Voice analysis is the study of vocal characteristics and patterns in spoken communication. It involves examining elements such as pitch, tone, speech rate, and emotional cues to gain insights into aspects like linguistics, emotions, and individual traits. This technology has applications in fields such as linguistics, psychology, and healthcare.
Imagine you’re in a country you haven’t been to before. You don’t speak the language, and you certainly don’t understand it. You approach someone, or perhaps they approach you, and before you know it, they’re deep-diving into a string of sentences you have no chance of comprehending. Except, you do understand a few fundamental things. The context will of course help frame what’s being said, but there’s something else that reveals a lot about what else is going on – the way in which the person speaks.
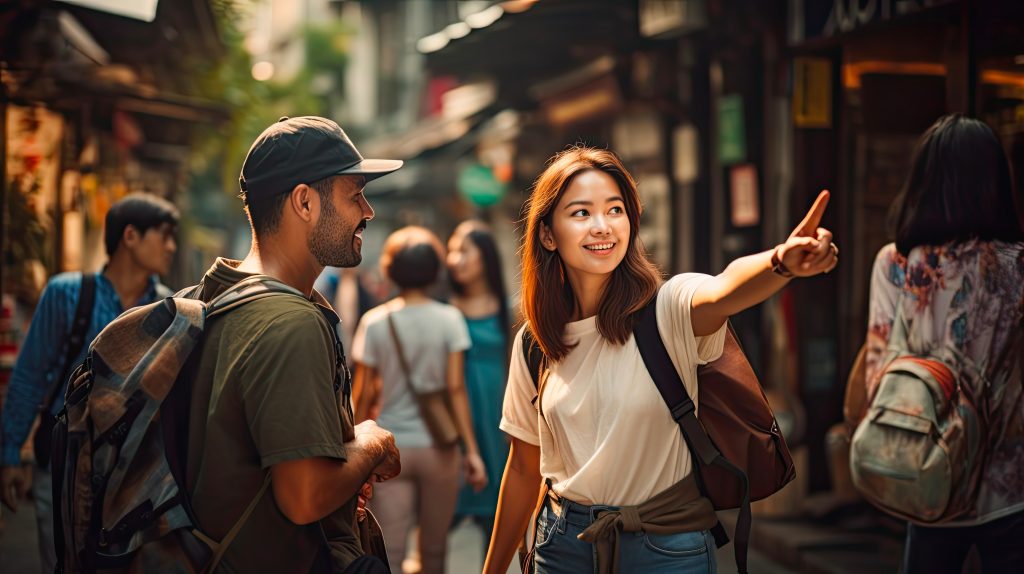
What we’re doing at that moment is analyzing the voice – we can hear shifts in tone, prosody, pitch (and more), and deduce whether the person is happy or sad, angry, or engaged. The information tells us a lot about the inner state of the person. We usually don’t have to be explicitly told about the speaker’s emotions – we automatically analyze the voice and know the answer.
This phenomenon – what we can refer to today as voice analysis – has of course been known as long as people have been talking, and initial attempts at measuring vocal production have been going on since at least the late 1900s [1].
This later became more formalized in the 1960s with rigorous scientific analyses [2], but only with the advent of modern computing has voice analysis as a scientific field really taken shape. Now we are at the dawn of the next shift in the science of voice analysis with the emergence of AI methods.
But what is voice analysis, and how can it used to better understand human emotions and behavior? And how does AI help? Below we will go through some of the central ways in which voice analysis is already helping researchers make advances in health research, human-computer interaction, UX, and understanding decisions such as purchase intent.
What is voice analysis?
Voice analysis is the process by which vocal sounds are measured and associated with defined metrics – such as the emotion, age, gender, or presence/absence of speech. Crucially, voice analysis doesn’t provide any direct information about any words used, but rather the way in which they were produced.
The method involves segmenting the produced sound, and extracting multiple features which can then be assessed either independently or together. Different algorithms work in different ways, but usually features such as prosody, rate, and intonation are collected and analyzed. These are then used to build a prediction of higher-dimension characteristics of speech, such as whether the person is happy or angry.
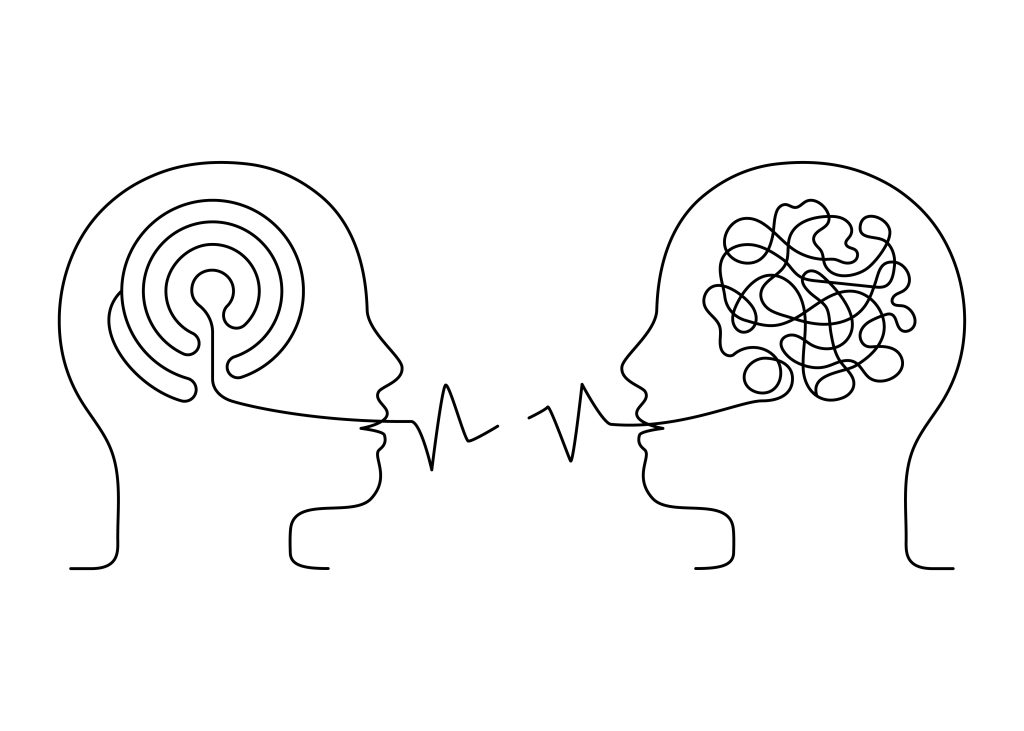
Measurements of both the fundamental mechanics of speech, and broader emotional results are used to provide information about human behavior. One of the fields showing particular promise with this approach is within healthcare.
Voice analysis in healthcare
The advantage of analyzing voice in healthcare is twofold. First, it’s almost effortlessly collected as a form of data, which can be recorded (with consent) from the very first point of contact. Secondly, it shows robust promise as a predictor of a wide range of neurological, psychiatric, and vocal disorders.
One of the first studies examining the relationship between voice and physiology was carried out by Meyer Friedman, who popularized the Type A and Type B personality theory [3]. This theory broadly splits people into type A or type B groupings, with Type As more “competitive, highly organized, ambitious, impatient” and Type Bs as more “relaxed, receptive, and less neurotic”.
The research group found, through a voice analysis test, that they could differentiate Type A participants and heart disease patients from Type B participants. This was taken to suggest that personality types could be differentiated on the basis of voice alone.
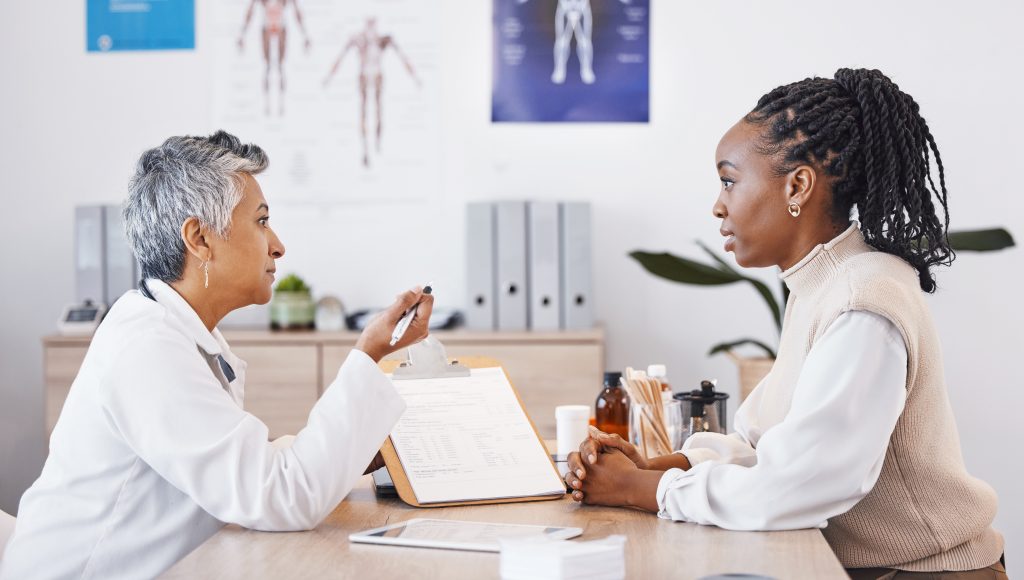
While the theory of Type A and Type B personalities later underwent rightful criticism, leaving it essentially debunked (see for example [4 and 5]), the approach of using voice with disease biomarkers had begun. For example, recent research has shown how biomechanical elements can be extracted from an app alone and used to identify Parkinson’s disease [6].
Research has also utilized brain scans of Parkinson’s patients to show a relationship between brain damage in areas associated with emotion processing, and emotional speech, tying a direct link between the brain and voice within the neurological disorder [7].
Research has gone on to exhibit the predictive capacity of voice to identify Parkinson’s disease, Alzheimer’s disease, depression, amyotrophic lateral sclerosis (ALS), bipolar disorder, and even long Covid [8, 9]. The methods for study design, recording, feature extraction, and analysis widely vary across studies, yet point in a consistent direction – to robust, early identification of health-impacting factors.
Several studies and companies have highlighted the promise of automated voice condition analysis (AVCA) for future healthcare applications. Ultimately, voice analysis shows great promise as an inexpensive, almost passive mode of early detection and diagnosis for a wide range of diseases and disorders.
Voice analysis in human-computer interaction
Voice analysis also provides another route for the improvement of healthcare beyond early diagnosis – by assisting the access and emotional understanding of healthcare-providing tools. One such example is that of telehealth, and particularly teletherapy.
Research has pointed toward integrating voice analysis measures within video or call-based therapy, providing practitioners with data that can guide their assessment of the patient. A recent study showed how suicide risk could be identified – potentially giving life-saving information to the therapist on the call [10].
Other studies have pointed towards the way in which doctor-patient interactions can be more readily, and objectively, assessed by voice analysis methods [11]. This can provide data for evidence-based improvements to be suggested for future calls.
This technique can similarly be applied in real-time to therapeutic chatbots. Various articles have discussed how these increasingly prevalent chatbots can integrate voice analysis methods to allow for responses that are more attuned to the patient’s needs through an assessment that goes beyond simply what the patient says [12, 13, 14].
This emotionally intelligent interactivity that can be provided by integration with voice analysis is of course not limited to therapeutic settings alone. Rather, any systematic interaction involving a human speaking can likely benefit from data that can enhance the emotional awareness of the person – or AI – that responds.
Voice analysis in UX
A similar approach as with HCI can be – and is – applied to UX design. This is specifically the case with think-aloud protocols, in which a test user voices their thoughts as they use or navigate through a newly designed product. The product, which can be anything from a website, to a physical item, to packaging, is trialed by someone who has not seen it before. The user simply states what comes to mind during the process.
Biosensors are already widely used to provide UX designers with an understanding of a user’s thoughts beyond what they simply state out loud. Eye tracking and facial expression analysis can provide data that provides insight into what people actually focus on, and how their emotional expressions relate to that.
Voice analysis presents the next logical step in this scientific progression, allowing designers to interrogate the fundamental biological component of think-aloud protocols. However, as researchers from the University of Toronto and Rochester Institute of Technology have remarked, “analyzing think-aloud sessions is often time-consuming and labor-intensive” [15].
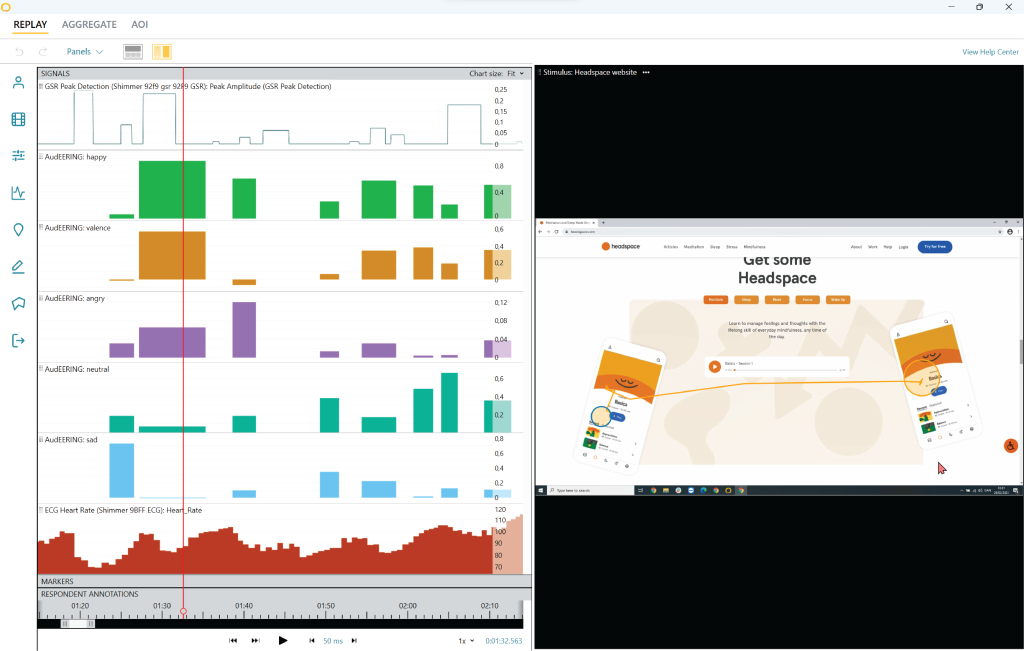
The researchers went on to develop their own internal machine learning method for analyzing verbalizations during the think-aloud protocol – time can even be saved here by using readily available models such as devAIce from audEERING.
Researchers are already combining facial expression analysis with voice analysis [16] for testing “emergency situations on a naval ship”, “a flood (crisis) situation”, and “players immersed in a virtual reality game”, finding promising results for future research. Other researchers have used voice analysis with think-aloud protocols for examining learning with web-based education [17], and for setting up analyses of gameplay experience [18].
Voice analysis in consumer neuroscience
The approach of analyzing voice within UX development has overlaps with the way in which it is often – but not exclusively – applied within consumer neuroscience. A product or platform is built, and a user interacts with it – that interaction is then ultimately measured as positive, negative, or neutral within discrete moments.
While the application of voice analysis within consumer neuroscience or neuromarketing contexts has been discussed since at least the 1980’s [20] – with researchers noting how it “can identify the ‘sincere’ versus the ‘lip-service’ response” [21] – it was also noted that the research of the time had “flawed research instruments, and a resultant overstatement of the findings”. This necessitated a need for improved computational methods of analysis (now readily available), and a reassessment of study designs for collecting voice data. This led to the overlaps we now see with the field of UX research.
While participants within a consumer neuroscience study might not spontaneously voice their thoughts when interacting with a new product or advertisement, analyzing their verbal responses during think-alouds, interviews, and focus groups provides a new dimension to the understanding of their inner thoughts and feelings.
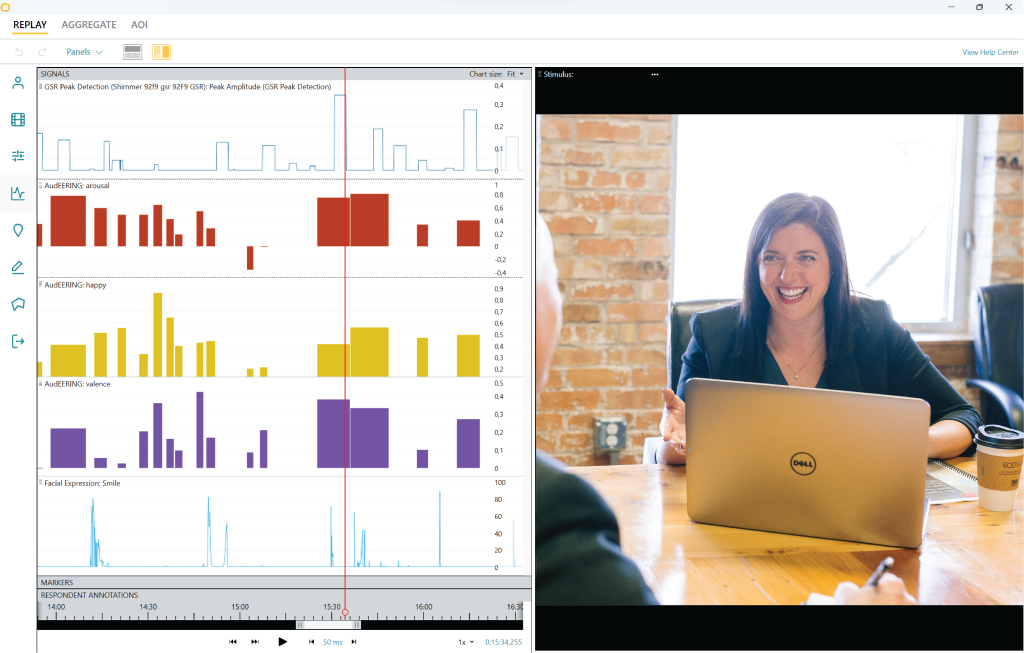
Ensuring there is a natural environment in which they can speak is crucial to making the most of voice analysis. This is, for example, shown within a study of online beauty and fashion articles in which a think-aloud and interview are used to assess voice data alongside eye tracking and facial expression analysis [19].
While analyzing consumers with voice analysis in response to adverts can be carried out (in combination with an interview or similar), the opposite is also true. A 2019 study from the University of Tohoku, in Japan, found that consumers listening to adverts for food products were more likely to prefer sweet or sour foods when the voice was at a high pitch [22].
They found that a “considerably high” vocal pitch was likely to encourage a specific preference for sweet foods. This shows another route by which neuromarketers can further understand and improve the resonance of the products they help bring to market.
Conclusion
While voice analysis is a relatively established methodology within diagnostic research, it has been a largely underexplored and underutilized tool in the kit of human behavior metrics. In the past, this has been due to the laborious work required to classify and decode vocal signals, and the lack of robust available methods. However, we are now, with refinements in software and advances in AI, able to see the true promise that this methodology holds for opening up and exploring this central signal of human behavior.
Free 22-page Voice Analysis Guide
For Beginners and Intermediates
- Get a thorough understanding of the essentials
- Valuable Voice Analysis research insight
- Learn how to take your research to the next level
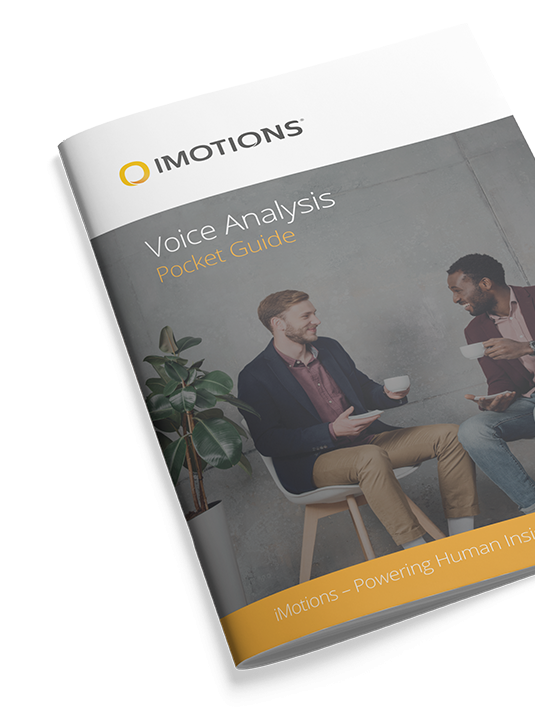
References
[1] Muckey, F. S. (1915). The natural method of voice production. The English Journal, 4(10), 625. https://doi.org/10.2307/801210
[2] Friedman, M. (1969). Voice analysis test for detection of behavior pattern. JAMA, 208(5), 828. https://doi.org/10.1001/jama.1969.03160050082008
[3] Meyer Friedman; Carl E. Thoresen; James J. Gill; Diane Ulmer; Lynda H. Powell; Virginia A. Price; Byron Brown; Leonti Thompson; David D. Rabin; William S. Breall; Edward Bourg; Richard Levy; Theodore Dixon (1 October 1986). “Alteration of type A behavior and its effect on cardiac recurrences in post heart attack patients: Summary results of the recurrent coronary prevention project”. American Heart Journal. 112 (4): 653–665. doi:10.1016/0002-8703(86)90458-8. PMID 3766365.
[4] Petticrew, M. P., Lee, K., & McKee, M. (2012). Type A behavior pattern and coronary heart disease: Philip Morris’s “Crown jewel.” American Journal of Public Health, 102(11), 2018–2025. https://doi.org/10.2105/ajph.2012.300816
[5] Wilmot, M. P., Haslam, N., Tian, J., & Ones, D. S. (2019). Direct and conceptual replications of the taxometric analysis of type a behavior. Journal of personality and social psychology, 116(3), e12–e26. https://doi.org/10.1037/pspp0000195
[6] Romero Arias, T., Redondo Cortés, I., & Pérez Del Olmo, A. (2023). Biomechanical parameters of voice in Parkinson’s disease patients. Folia phoniatrica et logopaedica : official organ of the International Association of Logopedics and Phoniatrics (IALP), 10.1159/000533289. Advance online publication. https://doi.org/10.1159/000533289
[7] Anzuino, I., Baglio, F., Pelizzari, L., Cabinio, M., Biassoni, F., Gnerre, M., Blasi, V., Silveri, M. C., & Di Tella, S. (2023). Production of emotions conveyed by voice in Parkinson’s disease: Association between variability of fundamental frequency and gray matter volumes of regions involved in emotional prosody. Neuropsychology, 10.1037/neu0000912. Advance online publication. https://doi.org/10.1037/neu0000912
[8] Hecker, P., Steckhan, N., Eyben, F., Schuller, B. W., & Arnrich, B. (2022). Voice Analysis for Neurological Disorder Recognition–A systematic review and perspective on emerging trends. Frontiers in Digital Health, 4. https://doi.org/10.3389/fdgth.2022.842301
[9] Lin, C. W., Wang, Y. H., Li, Y. E., Chiang, T. Y., Chiu, L. W., Lin, H. C., & Chang, C. T. (2023). COVID-related dysphonia and persistent long-COVID voice sequelae: A systematic review and meta-analysis. American journal of otolaryngology, 44(5), 103950. https://doi.org/10.1016/j.amjoto.2023.103950
[10] Iyer, R., Nedeljkovic, M., & Meyer, D. (2022). Using Voice Biomarkers to Classify Suicide Risk in Adult Telehealth Callers: Retrospective Observational Study. JMIR mental health, 9(8), e39807. https://doi.org/10.2196/39807
[11] Habib, M., Faris, M., Qaddoura, R., Alomari, M., Alomari, A., & Faris, H. (2021). Toward an Automatic Quality Assessment of Voice-Based Telemedicine Consultations: A Deep Learning Approach. Sensors (Basel, Switzerland), 21(9), 3279. https://doi.org/10.3390/s21093279
[12] Jadczyk, T., Wojakowski, W., Tendera, M., Henry, T. D., Egnaczyk, G., & Shreenivas, S. (2021). Artificial Intelligence Can Improve Patient Management at the Time of a Pandemic: The Role of Voice Technology. Journal of medical Internet research, 23(5), e22959. https://doi.org/10.2196/22959
[13] Pereira, J., & Díaz, Ó. (2019). Using Health Chatbots for Behavior Change: A Mapping Study. Journal of Medical Systems, 43(5). https://doi.org/10.1007/s10916-019-1237-1
[14] Devaram, S. (2020). Empathic chatbot: Emotional intelligence for empathic chatbot: Emotional intelligence for mental health well-being. arXiv preprint arXiv:2012.09130.
[15] Fan, M., Li, Y., & Truong, K. N. (2020). Automatic detection of usability problem encounters in think-aloud sessions. ACM Transactions on Interactive Intelligent Systems, 10(2), 1–24. https://doi.org/10.1145/3385732
[16] Truong, K.P., Neerincx, M.A., & Leeuwen, D.A. (2008). Measuring spontaneous vocal and facial emotion expressions in real world environments.
[17] Young, K. (2009). Direct from the source: the value of ‘think-aloud’ data in understanding learning. The Journal of Educational Enquiry, 6.
[18] Sykownik, P., Born, F., & Masuch, M. (2019). Can you hear the player Experienceƒ A pipeline for automated sentiment analysis of player speech. 2019 IEEE Conference on Games (CoG). https://doi.org/10.1109/cig.2019.8848096
[19] Miclau, C., Peuker, V., Gailer, C., Panitz, A., & Müller, A. (2023). Increasing customer interaction of an online magazine for beauty and fashion articles within a media and Tech Company. HCI in Business, Government and Organizations, 401–420. https://doi.org/10.1007/978-3-031-35969-9_27
[20] Brickman, G. A.(1980). Uses of Voice-Pitch Analysis. Journal of Advertising Research, 20(2), 69-73.
[21] Klebba J. M. (1985). Physiological measures of research: a review of brain activity, electrodermal response, pupil dilation, and voice analysis methods and studies. Curr. Issues Res. Advert. 8 53–76.
[22] Motoki, K., Saito, T., Nouchi, R., Kawashima, R., & Sugiura, M. (2019). A Sweet Voice: The Influence of Cross-Modal Correspondences Between Taste and Vocal Pitch on Advertising Effectiveness. Multisensory Research, 32(4-5), 401-427. https://doi.org/10.1163/22134808-20191365