How are simulations used in human behavior research? This article delves into the significance of simulations in understanding human behavior. Exploring the methods and implications, it sheds light on the integral role of simulations in contemporary research on human behavior.
Table of Contents
Balancing Control and Realism in Human Behavior Research: Lab vs. Real-World Studies
Human behavior research has often grappled with choosing between two alternative approaches to experimental work – studying behavior in controlled, or natural environments.
On the one hand, carefully controlled lab settings allow fine-tuned changes to experimental setups to be made – the participants all experience the same setting, with little room for experimental noise to be introduced, and a single experimental variable is adjusted.
The experiment works well as it’s possible to measure how human behavior can vary in response to an identifiable change. The downside is that these environments are inherently unnatural – by removing the chance of variation it’s often the case that the environment represents real life less and less.
On the other hand, experiments carried out “in the wild” allow participants to behave and experience the world in a way that is normal and natural. A single experimental variable will be changed, but the chance that experimental noise can be introduced is high. This means that more natural behavior will be carried out, but it’s more difficult to assure that any change is solely due to the experimental variable.
If we want to really understand human behavior (and spoiler alert: we do), we often need to compromise between these two approaches. We need to assess how much noise we think will be introduced, and how much variation is acceptable to create an environment that feels right to the participant. Making that decision is a big part of the experimental design process. However, there is sometimes a third way: simulations.
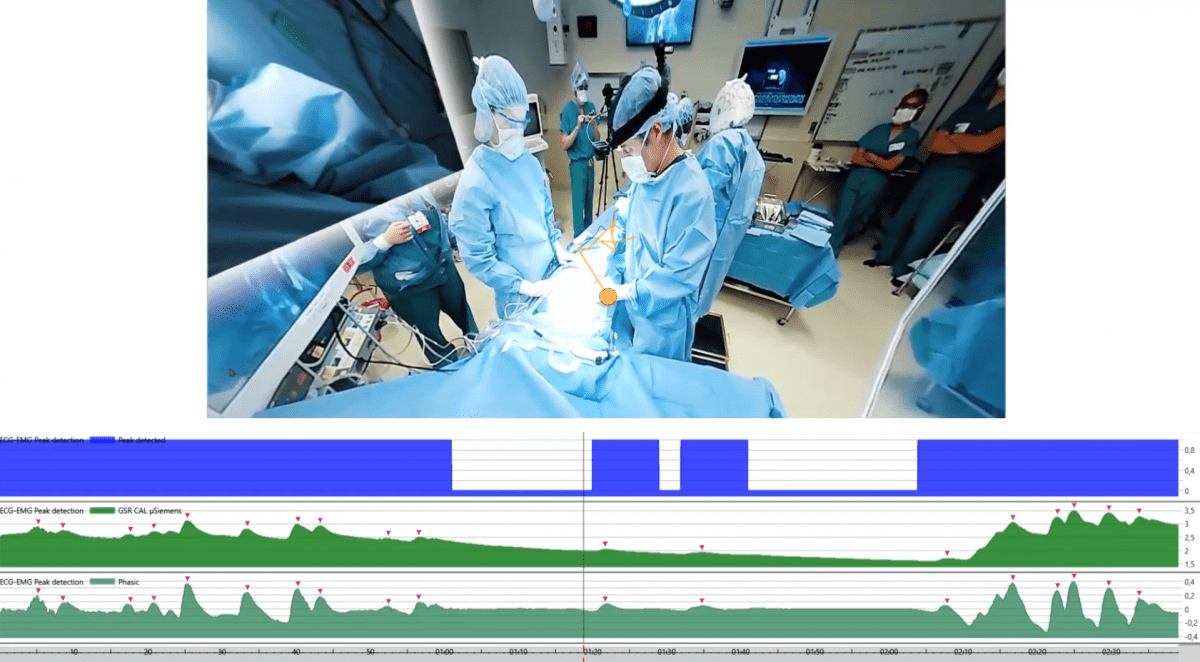
What is a Simulation?
A simulation is an imitation of a real thing. Within human behavior research, it often takes the form of a controlled presentation of a setting that can’t reasonably be experienced by a participant. While it may be interesting and important to know how a pilot reacts in dangerous flying conditions, it’s clearly not ideal to make this happen in real life.
Simulations allow us to not only test responses to settings that are dangerous, it also allows us to precisely replicate them. We can see how different pilots (or non-pilots for that matter) react to the same dangerous setting time and time again.
A flight simulator is an obvious example of a commonly used simulation for assessing behavior, but there are now a surprising variety of different simulators, all aimed at measuring responses in high-fidelity environments. With the rise of VR, it’s now also possible to create entirely new environments (and even to create unreal ones) with little relative cost.
These environments have become both more accessible to researchers and participants, with a better understanding of aspects such as motion sickness [1].
Below, we’ll go through examples of different simulation scenarios, and how researchers are investigating human behavior in each.
Enhancing Driver Safety and Behavior Research with Advanced Driving Simulations
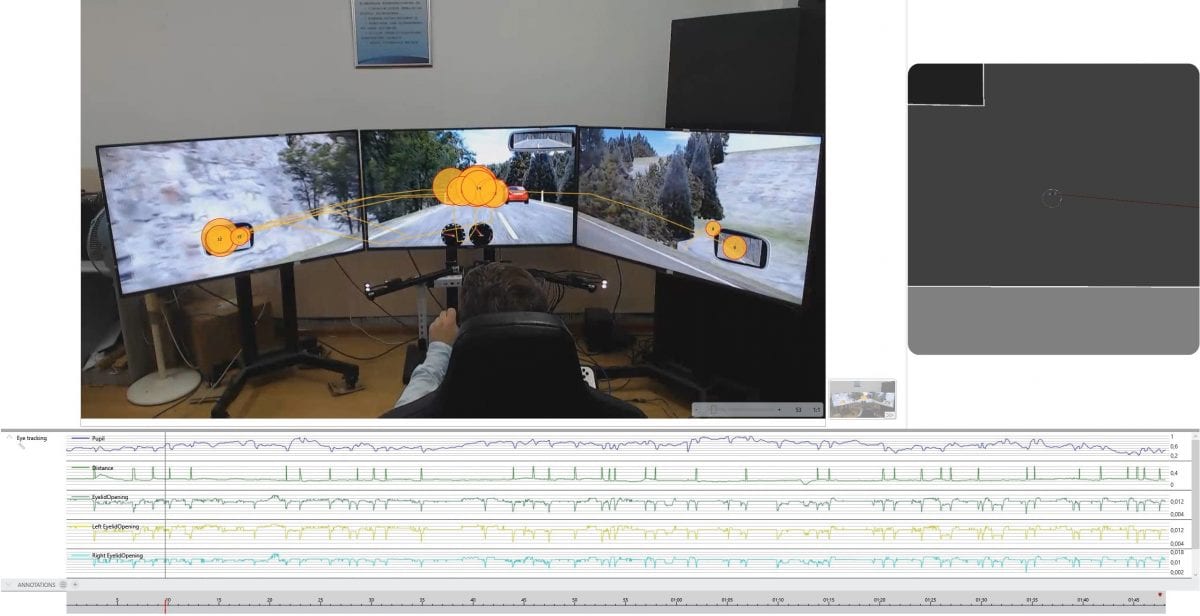
Driving simulations are familiar to many of us. Found in a similar form in many games arcades, the simulators provide a safe environment in which to test driving skills and responses, complete with a steering wheel, gears, and sometimes much more.
The driving lab at Stanford uses a real car surrounded by high-definition screens to provide an immersive experience of driving a car. The data from this vehicle and other sensors are all integrated within iMotions to help the researchers understand driver behavior.
Research often focuses on how to improve driver (and pedestrian) safety by testing which factors can impact the likelihood of an accident. Testing out new features that can improve (or worsen) driver awareness can be readily completed inside a simulator without any real risk.
Researchers have used sensors such as EEG to investigate mind-wandering in a driving simulator [2], finding that not only can this mental state have a negative effect on driving ability, it can also be detected – opening up the possibility of equipping cars with sensors (possibly, although not necessarily EEG) that can provide relevant warnings should the driver’s attention shift too far from the road.
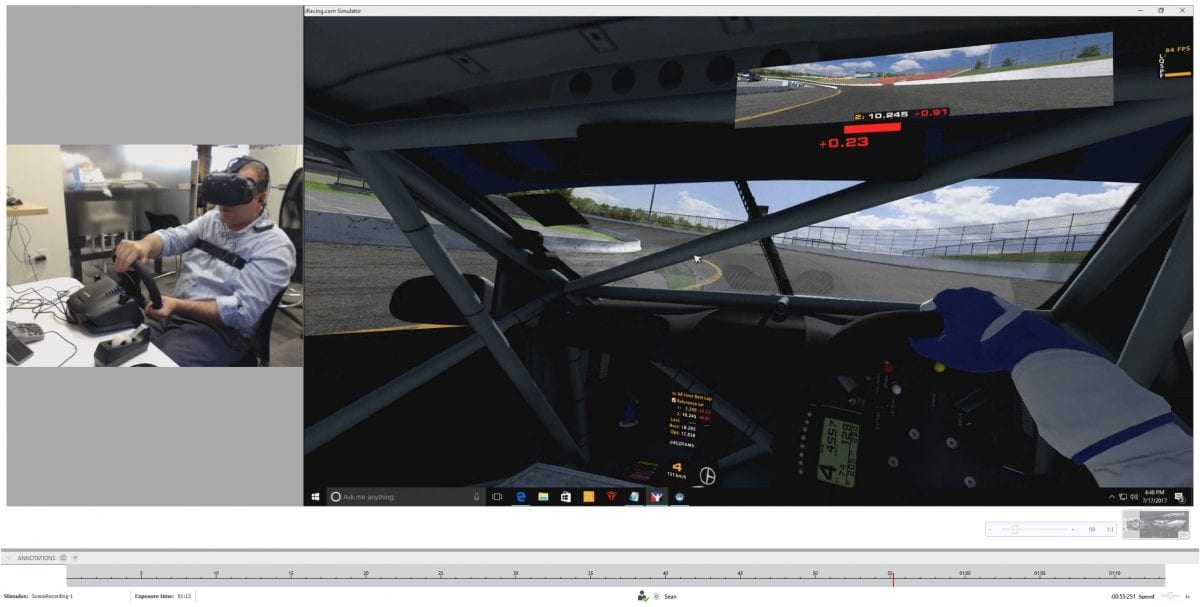
Eye tracking is also a popular tool used within driving simulators, as it offers the possibility of implementation into a real-world vehicle without the technical constraints that EEG imposes. Researchers have used eye tracking to estimate cognitive load [3] within a driving simulator, suggesting this could be used as a method to monitor the cognitive states of drivers.
Both of these methods – EEG and eye tracking – have also been combined with iMotions in a driving simulator to test out an early warning system for drivers [4]. Cognitive load and the measurement of cognitive states through EEG was used in a preliminary test of driving behavior. It was found that these sensors could indicate whether or not an early warning system of danger needed to be delivered or if the driver was already alert enough.
Beyond driving simulators, researchers also explore behavior in a variety of other vehicle simulators, from flight simulations, to maritime research in ship simulators (Force Technologies uses iMotions to assess ship steering behavior in a fully-rotational freight ship simulation).
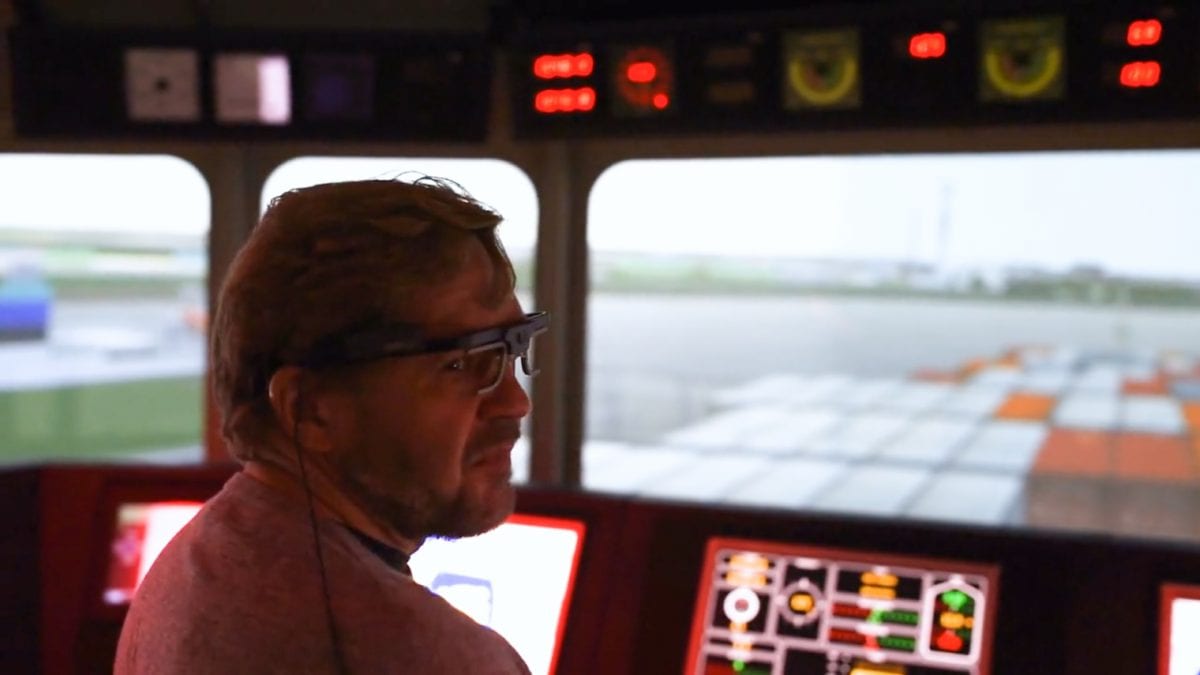
Work Simulations: Advancing Human Behavior Research Across Industries
Simulations aren’t necessarily bound to a vehicle, but can also take place in environments where participants can freely move around. Researchers have, for example, used eye tracking within medical education contexts to evaluate how well each aspect of a protocol was attended to [5].
Simulations determining control room operator performance, in contexts ranging from nuclear power plants [6], petrochemical power stations [7], and air traffic control towers [8, 9, 10] commonly use eye tracking and / or EEG to assess the attentional and cognitive resources of operators.
Researchers at the University of Luleå use iMotions and a variety of sensors to study the cognitive processes occurring in naturalistic simulations of train traffic controllers.
The use of simulations can even be expanded into military applications. Researchers have gone to great lengths to recreate realistic simulations of weapons [11] and war-like environments [12] in order to understand the cognitive and physiological pressure that soldiers are often placed under.
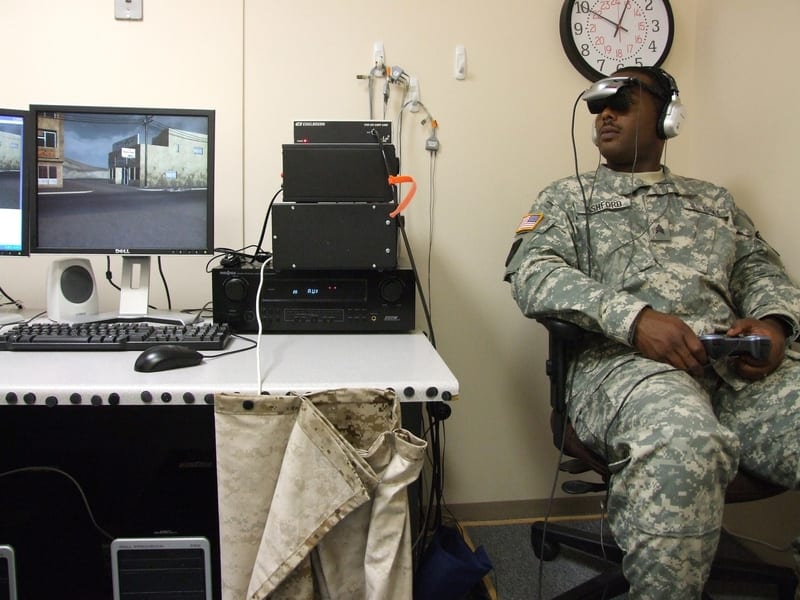
Various investigations have also focused on how military pilots respond to demanding situations, in which quick decisions have to be made. Eye tracking has been used in this context to investigate the impact of visual load (how much visual information is presented), and how this can impact the decision-making capabilities of the pilots [13].
Researchers have also explored the use of EEG and heart rate measures within both real flight and flight simulations [14]. The results indicated that the most challenging aspects of the operation could be identified on an individual basis for the pilots, providing data that could inform which aspects of training would need more work for each pilot.
If you are thinking about what over fields use can use biometrics in relation to human behavior research, checkout our blog listing 100 common application areas.
To get a better understanding of one of the central modalities in research using simulations, download our free eye tracking guide below.
Eye Tracking
The Complete Pocket Guide
- 32 pages of comprehensive eye tracking material
- Valuable eye tracking research insights (with examples)
- Learn how to take your research to the next level
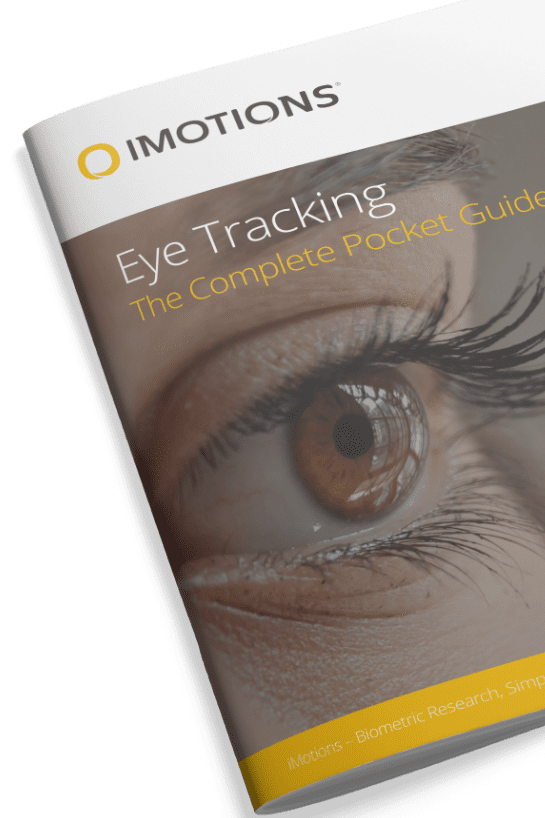
References
[1] Gavgani, A. M., Nesbitt, K. V., Blackmore, K. L., Nalivaiko, E. (2017). Profiling subjective symptoms and autonomic changes associated with cybersickness. Autonom Neurosci. 203:41–50. pmid:28010995.
[2] Baldwin, C. L., Roberts, D. M., Barragan, D., Lee, J. D., Lerner, N., & Higgins, J. S. (2017). Detecting and quantifying mind wandering during simulated driving. Frontiers in Human Neuroscience, 11, 406.
[3] Palinko, O., Kun, A. L., Shyrokov, A., & Heeman, P. (2010). Estimating cognitive load using remote eye tracking in a driving simulator. In Proceedings of the 2010 Symposium on Eye-Tracking Research & Applications (pp. 141–144). New York, NY: ACM Press.
[4] E, Pakdamanian., L. Feng., I, Kim. (2018). The Effect of Whole-Body Haptic Feedback on Driver’s Perception in Negotiating a Curve. Proceedings of the Human Factors and Ergonomics Society Annual Meeting, 62, 19-23.
[5] Henneman, E. A., Cunningham, H., Fisher, D. L., et al. (2014). Eye tracking as a debriefing mechanism in the simulated setting improves patient safety practices. Dimens Crit Care Nurs, 33:129–135.
[6] Kovesdi, C., Rice, B., Bower, G., Spielman, Z, Hill, R., and LeBlanc, K. (2015). Measuring Human Performance in Simulated Nuclear Power Plant Control Rooms Using Eye Tracking, INL/EXT-15- 37311.
[7] Ikuma, L. H., Harvey, C., Taylor, C. F., and Handal, C. (2014). A guide for assessing control room operator performance using speed and accuracy, perceived workload, situation awareness, and eye tracking. Journal of Loss Prevention in the Process Industries, 32: 454-465.
[8] Kearney, P., Li, W. C., Yu, C. S., Braithwaite, G. (2018). The impact of alerting designs on air traffic controller’s eye movement patterns and situation awareness. Ergonomics, 5:1-14.
[9] Giraudet, L., Imbert, J. P., Bérenger, M., et al. (2015). The neuroergonomic evaluation of human machine interface design in air traffic control using behavioral and EGG/ERP measures. Behavioural Brain Research, vol. 294, pp. 246-53.
[10] Aricò, P., Borghini, G., Di Flumeri, G., Colosimo, A., Bonelli, S., Golfetti, A., et al. (2016). Adaptive automation triggered by EEG-based mental workload index: a passive brain-computer interface application in realistic air traffic control environment. Front. Hum. Neurosci. 10:539.
[11] L. C. A. Campos, and L. L. Menegaldo. (2018). A battle tank simulator for eye and hand coordination tasks under horizontal whole-body vibration. Journal of Low Frequency Noise, Vibration and Active Control, Vol. 37(1) 144–155.
[12] Saus, E. R., Johnsen, B. H., Eid, J., Riisem, P. K., Andersen, R., and Thayer, J. F. (2006). The effect of brief situational awareness training in a police shooting simulator: an experimental study. Mil. Psychol. 18, S3–S21.
[13] Kacer, J., Kutilek, P., Krivanek, V., Doskocil, R., Smrcka, P., & Krupka, Z. (2017). Measurement and Modelling of the Behavior of Military Pilots. In International Conference on Modelling and Simulation for Autonomous Systems (pp. 434-449). Springer, Cham.
[14] G. Kloudova, M. Stehlik. (2017). The Enhancement of Training of Military Pilots Using Psychophysiological Methods. International Journal of Psychological and Behavioral Sciences, Vol:11, No:11.[/fusion_builder_column][/fusion_builder_row][/fusion_builder_container]