Human communication is multimodal in nature; it is through multiple modalities, i.e., language, voice, and facial expressions, that opinions and emotions are expressed. Data in this domain exhibits complex multi-relational and temporal interactions. Learning from this data is a fundamentally challenging research problem. In this paper, we propose Multimodal Temporal Graph Attention Networks (MTGAT). MTGAT is an interpretable graph-based neural model that provides a suitable framework for analyzing this type of multimodal sequential data. We first introduce a procedure to convert unaligned multimodal sequence data into a graph with heterogeneous nodes and edges that captures the rich interactions between different modalities through time. Then, a novel graph operation, called Multimodal Temporal Graph Attention, along with a dynamic pruning and read-out technique is designed to efficiently process this multimodal temporal graph.
By learning to focus only on the important interactions within the graph, our MTGAT is able to achieve state-of-the-art performance on multimodal sentiment analysis and emotion recognition benchmarks including IEMOCAP and CMU-MOSI, while utilizing significantly fewer computations.
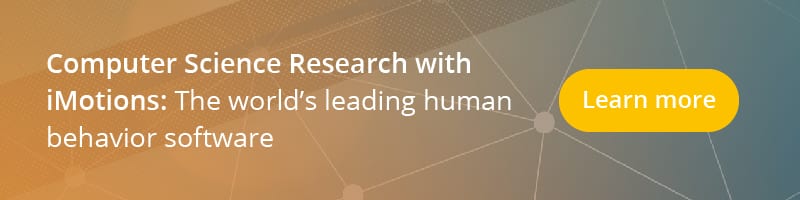
We provide more details regarding the word-aligned and unalignd data sequences. In the word-aligned version, video and audio features are average-pooled based on word boundaries (extracted using P2FA (Yuan and Liberman 2008)) resulting in an equal sequence lengths of 50 for all three modalities. In the unaligned version, original audio and video features are used, resulting in a variable sequence lengths. For both datasets, the multimodal features are extracted from the textual (GloVe word embedding (Pennington, Socher, and Manning 2014)), visual (Facet (iMotions 2017)), and acoustic (COVAREP (Degottex et al. 2014)) data modalities.
Have you done Research with iMotions?
We want to do more for researchers. Please contact us if you have done research using the iMotions Software Platform and would like to be featured here on our publications list and promoted to our community.