What is data quality and why it matters
How to manage data quality. Human behavior research is very data intensive in nature. Data is front and center in any research study, and it can be collected with a number of different sensors with high sample rates in multimodal experiments, and subsequently combined with qualitative data from participant observation as well as with audio/video data from participant recordings. With all that accumulated data, it is not surprising that an average iMotions study easily generates several tens or even hundreds of gigabytes of data.
While a dataset of this size can pose its own challenges, a key question that researchers should keep in mind regards the quality of the data they collect. There is no obvious way to define what good data quality is. Data quality literature proposed a context-dependent approach by saying that data should be accepted if it is “fit for use” (1,2).
Data quality is an essential aspect of any experiment and needs to be managed closely to ensure accurate and reliable results, as inaccurate or incomplete data can lead to incorrect conclusions and unreliable results.
The context determines the quality
An old adage in data science goes: “garbage in, garbage out”, meaning that, if the data you work on is “bad”, then the results of your analysis are bound to be bad as well. While this is catchy, it’s also a simplification that hides very important parts of the story. First of all, we should define what we mean by garbage or bad data! The answer to this is far from obvious and can be highly context- and application-dependent. If you work with electroencephalography data (EEG) measuring brainwave activity and you want to prove the existence of a new evoked potential, your data quality bar will be so much higher than if you are using video data to study facial expressions. In the former case, the room for error is very small, while in the latter you are probably fine with some percentage of video frames with bad quality.
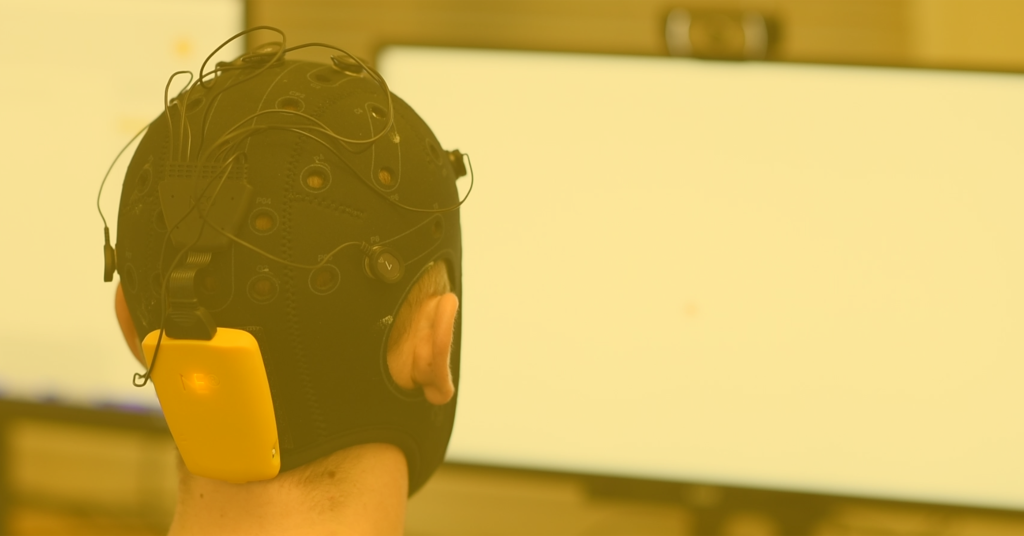
What matters is not aiming for perfect data quality, but for a level of data quality that matches the type of effect or research question that you want to address (3). With this in mind, considerations about data quality should be part of the research planning process, and should take into account at the very least the following possible issues related to data quality:
- Insufficient data: assuming that everything goes well with your experiment and you get all the planned data collected with no other issues, you have to ask yourself whether this amount is sufficient to perform your analysis as planned. It is important to keep in mind that different data modeling techniques and statistical methods require different amounts of data in order to prove or disprove the existence of the effect that is being studied. If we use the EEG example we mentioned earlier, an experiment with only 3-4 repetitions of the stimulus on a few subjects will most likely lead to an insufficient amount of data.
- Missing data: This can happen in a surprising number of ways. A loose electrode connection, a sensor’s Bluetooth connection that drops. The result? Data is missing from one or more participants in your study.
- Wrong data: the data you collect might be plagued by systematic errors if for instance a sensor was set up incorrectly, or maybe some miscommunication with the data collection team caused an electrode to be placed at the wrong location.
- “Dirty” data: this is a very broad category. Dirty or noisy data can be caused by excessive artifacts or noise from various sources, resulting in less information in the collected signals.
The take-home message here is the specifics of your experiment and your application determine the required level of data quality, and these should be central points when planning an experiment so that you can set the right expectations as to data quality.
How to manage data quality in iMotions
The iMotions software provides a number of tools to streamline the data collection process and ensure the best possible data quality.
An ounce of planning is worth a pound of data cleaning! Planning thoroughly is one of the best ways to boost data quality. Best practices include:
- Define a clear protocol for data collection, and make sure that every person involved in the experiment understands it fully.
- Plan a pilot phase before the actual experiment. During the pilot phase, you should run all the steps exactly as if you were running the real experiment, with the only difference being that your participants will not be the real ones. The purpose is to make sure that your data collection plan works in practice and that nothing was missed in the protocol. You also get a first impression of the data from the pilot participants, which can set the expectations for the data you will obtain in the actual experiment. If the pilot phase reveals some issues, don’t hesitate to implement solutions in the protocol and iterate with a new pilot phase.
- Check that all the equipment is there and functioning in advance. Doing a final test run the day before your experiment is a very good idea, and after the test succeeds, leave the equipment as it is and lock the door! Last-minute changes are the enemy of reliable data.
- Instruct your participants properly. Remember that in most cases your participants don’t know anything about biometrics – it is your task to ensure that they understand how the experiment works and what they are supposed to do. If you’re running an online study and using a respondent panel to get your participants, it’s also worth considering choosing a panel service that can provide participants that fit your requirements (4).
- Use data quality metrics to check the data you collect. iMotions provides a number of ways to verify the quality of your data. For instance, if you use EDA data, you can use our signal-to-noise ratio analysis, which reveals how well your collected signal falls in the expected frequency range. Other checks verify that the collected data has the expected sample rate. We are always working to add new ways to check data quality in order to make human behavior research more accessible to all our users.
After you are done with data collection, you will typically go through a pre-processing phase, as the initial step of your data analysis. This can include, for instance, excluding participants or stimuli that gave data with a quality lower than your set threshold. Data cleaning can also be a part of this, for example filtering the data from certain types of noise that can be removed.
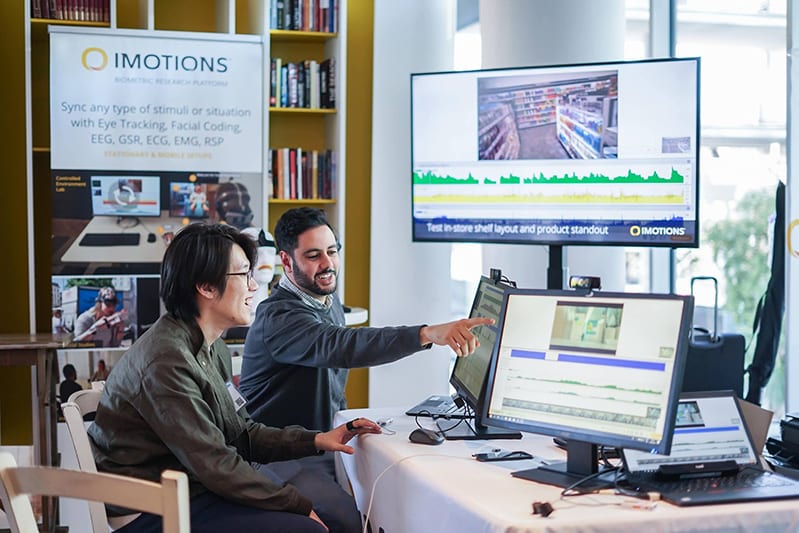
Running a research project is a very challenging task that requires a large investment of time and resources. Ending up with data that is not fit for purpose because of bad data quality after all the work can be a very frustrating experience for everyone involved. Therefore it’s very important to do proper planning and ensure that best practices are followed all the way through. iMotions supports you through your research work, both with our software that helps you identify potential issues with your data, and with our team which is always ready to answer any questions you might have. With this approach, we’re certain that your research project will be successful, and if you are interested in how we support our customers and what you can expect from being an iMotions customer, reach out to us through the link below.
Let’s talk!
We love to learn about the research projects that you are working on and helping you out with achieving your goals. Get a free demo of our software, get advice on hardware purchases or learn about our enablement services that teaches you the skills needed for conducting biometrics research.
References
- Wang, R. Y., & Strong, D. M. (1996). Beyond accuracy: What data quality means to data consumers. Journal of management information systems, 12(4), 5-33. link.
- Cai, L., & Zhu, Y. (2015). The challenges of data quality and data quality assessment in the big data era. Data science journal, 14. link.
- Haug, A., Zachariassen, F., & Van Liempd, D. (2011). The costs of poor data quality. Journal of Industrial Engineering and Management (JIEM), 4(2), 168-193. link.
- Eyal, P., David, R., Andrew, G., Zak, E., & Ekaterina, D. (2021). Data quality of platforms and panels for online behavioral research. Behavior Research Methods, 1-20. link.