Failing to successfully exclude deceitful survey respondents could potentially set research, branding, and/or marketing endeavors on the wrong path to the detriment of time, cost, and effort. Researchers at the University of South Florida evaluated respondents’ facial expressions using iMotions to find signs of “bad actors.”
A Research Case
The rise of digitalization allows for more and more human behavior research to take place online. As a result, the multiplication of online surveys offers researchers new, much-needed avenues and tools to gain consumer insights. Conducting research is essential when launching a new brand, product, or service on the market – and many companies now use online surveys to accomplish this.
Since such a significant weight is put on especially online surveys, are they as foolproof and universally applicable as their popularity suggests? Professors Robert Hammond, Claudia Parvanta, and Rahel Zemen from the University of South Florida have taken this question under serious consideration in a recent article. In it, they discovered that compensated online surveys require a ‘blinking warning light,’ as you never know who is taking them, or do you?
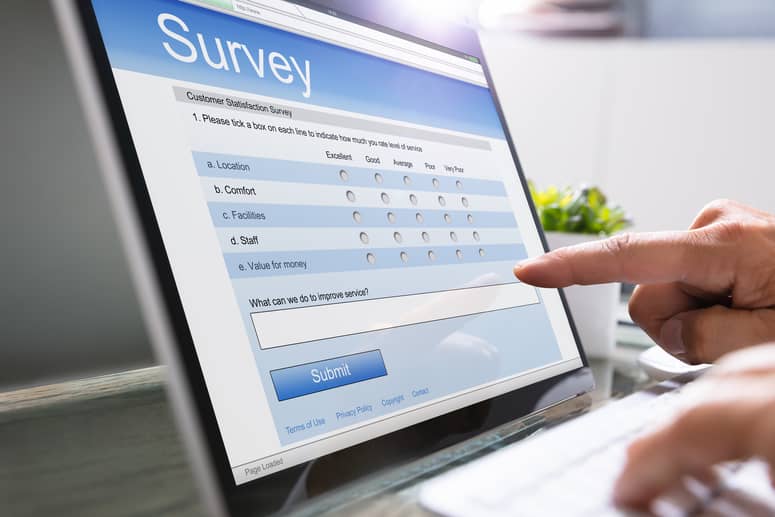
The group came upon the topic indirectly, as the unwelcome by-product of their study researching the effect of public service announcements (PSA) on tobacco quit intentions. They were given a grant from their state’s health department to be the first to try facial expression analysis as a way of evaluating viewer response to the PSAs. In the “side” study, they analyzed the effect of fraudulent data if it were included in the results, and through that research have provided us with valuable advice on how to obtain the highest data validity possible by studying facial expressions and attention to differentiate survey participants.
Weeding out “deceitful actors” is crucial aside from it being good practice. Failing to successfully exclude deceitful respondents could potentially set research, branding, and/or marketing endeavors on the wrong path to the detriment of time, cost, and effort. That is why having tools to identify and remove respondents who are ‘careless’ in their response or intentionally misrepresenting their eligibility to take the survey, is so important.
The “problem” with online survey validity
Thousands of honest individuals likely complete online surveys every day. It is therefore important to mention that the authors are not condemning all use of this method to gather input. But, when respondents are compensated directly by the researcher and survey links escape into the internet, there are fewer guard rails to prevent individuals, or even groups, from taking the survey for compensation. In a typical survey methodology, the researcher has to take it on faith that the person completing the study meets the criteria sought for the study. [There is a higher likelihood this is true when using vetted panels, as we discuss below.] In the case of the authors’ study, this was meant to be adults 18+ who lived in their state and used tobacco.
What prevented the authors from including hundreds of data points from individuals who in no way met those criteria was the use of facial expression analysis.
Research study – the perceived effectiveness of PSAs
As part of the research on Public Service Announcements, the team used a scheduled survey portion, which was completed by two groups. Firstly, it was sent to the “community”. These participants responded to an on-campus and neighborhood digital flyer and subsequently received an email, mentioning a 20 dollar compensation for participation in the form of a gift card. The study link from the community and neighborhood flyer was shared beyond the intended confines and was subsequently lost in the “wild” where several “bad actor” respondents got their hands on it, assumedly to obtain the monetary compensation. The second group of survey takers was recruited through a vetted panel from a commercial panel provider.
All respondents were recorded watching three different PSAs on the topic of tobacco usage and then asked to complete scaled questions to rate PSA effectiveness. Two main measurements were used to distinguish the difference of validity between the community and the vetted panel participants: attention and facial expressions. Both were analyzed using iMotions Software. Attention is a provided metric by iMotions (Affectiva) based on the head position (pitch, yaw, roll), and 20 Facial Action Units (FAU) are the output of iMotions (Affectiva).
The researchers then applied statistical measures to the output (i.e. regression). Moving features such as a lip curl, a smirk, or a cheek rise indicate the intentions of the participant and the focus he/she has. These measurements allowed participants to be classified into one of the three categories: deceitful, disinterested, and valid.
These three categories were defined by video analysis, and then subsequently the FAUs were analyzed to test the ability to predict the result. A participant classified as interested was paying attention, filled in all the attention checks correctly, and has valid results. The perfect participant! Disinterested participants lose focus, look away or take breaks during the study leading to invalid data. “Bad actor” participants could for instance replace their faces with a picture or switch off the lights in their rooms to avoid being seen.
Overall results
The results were as such: among the community, 58% of participants were deceitful and only 42% were valid. Among the panel participants, a high 87% of results were valid, 11% deceitful and 2% disinterested.
The community sample ended up being much smaller than the panel with a sample size of 92 as opposed to 409 for the panel.
What has the use of facial expression analysis taught us?
Using a camera to track participants’ facial expressions has helped the researchers identify more accurately which participants provide valid data and ones who do not. For instance, it appears that a participant who smirks in front of the camera during the experiment is 85% of the time associated with cheating and results in invalid data. It has also helped identify which participants who take longer than average than others are just slower, and which ones are distracted and give unauthentic answers. Facial expression analysis during online surveys might help get a cleaner data set and identify where false answers are coming from.
Where should I recruit my participants from an online survey?
There is of course no concise answer to the question of which participant recruitment method is “best”. It is up to the individual researchers or research teams to weigh the pros and cons of the mentioned participant recruitment channels, in light of what constraints on budget, time, etc, each researcher or team might face.
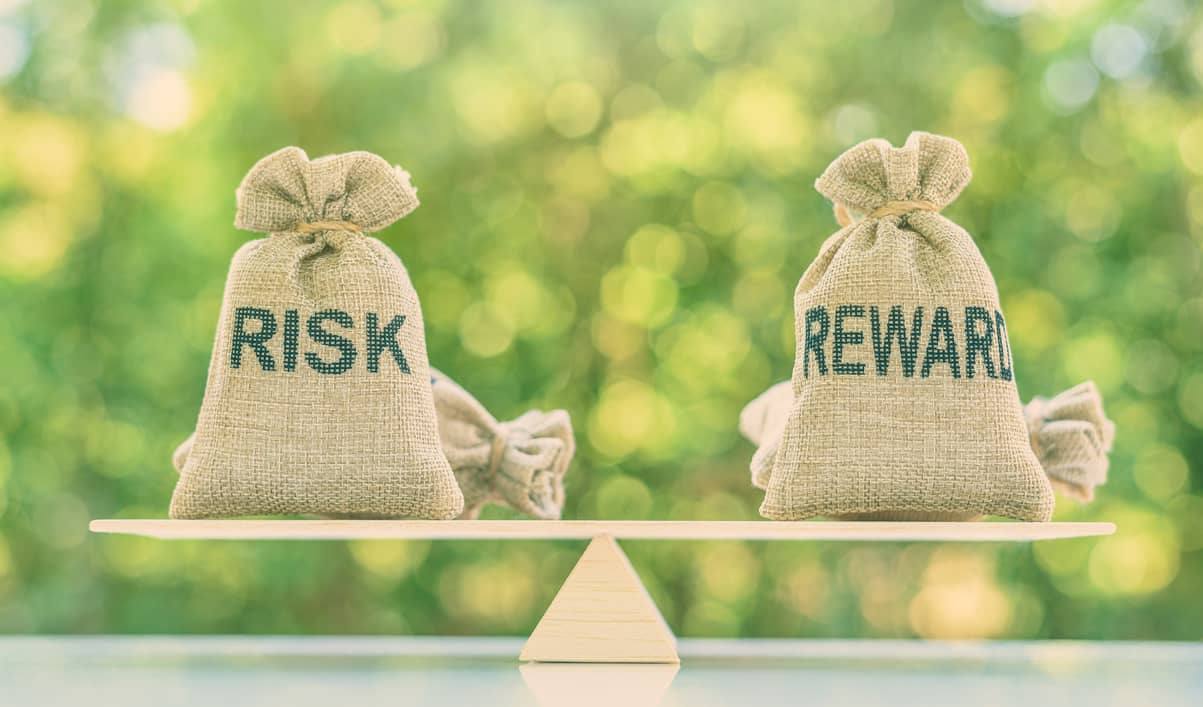
As mentioned above, when choosing the appropriate participant recruitment channel, has to be assessed on a case by case basis. Both recruitment channels, vetted and community have benefits to them. The vetted panels are usually considered to deliver more reliable data as the respondents are experienced in responding to surveys. Community recruitment is the faster and cheaper alternative and the hope could be to have higher engagement through people volunteering and receiving a cash incentive.
In turn, both channels also come with drawbacks that need to be taken into consideration. On the one hand, answers from the participants recruited from vetted panels have the risk of exhibiting lower attention which could then, in turn, mean that some responses have less authenticity. On the other hand, in regards to respondents recruited in the community channels, it can be more difficult to find a “controlled” sample as the compensated survey link leaked and many people filled it in who were not from the intended university and neighborhood sample. A reward of 20 dollars is quite attractive and might draw in participants with the wrong motivation, increasing the number of deceitful responses.
To learn more about this study and go in-depth with the statistics of their research and findings, you can access the entire article here.
Free 52-page Human Behavior Guide
For Beginners and Intermediates
- Get accessible and comprehensive walkthrough
- Valuable human behavior research insight
- Learn how to take your research to the next level
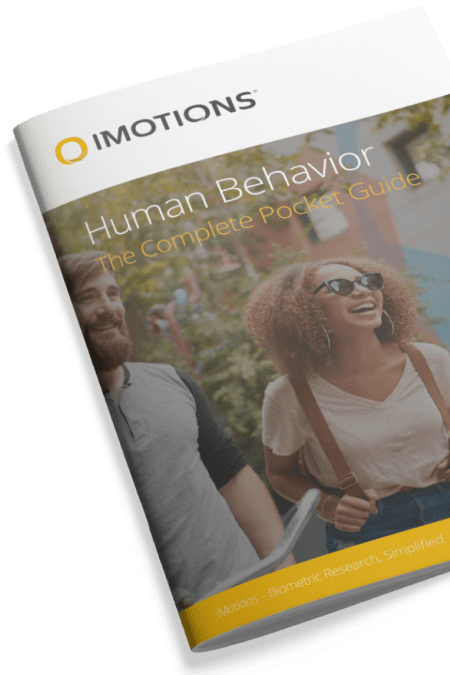