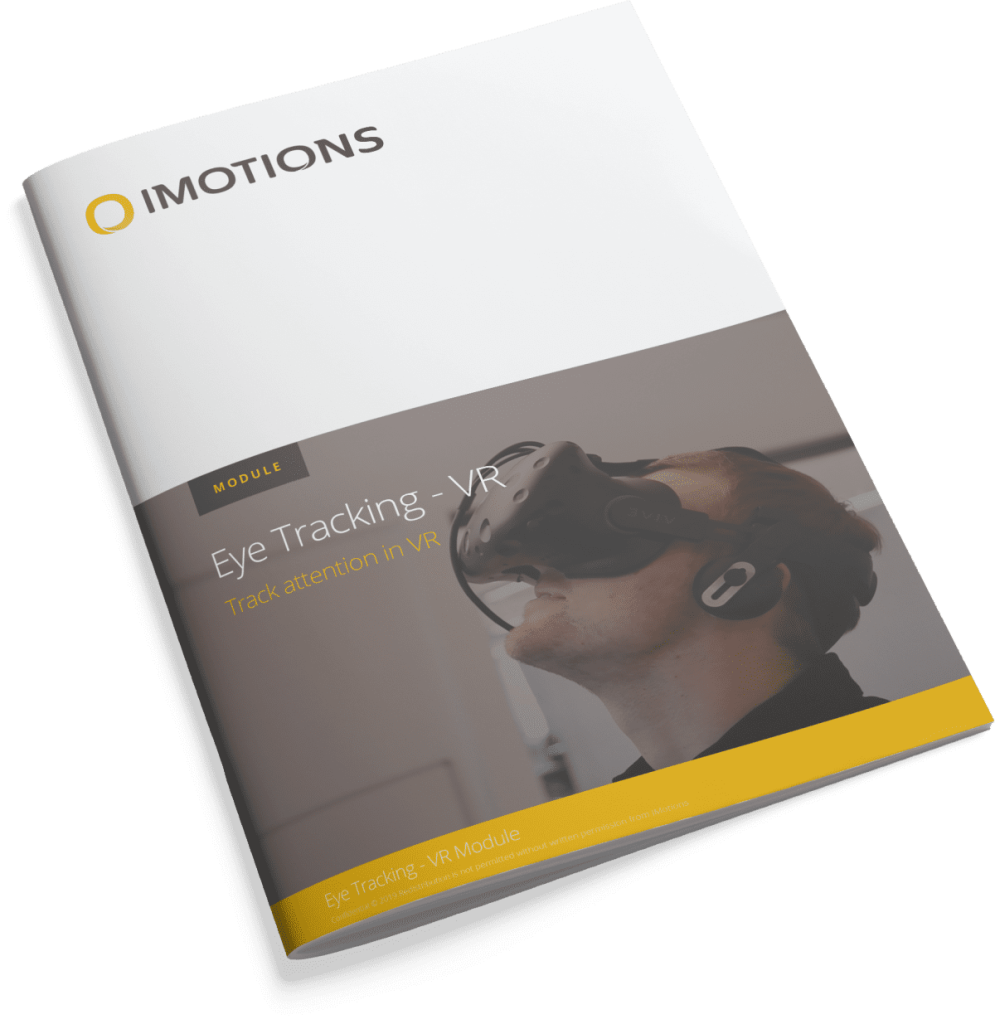
References
Acheampong, R. A., & Cugurullo, F. (2019). Capturing the behavioural determinants behind the adoption of autonomous vehicles: Conceptual frameworks and measurement models to predict public transport, sharing and ownership trends of self-driving cars. Transportation research part F: traffic psychology and behaviour, 62, 349-375.
Avila-Vázquez, R., Navarro-Tuch, S., Bustamante-Bello, R., Mendoza, R. A. R., & Izquierdo-Reyes, J. (2017). Music recommendation system for human attention modulation by facial recognition on a driving task: a proof of concept. In MATEC Web of Conferences (Vol. 124, p. 04013). EDP Sciences.
Brown, B., Park, D., Sheehan, B., Shikoff, S., Solomon, J., Yang, J., & Kim, I. (2018). Assessment of human driver safety at Dilemma Zones with automated vehicles through a virtual reality environment. Systems And Information Engineering Design Symposium (SIEDS).
Du, N., Zhou, F., Pulver, E., Tilbury, D., Robert, L., Pradhan, A., & Yang, X. J. (2020). Predicting Takeover Performance in Conditionally Automated Driving.
Izquierdo-Reyes, J., Ramirez-Mendoza, R. A., Bustamante-Bello, M. R., Navarro-Tuch, S., & Avila-Vazquez, R. (2018). Advanced driver monitoring for assistance system (ADMAS). International Journal on Interactive Design and Manufacturing (IJIDeM), 12(1), 187-197.
Shariff, A., Bonnefon, J. F., & Rahwan, I. (2017). Psychological roadblocks to the adoption of self-driving vehicles. Nature Human Behaviour, 1(10), 694-696.
World Health Organization. (2018). Global status report on road safety 2018. World Health Organization.
Van Der Zwaag, M. D., Dijksterhuis, C., De Waard, D., Mulder, B. L., Westerink, J. H., & Brookhuis, K. A. (2012). The influence of music on mood and performance while driving. Ergonomics, 55(1), 12-22.