Gaze estimation discerns where one is looking, critical for eye-tracking in fields like psychology, marketing, and HCI. It uses eye anatomy and movements to interpret cognitive states and intentions from visual patterns, requiring precise measurement for reliable insights. Innovations in non-invasive and invasive techniques continue to advance this technology, expanding its applications and importance.
Table of Contents
Introduction
Gaze estimation, in essence, refers to the process of determining where a person is looking — a point of regard on a visual stimulus, whether it be a computer screen, an artwork, or the horizon. In a world where technologies evolve ceaselessly to decipher the unspoken nuances of human behavior, gaze estimation stands out as a pivotal methodology for one of the most prevalent biometric instruments, namely eye tracking. The subtle yet profound ability to discern the focus of one’s visual attention is at the core of eye tracking research, weaving a tapestry of applications that span across diverse fields such as psychology, marketing, healthcare, and human-computer interaction.
Eye tracking, the practice of measuring either the point of gaze or the movement of the eyes relative to the head, is intrinsically bound to accurate gaze estimation. The eyes, often referred to as the “windows to the soul,” offer a gateway into internal cognitive processes, emotional states, and individual intentions . By understanding where and how the eyes move, researchers and professionals can glean insights into patterns of visual attention, information processing, and even physiological conditions.
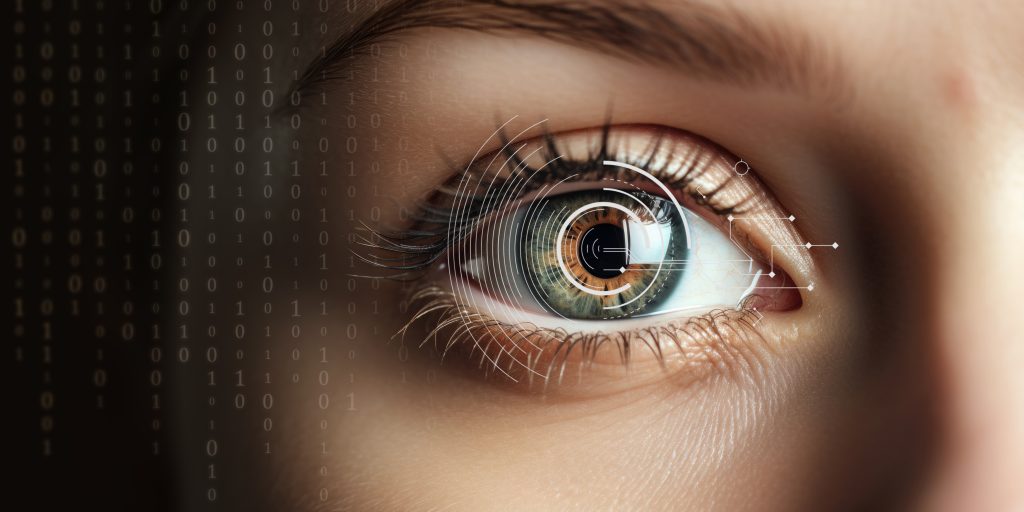
Gaze estimation is crucial for transforming raw eye tracking data into meaningful insights. The precision with which we can estimate gaze directly influences the quality and reliability of eye tracking results. From understanding a user’s interaction with a website or application to unraveling the cognitive underpinnings of reading disorders, gaze estimation is the key that holds the potential to unlock a myriad of applications.
The importance of gaze estimation is underscored by its potential to render interactions more intuitive, create experiences that are more engaging, and develop solutions that are profoundly personalized. As we venture further into an era marked by technological advancements, the exploration of gaze estimation within eye tracking research becomes not just important but indispensable.
This article aims to delve into the intricacies of gaze estimation, exploring its methodologies, applications, and its indispensable role in shaping the future of eye tracking research. Through this journey, we aim to illuminate how the silent gaze can indeed speak volumes, bridging the gap between observation and understanding.
Eye Anatomy and Physiology
Gaze estimation, a cornerstone of eye tracking research, hinges on a comprehensive understanding of the physiology of the human eye. This dynamic organ is far more than a passive receptor of light; it plays an active role in vision, which is crucial for devising accurate tracking methodologies.
Anatomy and Eye Movements: The Pillars of Gaze Estimation
The eye’s anatomy is intricate, with each component playing a pivotal role in gaze estimation. The cornea and lens are instrumental in refracting light to form images on the retina, with their curvature and clarity impacting the quality of reflected images used in tracking. The pupil, acting as the eye’s aperture, adjusts its size in response to light intensity and emotional states, influencing pupil-based gaze estimation techniques. The iris, controlling the pupil size, contributes to the visibility and contrast of the pupil, crucial for accurate tracking.
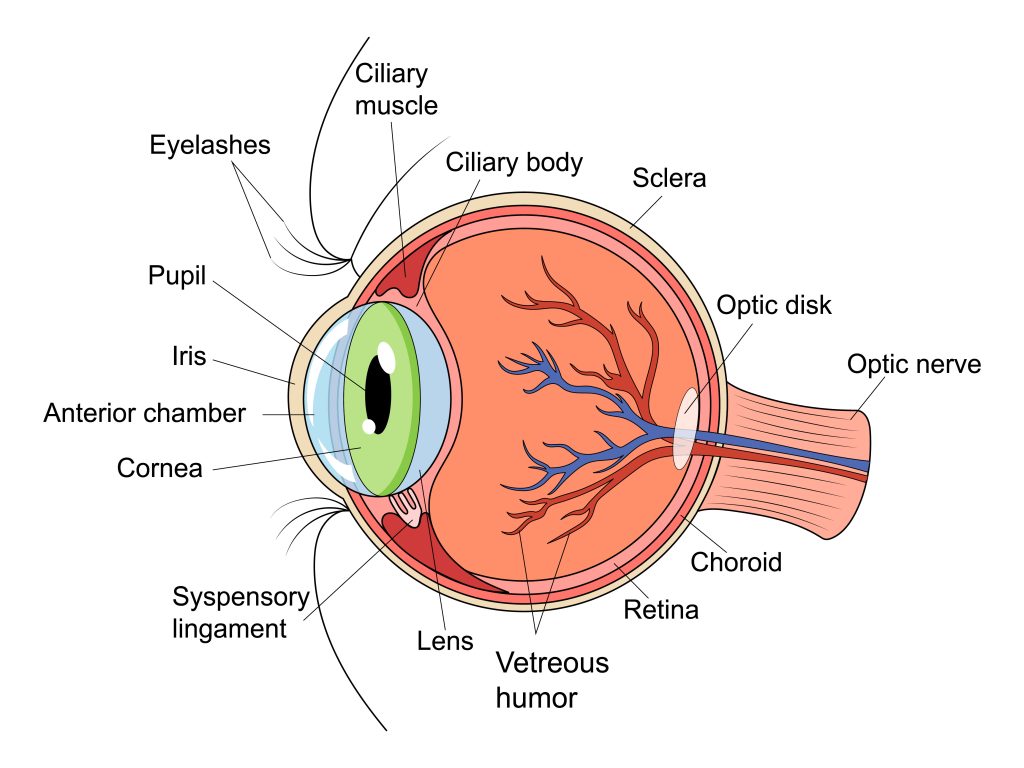
The retina is a complex layer of photoreceptive cells that convert light into electrical signals. A small depression in the retina, known as the fovea, is densely populated with cones and is the point of sharpest vision. The optic nerve, though not directly involved in gaze estimation, plays a vital role in transmitting visual information to the brain.
Eye movements also have profound implications for gaze estimation. Saccades, rapid eye movements adjusting the line of sight, and fixations, moments when the eye is still, offer insights into cognitive processes and attention. The duration of fixations can be indicative of cognitive engagement. Smooth pursuit movements, which are slower and track moving objects, can be influenced by factors such as age and neurological conditions.
Physiological Signals and External Factors
Monitoring physiological signals, such as pupil dilation and blinks, is vital for gaze estimation. Changes in pupil size can indicate cognitive load and emotional arousal, while the frequency and duration of blinks can be indicative of fatigue. Microsaccades, tiny jerk-like movements during fixations, introduce noise into gaze data but also hint at attentional focus.
External factors, such as ambient lighting, can also influence eye physiology and, consequently, gaze estimation. The level of ambient light affects the pupil size and the quality of reflections used in tracking.
Inter-individual Variability and Health Considerations
Inter-individual variability, including differences in eye shape, size, and refractive errors (like myopia and astigmatism), can affect the accuracy of gaze estimation. Additionally, age-related changes and health conditions, such as dry eyes or neurological disorders, introduce variability that must be considered to ensure precise gaze tracking.
Gaze Estimation Techniques
There are two main types of gaze estimation techniques: Non-invasive and invasive. Invasive techniques involve attaching sensors to the eye or head, while non-invasive techniques do not require any physical contact with the user.
Non-Invasive Gaze Estimation Techniques
- Pupil-Corneal Reflection Method:
- Description: Utilizes a camera and light source to track the reflection on the cornea and the center of the pupil.
- Applications: Commonly used in eye tracking devices for research and usability testing.
- Appearance-Based Gaze Estimation:
- Description: Employs machine learning, often deep learning, to estimate gaze direction based on the appearance of the eye region.
- Applications: Used in real-time applications and virtual reality environments.
- Infrared Oculography (IROG):
- Description: Uses infrared light to track eye movements by contrasting the retina’s absorption against the sclera’s reflection.
- Applications: Used in dark environments or for tracking eye movements in sleep studies.
- Electrooculography (EOG):
- Description: Measures the corneo-retinal standing potential of the eyes, allowing for the estimation of gaze direction.
- Applications: Useful in sleep studies and assistive technologies.
- Eye Movement Tracking in Video:
- Description: Analyzes video footage to track eye movements and estimate gaze direction.
- Applications: Utilized in human-computer interaction and behavioral research.
- Remote Eye Tracking:
- Description: Uses specialized cameras placed at a distance to track eye movements without any contact with the participant.
- Applications: Used in large-scale or naturalistic settings.
- Gaze Estimation using Wearables:
- Description: Utilizes smart glasses or similar wearable devices equipped with cameras to estimate gaze direction.
- Applications: Real-world and on-the-go gaze tracking.
- Single Point Calibration Techniques:
- Description: Requires the participant to focus on a single point for calibration before estimating gaze direction.
- Applications: Useful for quick setups in research and gaming.
Invasive Gaze Estimation Techniques (primarily used in primate research)
- Implanted Coil Technique:
- Description: Involves surgically implanting a coil in the eye to track eye movements using magnetic fields.
- Applications: Primarily used in animal research due to its invasive nature.
- Intracranial Electroencephalography (iEEG) with Gaze Estimation:
- Description: Combines intracranial EEG data with eye tracking to understand neural activity related to gaze movements.
- Applications: Used in advanced neuroscience research.
- Microelectrode Recordings:
- Description: Involves implanting microelectrodes in regions of the brain responsible for visual processing to study gaze.
- Applications: Often used in primate studies and some clinical cases.
- Invasive Optogenetics with Gaze Estimation:
- Description: Combines genetic modification with light to control and monitor neurons related to eye movement.
- Applications: Primarily used in animal research.
- Subdural Electrode Recordings:
- Description: Places electrodes on the surface of the brain to study the relationship between neural activity and gaze direction.
- Applications: Used in clinical settings for patients undergoing monitoring for epilepsy treatment.
Both invasive and non-invasive gaze estimation techniques offer unique advantages and applications. While non-invasive methods are more commonly used due to their ease and ethical considerations, invasive methods allow for deeper insights into neural mechanisms.
How to calculate gaze estimation (with non-invasive methods)
Calculating gaze estimation involves determining the point of regard or where a person is looking on a visual stimulus based on the features of their eyes. Several methods and techniques have been developed to calculate gaze estimation, ranging from geometric models to machine learning approaches.
Geometric Models:
a. Pupil-Corneal Reflection Method:
- Step 1: Shine an infrared light towards the eye and capture the image using an eye tracking camera.
- Step 2: Identify the center of the pupil and the reflection of the infrared light on the cornea.
- Step 3: Calculate the vector between these two points.
- Step 4: Using known parameters like the distance between the camera and the eye, and the geometry of the eye, calculate the gaze point on the screen.
b. 3D Model-Based Methods:
- Step 1: Construct a 3D model of the eye, including the cornea, pupil, and lens.
- Step 2: Extract features such as the center of the pupil from the image.
- Step 3: Map the 2D features onto the 3D model to determine the line of sight.
- Step 4: Calculate the intersection of the line of sight with the display to find the gaze point.
Appearance-Based Methods:
a. Machine Learning Models:
- Step 1: Extract features such as eye images, head pose, and pupil location.
- Step 2: Train a machine learning model (e.g., Support Vector Machines, Neural Networks) using labeled data that associates the extracted features with known gaze points.
- Step 3: Use the trained model to predict the gaze point based on new input data.
b. Deep Learning Models:
- Step 1: Feed eye images directly to a deep learning model, such as a Convolutional Neural Network (CNN).
- Step 2: The model learns to directly predict the gaze point from the eye image, without explicit feature extraction.
- Step 3: With sufficient training data, the model can generalize to accurately predict gaze points in different scenarios.
Hybrid Methods:
a. Combining Geometric and Appearance-Based Methods:
- Step 1: Utilize both geometric information (like the position of the pupil) and appearance information (like the overall eye image).
- Step 2: Fuse these data points to improve the accuracy and robustness of gaze estimation.
Calibration:
Regardless of the method used, calibration is often necessary to improve accuracy. This involves having the user look at known points on a screen and adjusting the parameters of the gaze estimation model accordingly.
Deep Learning and Gaze Estimation
Deep learning has revolutionized the field of gaze estimation in recent years. Deep learning models are able to learn complex relationships between the input image and the gaze direction, resulting in accurate and robust gaze estimates.
Deep learning, a subset of machine learning, leverages neural networks to automatically learn and improve from experience, without being explicitly programmed. This paradigm is particularly suitable for gaze estimation, which involves predicting the point of regard on a stimulus based on eye-related features.
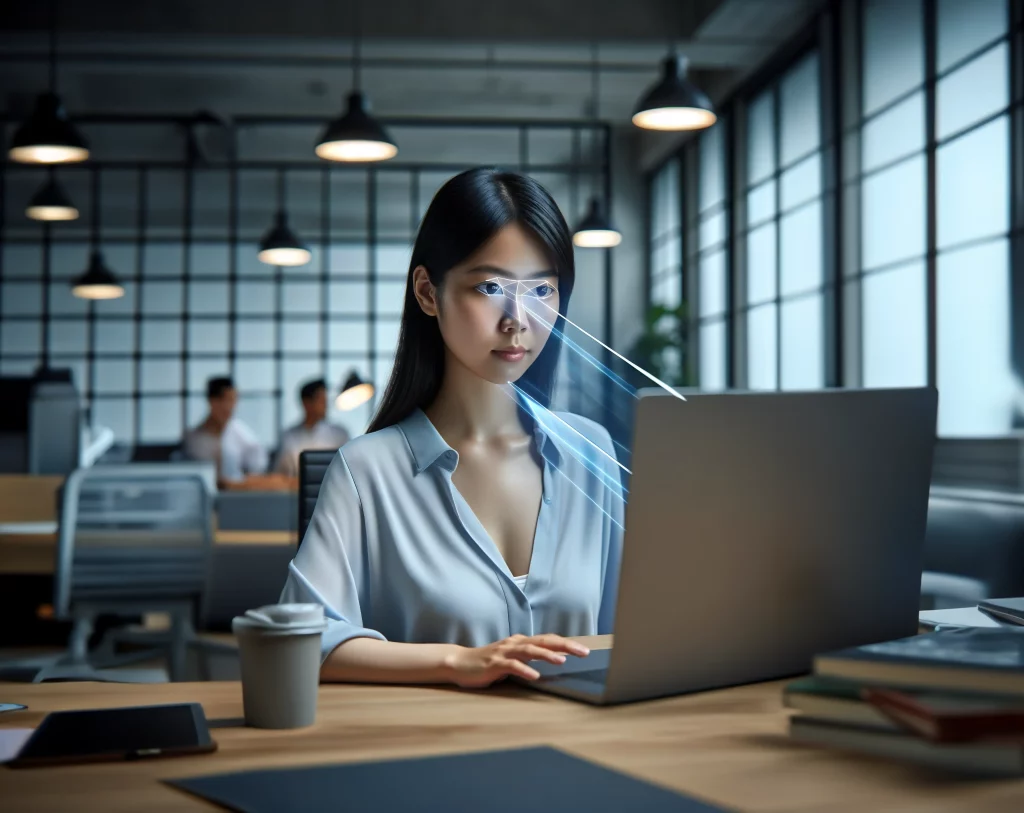
Traditional gaze estimation techniques sometimes require extensive calibration and might not generalize well across different environments and individuals. In contrast, deep learning-based methods harness the power of large datasets to improve their accuracy and robustness. These methods often employ Convolutional Neural Networks (CNNs), a class of deep neural networks adept at analyzing visual imagery.
CNNs can automatically extract hierarchical features from raw images of the eye region, thereby simplifying the gaze estimation problem. For instance, a CNN can learn to recognize patterns corresponding to the pupil’s position and shape, eyelid state, and eye corner locations, which are then used to estimate the gaze direction.
One of the key advantages of using deep learning for gaze estimation is its potential to reduce the need for extensive calibration. Some models can be trained on data from multiple individuals, enabling them to generalize across different users and environments. Moreover, these methods can adaptively learn from new data, continuously improving their estimation accuracy.
Deep learning is revolutionizing gaze estimation by automating feature extraction and reducing calibration requirements. By employing sophisticated neural networks, researchers and practitioners can develop more accurate, user-friendly, and adaptive gaze estimation systems, ultimately advancing fields such as human-computer interaction, psychology, and assistive technology.
Applications of Gaze Estimation
Gaze estimation, and by extension eye tracking, finds applications across various disciplines, bringing in new dimensions of interaction, analysis, and understanding. Here is a comprehensive list of application areas:
Human-Computer Interaction (HCI):
- Gaze-Based Control: Enabling hands-free navigation and control of digital interfaces.
- Virtual Keyboards: Assisting individuals with physical disabilities in typing or communicating.
- Gaze-Aware Systems: Dynamically adapting content based on where a user is looking.
Healthcare and Assistive Technologies:
- Augmentative and Alternative Communication (AAC): Helping individuals with severe speech and motor impairments communicate.
- Neurological Disorder Assessment: Analyzing gaze patterns for early diagnosis of disorders such as Autism, ADHD, and Parkinson’s.
- Rehabilitation: Using gaze-controlled games and activities for motor rehabilitation.
Psychology and Cognitive Science:
- Attention and Perception Studies: Understanding cognitive processes by analyzing gaze patterns.
- Reading and Language Research: Studying reading patterns, language comprehension, and dyslexia.
- Usability Testing: Evaluating user engagement and experience with products or interfaces.
Marketing and Advertising:
- Consumer Engagement Studies: Analyzing which areas of advertisements, websites, or store shelves attract attention.
- Consumer Behavior Studies: Evaluating product packaging and presentation effectiveness.
Automotive and Transportation:
- Driver Monitoring Systems: Detecting drowsiness, distraction, and ensuring driver attention for safe driving.
- In-Car Infotainment Control: Allowing drivers to interact with in-car systems using eye movements.
Gaming and Virtual Reality (VR):
- Immersive Gaming: Enhancing gaming experiences by incorporating gaze-based interactions.
- VR Navigation: Enabling gaze-driven navigation and interaction in virtual environments.
Education and Learning:
- Adaptive Learning Systems: Customizing content delivery based on a student’s focus and interest.
- Learning Disabilities Diagnosis: Identifying learning difficulties through gaze pattern analysis.
Entertainment and Media:
- Interactive Art Installations: Creating dynamic art that responds to viewers’ gaze.
- Movie and Content Testing: Evaluating viewers’ engagement and interest in media content.
Aerospace and Defense:
- Pilot Training: Monitoring gaze to evaluate situational awareness and decision-making during simulations.
- Remote Drone Control: Using gaze to enhance control interfaces for drones.
Social Robotics and Humanoid Systems:
- Robot-Human Interaction: Enhancing the naturalness of interactions between humans and robots through gaze-aware responses.
- Gesture Recognition: Incorporating gaze data to improve context understanding.
Sports and Performance Training:
- Skill Assessment: Analyzing gaze patterns to evaluate and improve athletes’ performances.
- Concentration Training: Utilizing eye tracking to enhance focus and concentration skills.
Conclusion
The human eye, with its complex anatomy and physiology, provides the foundation upon which gaze estimation is built. Through the exploration of corneal reflections, pupil dynamics, and eye movements, scientists have developed an array of methods to ascertain the focal point of a subject’s gaze with increasing accuracy.
Advancements in computational models, particularly the integration of machine learning and computer vision, have significantly enhanced the precision of gaze estimation. These developments facilitate a more granular understanding of visual attention and by extension, human cognition and behavior. The science of gaze estimation is integral to the progression of disciplines ranging from psychology to consumer behavior, offering insights that were once unattainable.
The implications for practical application are extensive. In user interface design, gaze estimation enables the creation of adaptive systems that respond to visual attention cues. In accessibility, it offers novel ways to interact with technology for individuals with motor impairments. In healthcare, it has the potential to aid in the diagnosis and monitoring of various conditions.
As gaze estimation technologies become more advanced, the accuracy and reliability of eye tracking data improve. This enhancement is critical for the development of systems and applications that rely on high-fidelity interpretation of gaze patterns. However, the methodology must also contend with challenges such as individual variability in eye physiology and external factors like lighting conditions.
In summary, gaze estimation is a practice marked by its detailed scrutiny of ocular behavior, translating the subtle movements of the eye into discernible data. The advancements in this field promise to refine the symbiosis between humans and technology, ensuring that as we look towards the future, our gaze is met with understanding. The trajectory of gaze estimation suggests a landscape of untapped potential, waiting to be explored as we continue to innovate and integrate eye tracking into various aspects of life and work.
Eye Tracking
The Complete Pocket Guide
- 32 pages of comprehensive eye tracking material
- Valuable eye tracking research insights (with examples)
- Learn how to take your research to the next level
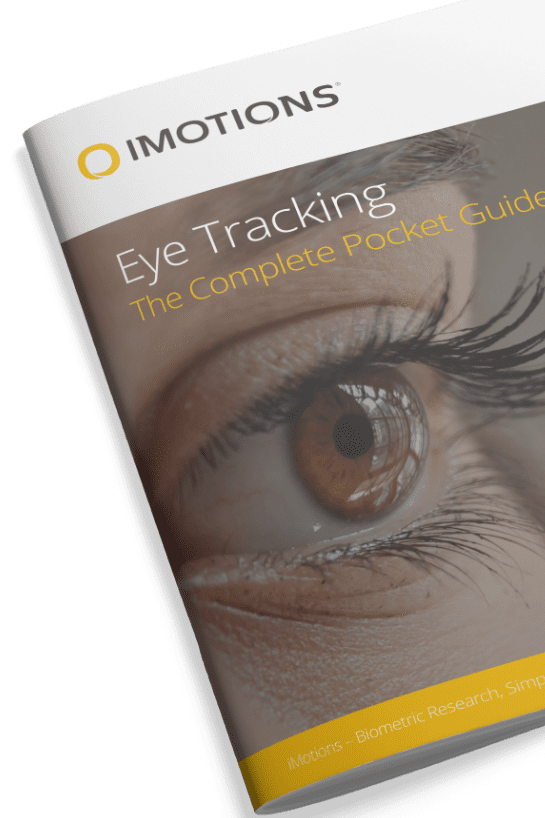
Eye Tracking Glasses
The Complete Pocket Guide
- 35 pages of comprehensive eye tracking material
- Technical overview of hardware
- Learn how to take your research to the next level
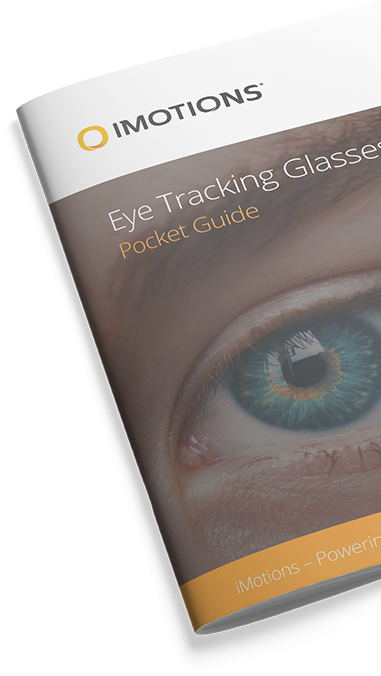