What is fatigue detection? In 1965, a man by the name of Dr. Dement rented a convertible in California and drove a 17-year-old boy around for a science experiment. This isn’t the starting scene of a horror movie, but part of the world record attempt for sleep deprivation that was pursued by the 17-year-old Randy Gardner. His science fair experiment ultimately succeeded, and he stayed awake for the planned 264 hours – 11 continuous days.
This is an extreme example of fatigue, and something only very few people come close to experiencing. But we all experience sleep deprivation at some point in our lives, and we don’t have the cover of participating in a scientific experiment to save us from the demands of life – work, family, and everything in between.
While sleep deprivation, and fatigue more generally, are commonly experienced, the effects of this exhaustion can be severe. Up to 274,000 workplace accidents and 6,000 fatalities from car crashes have been associated with sleeplessness – just in the USA alone [2-5].
While the source of sleeplessness within these instances likely largely results from societal and cultural demands, there are other circumstances in which a lack of sleep is part of the norm. Soldiers at war, truck drivers, and medical staff – to name just a few – all share the same demands for sleeplessness as part of the job.
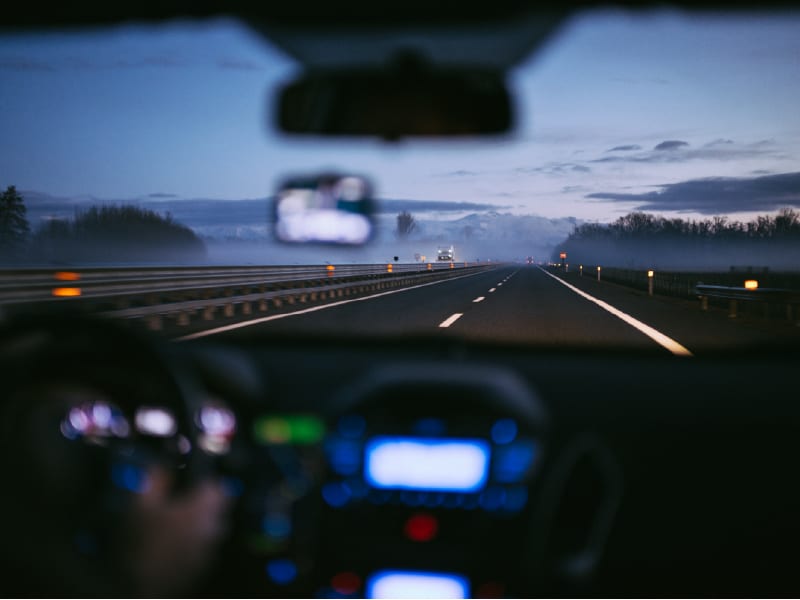
This can inevitably have dire consequences – each of these professions carries an increased risk of accidents if things don’t go according to plan. Understanding how fatigue affects people, and being able to predict it could dramatically reduce the incidence of accidents, improve health, and allow people to perform optimally for as long as possible. How do we do it?
Fatigue Detection Explained
Before we get to the details of detecting fatigue, it’s good to define what fatigue actually is. We might think that we know when we’re tired and that this is therefore easy to define – but this isn’t necessarily the case. Self-reports in general are notoriously unreliable, and self-reports of fatigue are no different [6-7].
Fatigue can be studied and defined in many different ways – there are differences between the fatigue induced by psychiatric disorders (such as depression), somatic disorders (such as Parkinson’s disease), or “consumption-oriented behaviors” (such as alcoholism) [8]. But when discussing accident rates caused by fatigue, it can be defined as a decrease in cognitive performance that can be attributed to sustained cognitive load, and/or prolonged periods of wakefulness.
Due to the inherent inaccuracies in self-reports of fatigue, asking someone how tired they are is unreliable – we are inaccurate reporters. Self-reports would also clearly be an inefficient system for detecting fatigue. We need signals that can be passively and non-invasively detected from the body that can reliably relay information about how fatigued someone truly is. Fortunately, there are several options to do this.
How To Detect Fatigue
Fatigue is a common contributor to driving related accidents – around 328,000 annual car crashes are attributed to tiredness at the wheel in the USA [3]. The setup of someone sitting in a car is also convenient scenario from which to track levels of fatigue. Recent research and innovations have made several advances in this area in recent years.
One of the most widely employed methods for fatigue detection is eye tracking (e.g. [9-12]), in which the gaze location, amount of gaze dispersion, and even eye blinks are used as indicators of tiredness. Researchers in 2018 used driving simulators coupled with eye trackers to assess eye movements during naturalistic driving behavior, finding that “The average fixation time of AOI and the pupil area can be used as an effective indicator of driver fatigue detection” [13].
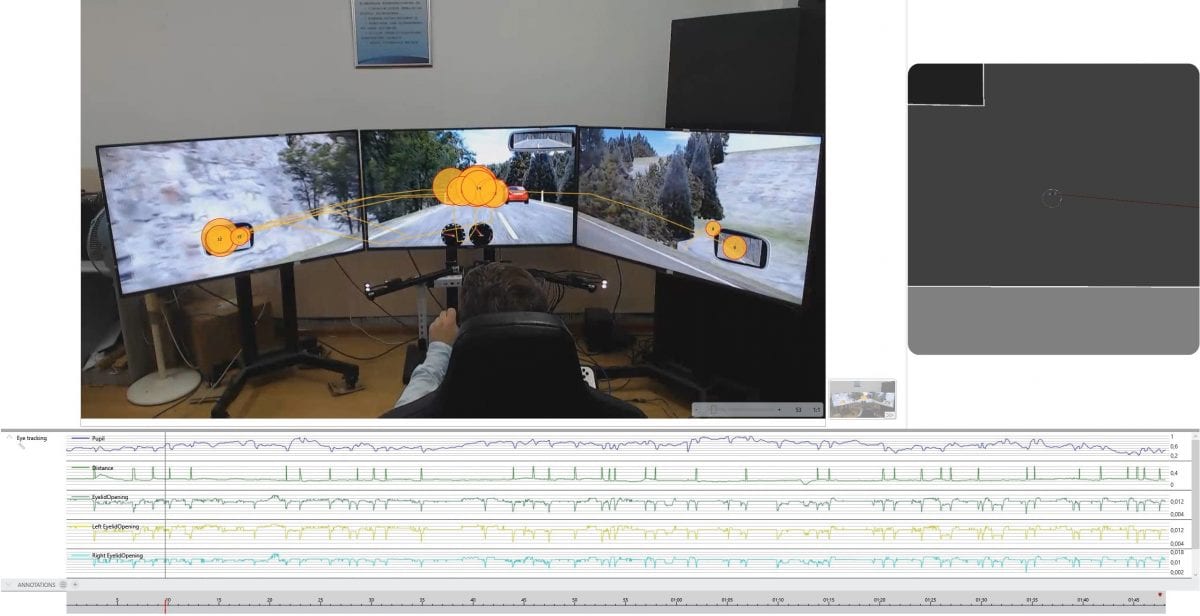
Other researchers have employed machine learning models in order to predict the level of fatigue that a participant is experiencing based on eye tracking data [14]. Eye closure percentage data has also been used as a valid predictor of fatigue levels [15]. Researchers have also just used measures of eyelid distance, with an average precision rate of fatigue of 90% [16]. These are just some examples showing the sensitivity of eye tracking data to provide information about the level of fatigue that an individual is experiencing.
Eye tracking methods can also be used in context beyond driving scenarios – adaptable methodologies with multiple camera tracking, or eye tracking glasses can be used to detect eye movements in challenging scenarios. This could be easily applied to pilots, air traffic controllers, or drone operators – providing a better understanding of when a worker is operating well, and when they will need to take a break.
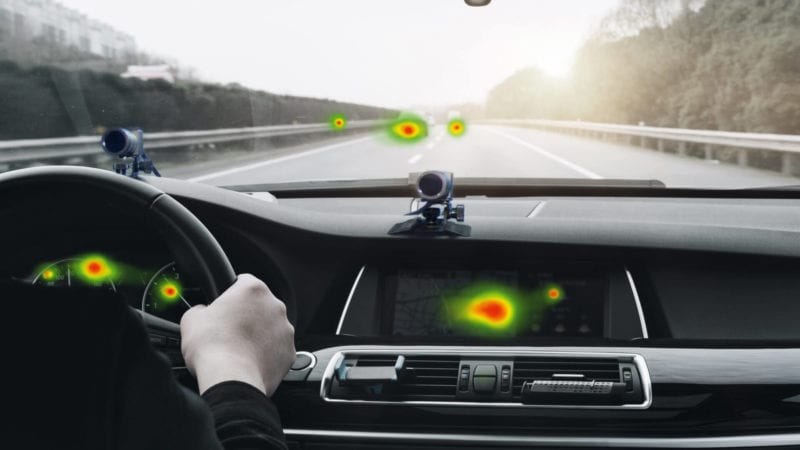
Facial expression analysis is another example of a methodology that can relay information about the level of tiredness that an individual is experiencing in a non-intrusive way. Either specific movements (such as yawning), or the collection of multiple facial movements can be used to predict levels of fatigue. Several researchers have used facial expression data that is automatically collected from systems like FACET and Affectiva to build machine learning models that can accurately predict the onset of drowsiness (e.g. [17-19]).
Another central methodology that can be reliably applied to detect levels of fatigue is electroencephalography, or EEG. This technology is particularly well suited to measuring drowsiness levels as it directly measures the activity of the brain [20]. Researchers have used both wired and wireless EEG headsets to detect increased incidences of wavelengths corresponding to the onset of drowsiness (e.g. an increase of slow wave occipital activity [21]).
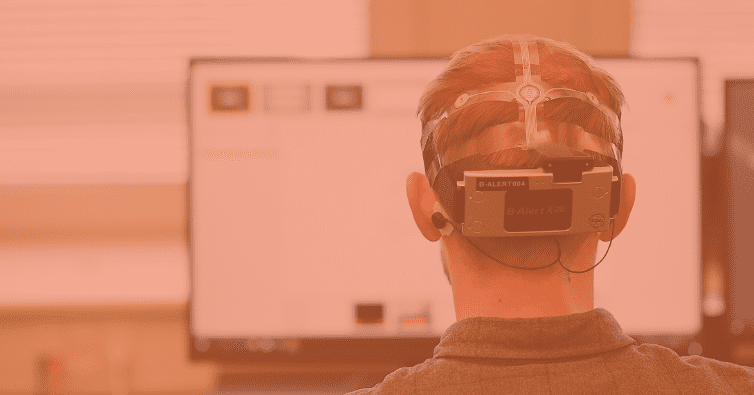
Researchers have also capitalised on the clear association between heart rate activity and level of physiological / psychological awareness. Measures such as heart rate and heart rate variability are able to reliably convey information about the onset of fatigue, as well as providing a more specific measure of cognitive load [22, 23].
It’s also possible to predict fatigue through measurements of electrodermal activity (EDA, also sometimes referred to as GSR). Researchers have used it to monitor the association between drowsiness and reductions in reaction times [24]. This has also been replicated within automotive driving tasks [25], and it has even been used to assess anxiety brought on by fatigue [26].
Conclusion
The effects of fatigue can be disastrous – the increased numbers of accidents and fatalities that occur when people are fatigued are testament to that. Fortunately a wide range of methods are available to track, measure, and predict when the risk of accident increases to unsafe levels. The varied nature of tasks that individuals can be faced with will inevitably mean that each situation will need to be examined in order to provide accurate assessment of safety and success, but the tools and capabilities are available. All that’s left is to get started.
I hope you’ve enjoyed reading about fatigue detection – if you’d like to learn more about one of the central methods that is used to track fatigue, download our free eye tracking guide below.
Eye Tracking
The Complete Pocket Guide
- 32 pages of comprehensive eye tracking material
- Valuable eye tracking research insights (with examples)
- Learn how to take your research to the next level
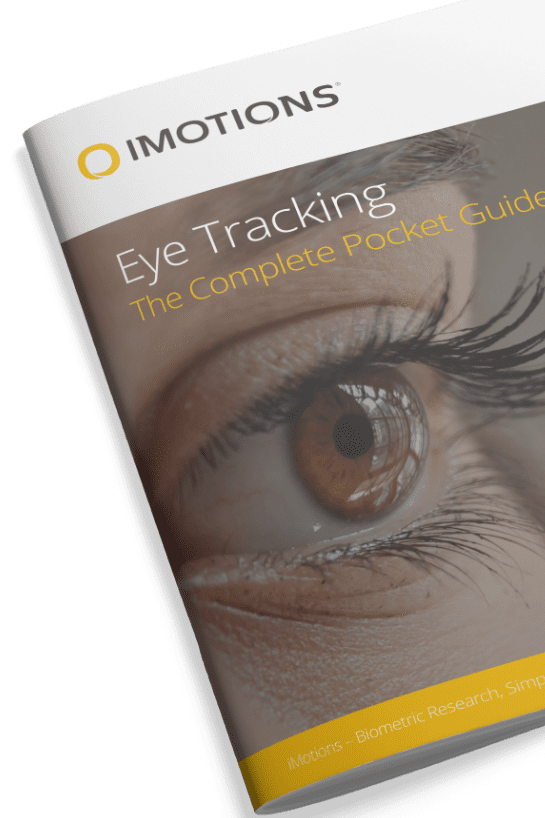
References
[1] Dement, William C. (1999). The Promise of Sleep. New York: Dell Publishing.
[2] Klauer SG, Dingus TA, Neale VL, Sudweeks JD, Ramsey DJ. The Impact of Driver Inattention on Near-Crash/Crash Risk: An Analysis Using the 100-Car Naturalistic Study Data, 2006. Springfield, VA: DOT; year. DOT HS 810 594.
[3] Tefft, B. C., AAA Foundation for Traffic Safety. Prevalence of Motor Vehicle Crashes Involving Drowsy Drivers, United States, 2009 – 2013 [457 KB]. Washington, DC: AAA Foundation for Traffic Safety; 2014. October 19, 2015.
[4] Institute of Medicine. Sleep Disorders and Sleep Deprivation: An Unmet Public Health Problem. Washington, DC: The National Academies Press; 2006.
[5] Shahly V, Berglund PA, Coulouvrat C, et al. The Associations of Insomnia With Costly Workplace Accidents and Errors: Results From the America Insomnia Survey. Arch Gen Psychiatry. 2012;69(10):1054–1063.
[6] Adams A, Soumerai S, Lomas J, Ross-Degnan D. (1999). Evidence of selfreport bias in assessing adherence to guidelines. Int J Qual Health Care; 11(3): 187–192.
[7] Dalziel, J.R., Job, R.F. (1997). Motor vehicle accidents, fatigue and optimism bias in taxi drivers. Accident Analysis Prevention 29, 489–494.
[8] Barofsky, I., Legro, M. W. (1991). Definition and Measurement of Fatigue. Reviews of Infectious Diseases, Volume 13, Issue Supplement_1, January-February, Pages S94–S97, https://doi.org/10.1093/clinids/13.Supplement_1.S94
[9] W. Horng, C. Chen, Y. Chang, C. Fan, Driver fatigue detection based on the eye tracking and dynamic template matching, in: Proceedings of the IEEE International Conference on Networking, Sensing and Control, Taiwan, vol. 1, 2004, pp. 7–12.
[10] H. Huang, Y. S. Zhou, F. Zhang, F. C. Liu. (2007). An optimized eye locating and tracking system for driver fatigue monitoring. In Proc. ICWAPR, vol. 3., pp. 1144–1149
[11] R. C. Coetzer and G. P. Hancke (2011). Eye detection for a real-time vehicle driver fatigue monitoring system. In Proc. IEEE Intell. Veh. Symp., Jun, pp. 66–71
[12] C. A. Perez, A. Palma, C. A. Holzmann, and C. Pena. (2001). Face and eye tracking algorithm based on digital image processing. In Proc. IEEE Int. Conf. Systems, Man, Cybernetics, vol. 2, Oct., pp. 1178–1183.
[13] Xu J, Min J, Hu J. Real-time eye tracking for the assessment of driver fatigue. Healthc Technol Lett. 5(2):54-58. doi:10.1049/htl.2017.0020.
[14] Yamada, Y., Kobayashi, M. (2018). Detecting mental fatigue from eye-tracking data gathered while watching video: evaluation in younger and older adults, Artif.Intell. Med. 91, 39e48,https://doi.org/10.1016/j.artmed.2018.06.005.
[15] Lin, L., Huang, C., Ni, X., Wang, J., Zhang, H., Li, X., & Qian, Z. (2015). Driver fatigue detection based on eye state. Technology and Health Care, 23(2), 453–463.
[16] Wenhui Dong and Xiaojuan Wu. (2005). Fatigue detection based on the distance of eyelid. Proceedings of 2005 IEEE International Workshop on VLSI Design and Video Technology, Suzhou, China, 2005, pp. 365-368.
[17] Henni, K., Mezghani, N., Gouin-Vallerand, C. et al. (2018). Appl Inform, 5: 7. https://doi.org/10.1186/s40535-018-0054-9
[18] Gu, H. & Ji, Q. (2004). An automated face reader for fatigue detection, Proc. IEEE Int’l Conf. Face and Gesture Recognition, pp. 111-116.
[19] J. Qiang, Z. Zhiwei, and P. Lan. (2004). Real-time nonintrusive monitoring and prediction of driver fatigue. IEEE Trans. Veh. Technol., vol. 53, pp. 1052–068, Jul.
[20] Borghini, G., Astolfi, L., Vecchiato, G., Mattia, D., and Babiloni, F. (2014). Measuring neurophysiological signals in aircraft pilots and car drivers for the assessment of mental workload, fatigue and drowsiness. Neurosci. Biobehav. Rev. 44, 58–75. doi: 10.1016/j.neubiorev.2012.10.003
[21] Neu, D., Mairesse, O., Verbanck, P., Linkowski, P., and Le Bon, O. (2014). Non-REM sleep EEG power distribution in fatigue and sleepiness. J. Pychosom. Res. 76, 286–291. doi: 10.1016/j.jpsychores.2014.02.002
[22] Segerstrom, S. C., & Nes, L. S. (2007). Heart rate variability reflects self-regulatory strength, effort, and fatigue. Psychological Science, 18, 275–281. doi:10.1111/j.1467-9280.2007.01888.x
[23] Patel, M.; Lal, S.K.L.; Kavanagh, D.; Rossiter, P. (2001). Applying neural network analysis on heart rate variability data to assess driver fatigue. Exp. Syst. Appl. 38, 7235–7242.
[24] Miro E, Cano-Lonzao MC, Buela-Casal G. (2002). Electrodermal activity during total sleep deprivation and its relationship with other activation and performance measures. J Sleep Res. 11:105–12.
[25] Dorrian, J., Lamond, N., Kozuchowski, K., & Dawson, D. (2008). The driver vigilance telemetric control system (DVTCS): Investigating sensitivity to experimentally induced sleep loss and fatigue. Behavior Research Methods, 40, 1016-1025.
[26] Rani, P., Sarkar, N., Smith, C,A,, Kirby, L.D. (2004). Anxiety detecting robotic system—towards implicit human-robot collaboration. Robotica, 22:85–95.