Imagine a world where cars safely and effortlessly connect people to diverse destinations, bridging the gaps between workplaces and bustling cities, ensuring an unrivaled fusion of safety, efficiency, and usability.
The efforts towards that scenario are continuously being deployed, considering key factors such as driving conditions, cognitive workload, and driving expertise.
The association between complex driving conditions and cognitive workload behind the wheel is a pressing determinant of the desired driver’s experience and, indeed, one of the foremost catalysts of traffic collisions. Cognitive workload, a construct that encompasses both the objective difficulty of a task and the user’s subjective perception of that difficulty, assumes particular significance in the realm of driving.
The objective difficulty depends on external factors like traffic load or inclement weather, which elevate the complexity of the driving experience. Simultaneously, a driver’s subjective experience of this workload often hinges on their comfort level and familiarity with handling such circumstances, manifesting it in different stress levels. Understanding the physiology of stress in this context represents a pivotal role in uncovering a rather intricate driver behavior, with the ultimate goal of curtailing the frequency of traffic accidents.
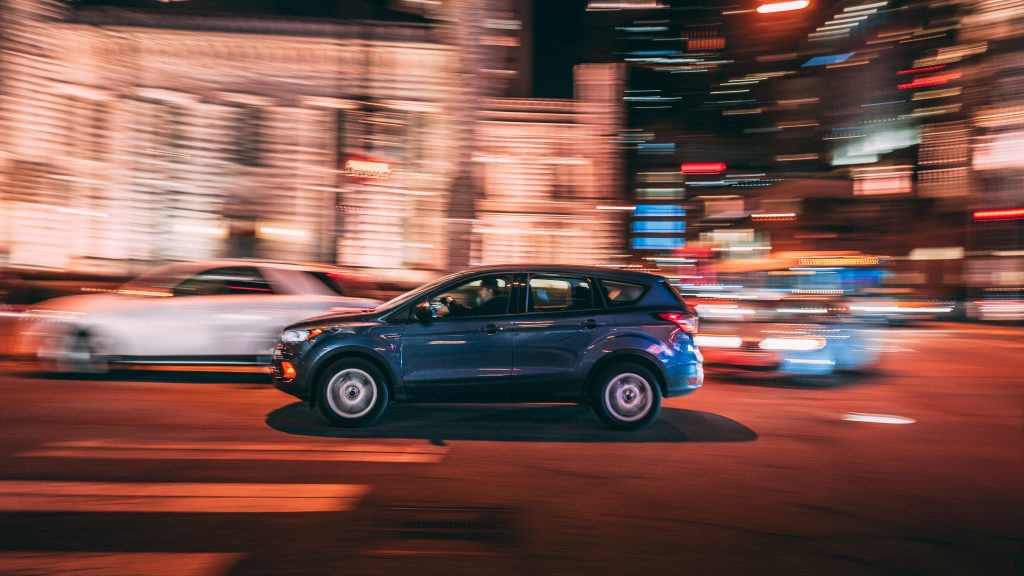
Human behavior research can help address some of these challenges, to make driving a more safe and comfortable experience. The field of Human-Computer Interaction (HCI) is perfectly situated to take on this challenge, as they routinely aim to develop robust designs and frameworks for actionable results with humans in focus.
An example of this kind of research was recently presented at the recent HCI international conference. This conference is a well-known international forum for researchers and companies in the space of user experience, user interface design, usability, interaction techniques, cognitive ergonomics, accessibility, human factors, and more. This year, at the 25th edition of the HCI international conference, iMotions presented the preliminary results from a multimodal study done in collaboration with the University of Padua (Italy) and VI-Grade. This study simultaneously evaluates visual attention, facial expression analysis, as well as electrocardiographic (ECG), respiratory (RSP), muscular (EMG), and electrodermal (EDA) activity in three different driving tasks with increased workload: low, medium, and high (Figure 1).
The study aimed to evaluate how different levels of workload can impact driver performance, with the results hopefully pointing toward ways in which driving can be performed more safely. Below, we provide an overview of the study – a true multimodal experiment into driver behavior.
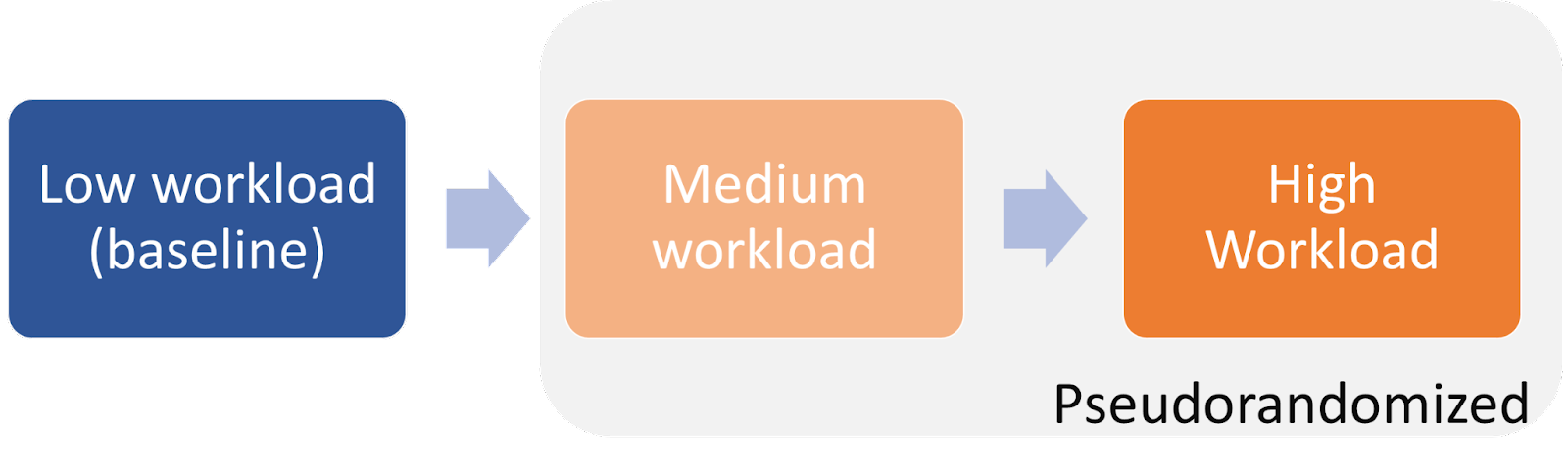
Figure 1: Diagram of the study. Drivers completed three driving tasks with low (baseline), medium and high workload.
The 3 driving tasks are:
Low workload (baseline): Drive on a free open highway, without traffic, doing double lane changes, maneuvers, acceleration and braking.
Medium workload: Slalom the car between traffic cones and perform a double lane change maneuver as fast as reasonably possible.
High workload: Drive through a city with random traffic conditions, traffic lights, multiple vehicles circulating in it, pedestrians crossing the street.
It was hypothesized that higher workload tasks induce higher visual demand (as indexed with dwell time %), higher heart rate and respiration rate as well as higher muscular and electrodermal activity.
Figure 2: Replay video of the high workload task in iMotions software suite integrating a multi-screen simulator with three Smart Eye Pro eye trackers, and biosensor responses from FEA, ECG, RSP, EMG and EDA.
The preliminary results of the present study show higher EMG activity, modulated arousal (heart rate and GSR peaks/min), and higher perceptual load (dwell time %) in complex driving scenarios (Figure 3).
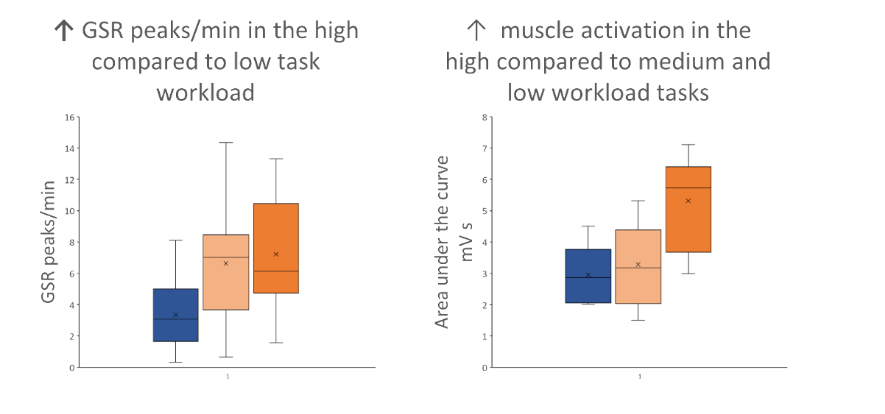
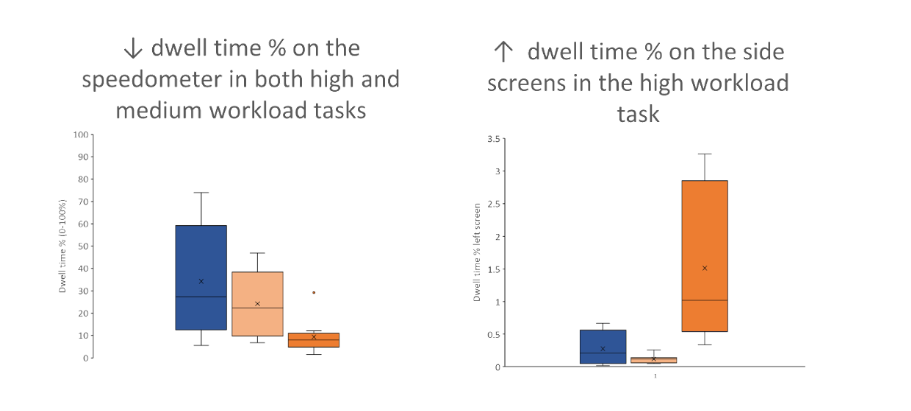
Figure 3: Electrodermal (EDA) and muscular (EMG) activity as well as dwell time % in the low, medium, and high workload tasks.
The results showed significant differences with EDA, EMG, and dwell time from eye tracking data. This indicated that these measures are suitable for understanding the cognitive workload for driving. As these measures are also related to the likelihood of an accident occurring, it may be beneficial for car manufacturers to seek ways in which such measures can be incorporated into vehicle design in order to provide early warning or assistive services from the car when needed.
This could ultimately provide an automated mechanism for reducing crash likelihood. Such a system wouldn’t require driver interaction as it would be triggered by the psychophysiological data points alone – crucial when the cognitive workload is self-evidently too high at that time. Such a system has recently been released by Smart Eye that can be built into vehicles for such detection.
For more details about this work, find the methods and results here.
Integrating these physiological measures with other data sources, such as vehicle performance metrics (throttle, brakes, gear) and environmental conditions, enables a comprehensive understanding of the driver’s behavior. This holistic approach can contribute to the development of advanced driver assistance systems (ADAS), enhancing safety and performance on the road.
Monitoring of these physiological signals can also be used in driver training and education programs. By providing drivers with personalized feedback on their stress levels, attentiveness, and focus, they can actively work on improving their driving skills, leading to safer roads and reduced accident rates.
References
- Healey, J.A., Picard, R.W.: Detecting stress during real-world driving tasks using physiological sensors. IEEE Transactions on Intelligent Transportation Systems 6(2), 156-166 (2005). doi: 10.1109/TITS.2005.848368.
- Zontone, P., Affanni, A., Bernardini, R., Piras, A., Rinaldo, R., Formaggia, F., Minen, D., Minen, M., Savorgnan, C.: Car Driver’s Sympathetic Reaction Detection Through Electrodermal Activity and Electrocardiogram Measurements”. IEEE Transactions on Biomedical engineering, vol. 49(12), 3413-3424 (2020). doi: 10.1109/TBME.2020.2987168
- Lemonnier, S., Bremond, R., Baccino, T.: Gaze behavior when approaching an intersection: Dwell time distribution and comparison with a quantitative prediction. Transportation Research Part F: Traffic Psychology and Behaviour 35, 60-74 (2015). doi: 10.1016/j.trf.2015.10.015
- Jabon, M., Bailenson, J., Pontikakis, E., Takayama, L., Nass, C.: Facial expression analysis for predicting unsafe driving behavior. IEEE Pervasive Comput 10(4), 84-95 (2011). doi: 10.1109/MPRV.2010.46
- Strayer, D.L., Drews, F.A., J
- Mehler B., Reimer, B., Coughlin, J.F., Dusek, J.A.: Impact of incremental increases in cognitive workload on physiological arousal and performance in young adult drivers. Transp Res Rec 2138(1), 6-12 (2009). doi: 10.3141/2138-02
- Collet, C., Clarion, A., Morel, M., Chapon, A., Petit, C.: Physiological and behavioral changes associated to the management of secondary tasks while driving. App Ergon 40(6), 1041-1046 (2009). doi: 10.1016/j.apergo.2009.01.007
- Wierwille, W.W., Eggemeier, F.T.: Recommendations for mental workload measurement in a test and evaluation environment. Hum Factors 35(2), 263-281 (1992). doi: 10.1177/001872089303500205