Discover the essentials of survey data analysis with iMotions’ comprehensive guide. Learn how to prepare, analyze, and interpret survey results for meaningful insights. From basics to advanced techniques, enhance your research with our step-by-step approach. Perfect for anyone looking to make informed decisions from survey data.
Surveys are a common research tool used to collect data and gather information from a targeted audience. Analyzing survey data is a crucial step in extracting meaningful insights and making informed decisions. In this comprehensive guide, we will explore the various aspects of survey data analysis, from understanding the basics to interpreting the results. So, let’s dive in!
Table of Contents
Understanding the Basics of Survey Data
Defining Survey Data
Survey data refers to the information collected through surveys, which are structured questionnaires designed to gather specific data from a sample population. This data can include demographic information, opinions, preferences, and more. By analyzing survey data, researchers can identify patterns, trends, and relationships within the data set.
When conducting a survey, it is important to carefully design the questionnaire to ensure that the data collected is relevant and reliable. The questions should be clear and concise, avoiding any ambiguity that could lead to misinterpretation. Additionally, the sample population should be representative of the target audience to ensure the survey results accurately reflect the opinions and preferences of the intended group.
Survey data can be collected through various methods, including online surveys, phone interviews, mail surveys, and face-to-face interviews. Each method has its advantages and disadvantages, and researchers must consider factors such as cost, time, and accessibility when choosing the most appropriate method for their study.
Importance of Survey Data Analysis
The analysis of survey data plays a crucial role in transforming raw data into valuable insights. It helps researchers identify key findings, understand the target audience better, and make data-driven decisions. By analyzing survey data, organizations can improve their products, services, and overall customer experience.
Survey data analysis involves various techniques, such as descriptive statistics, inferential statistics, and data visualization. Descriptive statistics summarize and describe the main characteristics of the data, providing a clear overview of the survey results. Inferential statistics, on the other hand, allow researchers to draw conclusions and make predictions about the larger population based on the survey data.
Data visualization is another important aspect of survey data analysis. It involves presenting the survey results in a visual format, such as charts, graphs, and infographics, to enhance understanding and facilitate communication of the findings. Visual representations of data can make complex information more accessible and engaging, enabling stakeholders to grasp the key insights quickly.
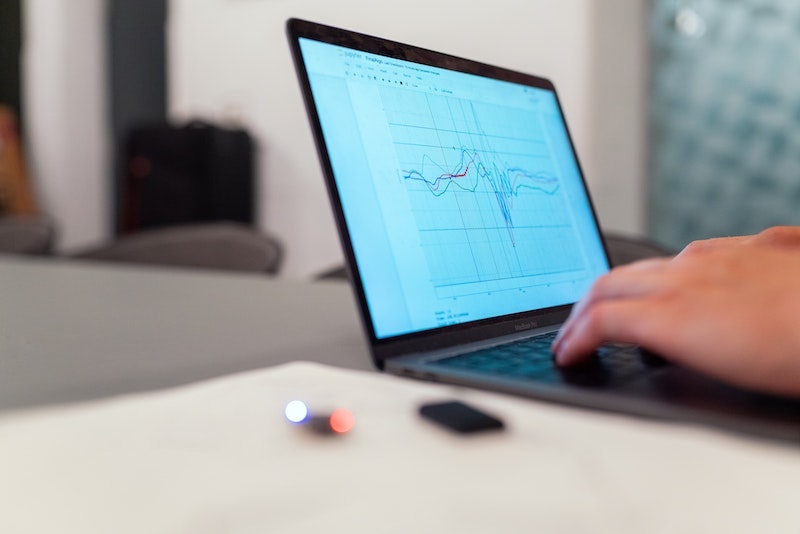
Furthermore, survey data analysis can uncover hidden patterns and correlations within the data set. By examining the relationships between different variables, researchers can gain a deeper understanding of the factors that influence people’s opinions, behaviors, and preferences. This knowledge can be invaluable for organizations seeking to improve their marketing strategies, product development, and customer satisfaction.
In conclusion, survey data analysis is a powerful tool that allows researchers to extract meaningful insights from raw data. It enables organizations to make informed decisions, understand their target audience better, and drive positive change. By employing effective survey design and utilizing appropriate analysis techniques, researchers can unlock the full potential of survey data and harness its value for various purposes.
Preparing Your Survey Data for Analysis
When it comes to analyzing survey data, there are a few crucial steps that need to be taken to ensure accurate and reliable results. One of the first steps in this process is cleaning your data. Cleaning involves removing any errors, inconsistencies, or incomplete responses that may have been collected during the survey. By doing this, you can ensure that your data set is free from any potential biases or inaccuracies that could skew your results.
Once you have cleaned your data, the next step is to organize it in a way that is conducive to analysis. This step, known as data organization, involves structuring your data in a way that aligns with your analysis goals. Depending on the nature of your data, this could involve grouping responses, creating variables, and categorizing data into relevant categories.
Grouping responses is a common practice in data analysis, as it allows you to identify patterns and trends within your data set. By grouping similar responses together, you can gain a better understanding of the overall sentiment or opinion of your survey participants. This can be particularly useful when analyzing open-ended questions, where participants have the freedom to provide detailed responses.
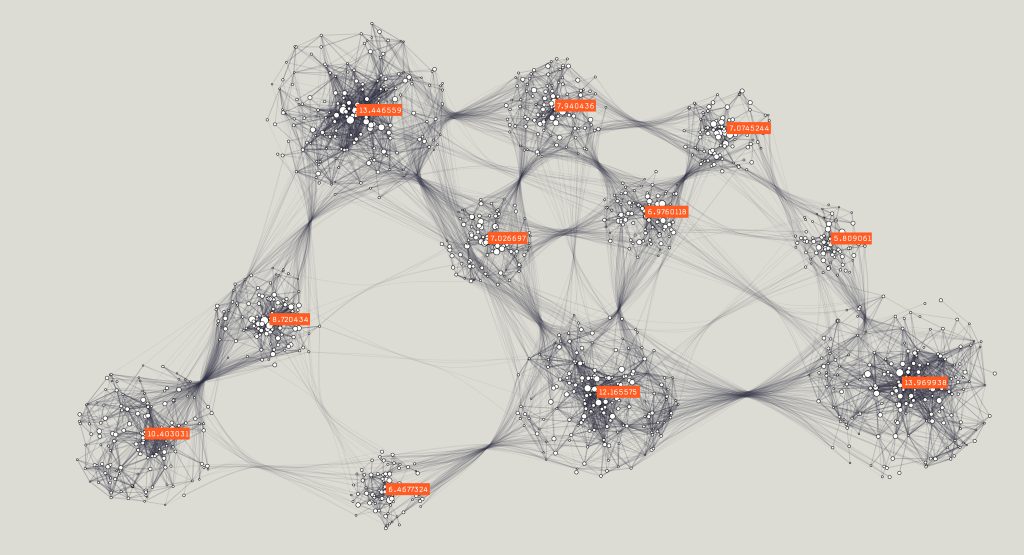
In addition to grouping responses, creating variables is another important aspect of data organization. Variables allow you to assign numerical values to different attributes or characteristics within your data set. This can be useful when conducting statistical analysis, as it enables you to quantify and compare different variables to identify any significant relationships or correlations.
Categorizing data into relevant categories is also crucial for effective data organization. By categorizing your data, you can create meaningful groups that can be easily compared and analyzed. This can be particularly useful when dealing with survey questions that have multiple response options, as it allows you to aggregate and summarize the data in a more meaningful way.
Overall, preparing your survey data for analysis involves more than just cleaning and organizing. It requires careful consideration of the specific goals and objectives of your analysis, as well as the nature of your data. By taking the time to properly prepare your data, you can ensure that your analysis is accurate, reliable, and ultimately, provides valuable insights.
Different Methods of Survey Data Analysis
When it comes to analyzing survey data, there are various methods that researchers can employ to gain valuable insights. Two commonly used methods are descriptive analysis and inferential analysis.
Descriptive Analysis
Descriptive analysis is an essential first step in survey data analysis. It involves summarizing and describing the main characteristics of the collected data. By calculating frequencies, percentages, averages, and other measures, researchers can gain a better understanding of the data’s basic features.
For example, let’s say you conducted a survey to gather information about people’s preferences for different types of music. Through descriptive analysis, you can determine the most popular genre, the average age of respondents, and the distribution of preferences across different age groups.
Descriptive analysis provides researchers with valuable insights into the survey findings. It helps in identifying patterns, trends, and relationships within the data. This method is particularly useful for exploratory research, where the goal is to gain a comprehensive understanding of the survey population.
Inferential Analysis
While descriptive analysis provides a snapshot of the survey data, inferential analysis takes it a step further by drawing conclusions and making predictions about a larger population based on a sample. This method involves statistical techniques that allow researchers to generalize findings beyond the sample surveyed.
For instance, let’s consider a scenario where you conducted a survey to determine the satisfaction levels of customers who recently purchased a particular product. Through inferential analysis, you can make predictions about the satisfaction levels of the entire customer population based on the responses of the sample surveyed.
Inferential analysis employs various statistical techniques, such as hypothesis testing, regression analysis, and confidence intervals. These methods help researchers assess the significance of relationships, test hypotheses, and estimate population parameters.
By using inferential analysis, researchers can make informed decisions and recommendations based on the survey findings. It allows them to draw meaningful conclusions that have implications beyond the specific sample surveyed.
Both descriptive analysis and inferential analysis are crucial methods in survey data analysis. Descriptive analysis provides a comprehensive overview of the data, while inferential analysis enables researchers to make predictions and draw conclusions about the larger population. By employing these methods, researchers can unlock valuable insights that can inform decision-making and drive future research.
Tools and Software for Survey Data Analysis
Overview of Data Analysis Tools
There are numerous tools available to aid in survey data analysis. These tools range from basic spreadsheets to advanced statistical software. Some popular data analysis tools include Microsoft Excel, SPSS, R, Python, MatLab, and E-Prime. Each tool has its own strengths and features, allowing researchers to choose one that best suits their analysis needs.
Choosing the Right Software for Your Needs
When selecting a data analysis software, it is important to consider factors such as the complexity of your analysis, the size of your data set, and your familiarity with the software. It’s also essential to ensure that the chosen software can handle the required statistical tests and visualization options. Taking these considerations into account will help you choose the right software for your survey data analysis.
Interpreting Survey Data Results
Making Sense of the Numbers
Once you have analyzed your survey data, it’s time to interpret the results. This involves examining the patterns, trends, and relationships in the data and extracting meaningful insights. It’s crucial to approach the data objectively and avoid making assumptions or drawing conclusions that are not supported by the evidence.
Translating Data into Actionable Insights
The ultimate goal of survey data analysis is to translate the findings into actionable insights. These insights can drive decision-making, inform marketing strategies, improve products or services, or guide future research. By effectively communicating the analyzed data and insights, you can ensure that the efforts put into survey data analysis have a tangible impact.
Analyzing survey data is a necessary process to extract meaningful insights and make data-driven decisions. By understanding the basics, properly preparing the data, utilizing different analysis methods, and employing the right tools, researchers can successfully interpret survey data results and translate them into actionable insights. So, take the time to analyze your survey data thoroughly and unlock its full potential.
Free 44-page Experimental Design Guide
For Beginners and Intermediates
- Introduction to experimental methods
- Respondent management with groups and populations
- How to set up stimulus selection and arrangement
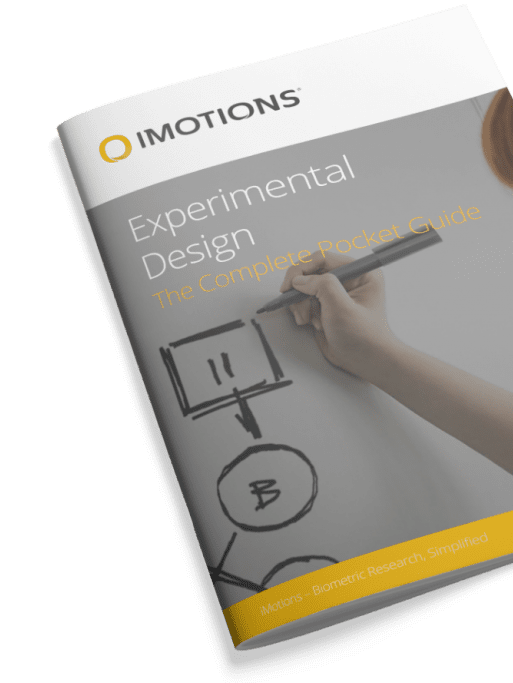