Discover the advancements in webcam eye tracking with iMotions’ WebET 3.0. Explore its affordability, remote accessibility, and improved accuracy for scalable research.
Table of Contents
Webcam-based eye tracking technology is experiencing rapid advancements, driven by cutting-edge computer vision algorithms. At the forefront of this innovation is iMotions’ latest webcam-based eye tracking algorithm, WebET 3.0, now integrated into all cloud-based applications, namely Remote Data Collection and iMotions Online. This development delivers new levels of accuracy and applicability, making eye tracking more accessible than ever.
Later in this article, we’ll explore just how accurate WebET 3.0 is, but first, let’s examine why webcam-based eye tracking has become so popular among researchers and developers.
Why Webcam-Based Eye Tracking Is Gaining Popularity
Webcam-based eye tracking uses advanced computer vision algorithms to monitor eye movements, offering insights into human behavior and cognitive processes. Its increasing adoption stems from its ease of use, affordability, and versatility, making it a game-changer for researchers, educators, and developers.
Remote Accessibility
One of the key reasons for its popularity is its ability to be used remotely. Unlike traditional systems requiring specialized hardware or dedicated labs, all that’s needed for webcam-based eye tracking is:
- A standard computer with a webcam.
- A stable internet connection.
This minimal setup makes the technology accessible to a broader audience, including smaller organizations, independent researchers, and educators who might not have the resources for more complex setups.
Affordability Without Compromise
Traditional eye-tracking systems are often expensive, limiting their usage to well-funded institutions or organizations. Webcam-based eye tracking offers a cost-effective alternative that doesn’t sacrifice quality.
- Affordable Entry Point: Perfect for small teams or individuals.
- Scalable Solutions: Suitable for large-scale projects or commercial applications.
With iMotions’ WebET 3.0, affordability meets precision, providing a solution that bridges the gap between high-quality tracking and budget-friendly access.
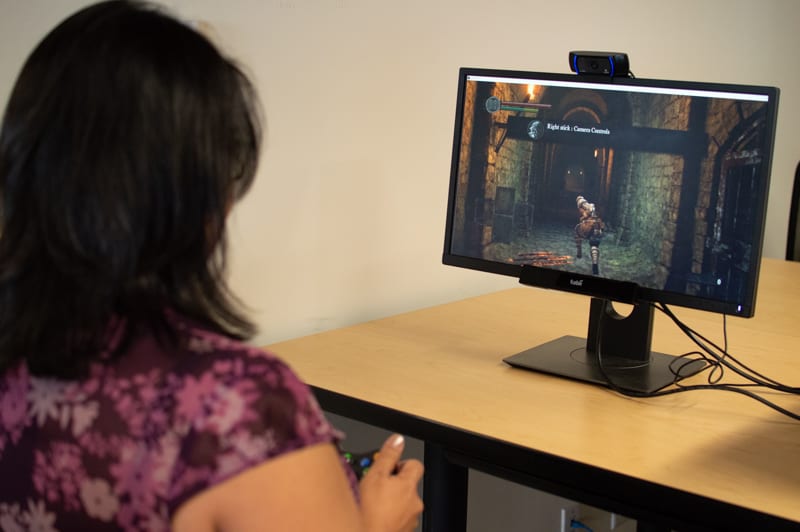
The High-Quality Standard of Webcam-Based Eye Tracking
While affordability and accessibility are key advantages, the technology’s impact hinges on its accuracy. iMotions’ WebET 3.0 algorithm delivers high performance, ensuring that users can rely on precise and actionable insights.
To validate the quality of WebET 3.0, iMotions conducted an in-house accuracy test, comparing it to one of the most accurate screen-based eye trackers on the market. The results demonstrated that webcam-based eye tracking can achieve levels of precision previously reserved for specialized systems.
Webcam-based Eye Tracking Accuracy in Practice
Study findings
As stated above, the study set out to measure the accuracy of, and subsequently the ideal conditions of use for, the webcam eye tracking algorithm. Below we go through the various conditions of the study setup, but if you want to study the findings of the study in detail you can download the white paper here.
Methodology
Stimuli were presented on a 22” computer screen in a dimly lit room. Respondents were sitting in front of a neutral grey wall and at a distance of 65 cm from the web camera, and a reading lamp illuminated the respondents’ faces from the front. Web camera data was collected with a Logitech Brio camera sampling at 30 Hz with a resolution of 1920×1080 px. Simultaneously, screen-based eye tracking data was collected with a top-of-the-line screen-based eye tracker without a chinrest. Respondents were instructed to sit perfectly still and not to talk.
Aside from the ideal conditions described above, four extra conditions were tested – participants wearing glasses, a low web camera resolution, suboptimal face illumination, or having the respondent move and talk.
Ideal Conditions
Under most ideal conditions, without any manipulations (n=10), the WebET had an average accuracy offset of 2.2 dva and 1% of trials were lost due to data dropout (for screen-based eye tracker data, average accuracy was 0.5 dva with no lost trials). In this condition, webET data from 12% of all trials had average offsets larger than 5 dva.
Note: “dva” is short for dynamic visual acuity, which is the ability to resolve fine spatial detail in dynamic objects during head fixation, or in static objects during head or body rotation.
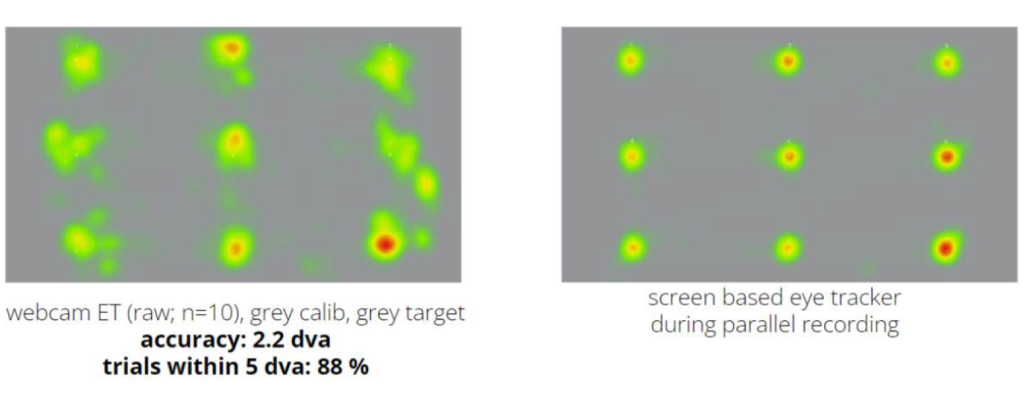
Movement
Data recorded from respondents who were talking and moving their heads (n=4) was worse than data recorded while the same respondents were sitting perfectly still. Data from the screen-based eye tracker confirmed that respondents correctly maintained their gaze on the targets (and average accuracy was 0.7 dva with 3% lost trials). The webET algorithm succeeded to calculate gaze data for 100% of the trials with moving respondents with an average offset of 5.0 dva. 38% of the trials had an offset larger than 5 dva.
A paired Wilcoxon signed-ranks test comparing webET data from trials with moving respondents (median 3.9 dva, Q1 2.2 dva, Q3 6.2 dva) to the equivalent blocks in which the same respondents sat still (median 1.8 dva, Q1 1.1 dva, Q3 3.1 dva) revealed highly significant differences (p<<0.01) between the two conditions.
Sidelight
Strong sidelight (n=4) (i.e light from a window, lamp, or sitting outside) also caused data offsets with an average accuracy of 4.9 dva for webET and 5% lost trials (for the screen-based eye tracker, average accuracy was 0.6 dva with 1% lost trials) and 4% of trials had average offsets of more than 5 dva for webET data. A paired Wilcoxon signed-ranks test comparing webET data from trials with bad face illumination (median 4.2 dva, Q1 2.5 dva, Q3 6.6 dva) to the equivalent blocks in which the same respondents were recorded under ideal conditions (median 1.8 dva, Q1 1.1 dva, Q3 3.1 dva) revealed highly significant differences (p<<0.01) between the two conditions.
Low resolution
Lower camera resolutions also caused some, but not as pronounced, increase in data offsets with an average accuracy of 5.0 dva (for the screen-based eye tracker, average accuracy was 0.6 dva) and a third of trials showing an average offset of webET data above 5 dva.
Glasses
For the 5 respondents who were re-recorded wearing glasses, the largest average offset of 3.6 dva was observed for webET (for the screen-based eye tracker, average accuracy was 0.9 dva) and 41% of the trials had an average offset of webET data higher than 5 dva.
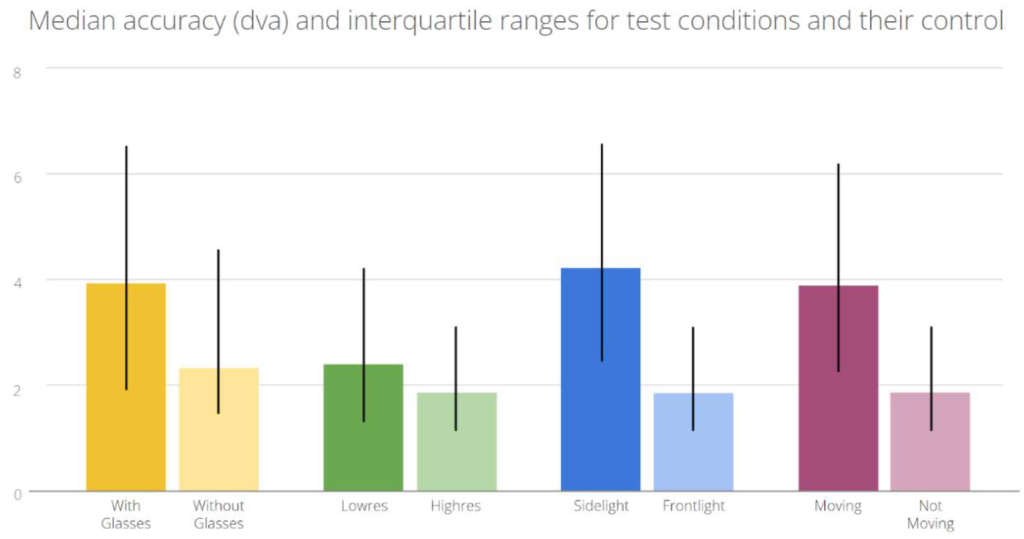
Conclusion
What this study shows is that the accuracy of webcam eye tracking is becoming less and less negatively affected by the aforementioned distorting factors (moving, talking, bad lighting, and wearing glasses) than was the case in the initial iterations of the algorithm.
However, while it is clear that the quality of eye tracking data gets better and better, it is also clear that, in order to optimally employ webcam eye tracking in research, respondents must still adhere to the instructions of best practices from the study organizer in order to ensure the best quality of data.
It is very encouraging to note that when ideal conditions are met, webcam eye tracking shows good data consistency and good data quality, making it a very viable data collection tool.
Webcam-based eye tracking is gaining momentum
Even though the data quality of webcam eye tracking is still not exactly comparable to dedicated eye tracking hardware, it is the perfect option for when you want to scale your research. We like to think of it as making our clients among the first to be able to conduct quantitative human behavior research.
If you plan on conducting continuous eye tracking research where both accuracy and precision are key, then investing in proper hardware is still well worth it. But if you are setting out to conduct large-scale UX testing, A/B testing, or image/video studies with eye tracking we are certain that webcam eye tracking will be a valuable tool for you – and it’s only getting better.
If you are interested to know more about how webcam eye tracking can help you scale your research and reach a global audience, please check out our Remote Data Collection page. If you are interested in a teaching tool that uses web camera based eye tracking, see our iMotions Online page.
Get a Demo
We’d love to learn more about you! Talk to a specialist about your research and business needs and get a live demo of the capabilities of the iMotions Research Platform.